8 Things You Should Know About Large Language Models

In Brief
Large language models (LLMs) are used to explore the nuances of natural language, improve the ability of machines to comprehend and generate text, and automate tasks such as voice recognition and machine translation.
There is no easy solution to managing LLMs, but they are just as capable as humans.
With a surge in the development of natural language processing and its usage in business, there is a growing interest in large language models. These models are used to explore the nuances of natural language, improve the ability of machines to comprehend and generate text and automate tasks such as voice recognition and machine translation. Here are eight essential things that you should know about large language models (LLM).
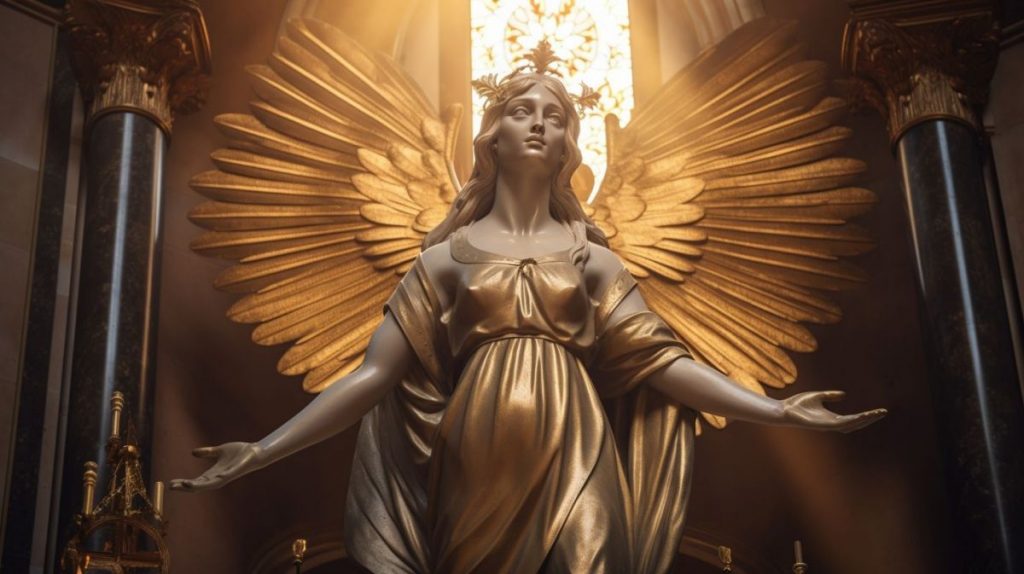
- LLMs are more “capable” as costs keep rising
- A quick look at how GPT models adapt as training costs rise
- LLMs learn to play board games by using representations of the outside world
- There is no easy solution to managing LLM
- Experts have trouble explaining how the LLM works
- LLMs are just as capable as humans
- LLMs must be more than just ”jack-of-all-trades”
- Models are ‘smarter’ than people think based on first impressions
LLMs are more “capable” as costs keep rising
LLMs predictably become more “capable” with increasing costs, even without cool innovations. The main thing here is predictability, which was shown in the article about GPT-4: five to seven small models were taught with a budget of 0.1% of the final one, and then a prediction was made for a huge model based on this. For a general assessment of perplexity and metrics on a subsample of one specific task, such a prediction was very accurate. This predictability is important for businesses and organizations that rely on LLMs for their operations, as they can budget accordingly and plan for future expenses. However, it’s important to note that while increasing costs may lead to improved capabilities, the rate of improvement may eventually plateau, making it necessary to invest in new innovations to continue advancing.
A quick look at how GPT models adapt as training costs rise
However, specific important skills tend to emerge unpredictably as a by-product of increasing training costs (longer training, more data, bigger model) — it’s almost impossible to predict when models will start to perform certain tasks. We explored the topic more in-depth in our article about the history of the development of GPT models. The picture shows the distribution of the increase in the quality of models across different tasks. It’s only the big models that can learn to do various tasks. This graph highlights the significant impact of scaling up the size of GPT models on their performance across various tasks. However, it is important to note that this comes at the cost of increased computational resources and environmental impact.
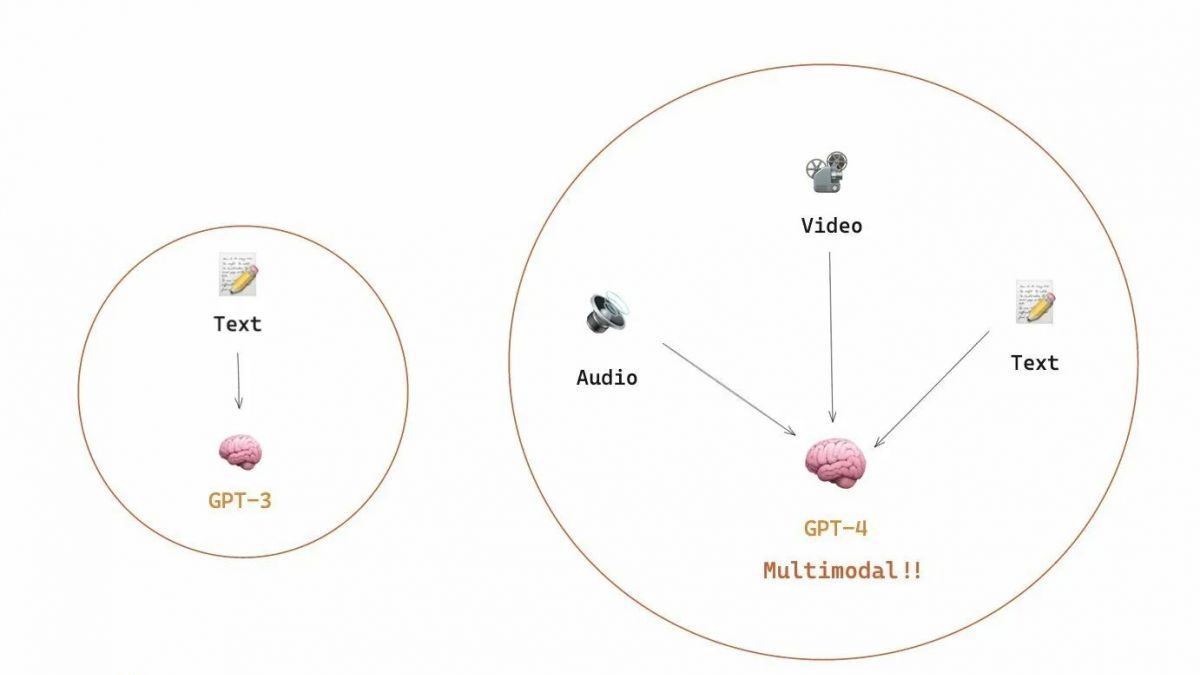
LLMs learn to play board games by using representations of the outside world
LLMs often learn and use representations of the outside world. There are many examples here, and here is one of them: Models trained to play board games based on descriptions of individual moves, without ever seeing a picture of the playing field, learn internal representations of the state of the board at each move. These internal representations can then be used to predict future moves and outcomes, allowing the model to play the game at a high level. This ability to learn and use representations is a key aspect of machine learning and artificial intelligence.
There is no easy solution to managing LLM
There are no reliable methods to control LLM behavior. Although there has been some progress in understanding and mitigating various problems (including ChatGPT and GPT-4 with the help of feedback), there is no consensus on whether we can solve them. There is growing concern this will become a huge, potentially catastrophic problem in the future when even larger systems are created. Therefore, researchers are exploring new methods to ensure that AI systems align with human values and goals, such as value alignment and reward engineering. However, it remains a challenging task to guarantee the safety and reliability of LLMs in complex real-world scenarios.
Experts have trouble explaining how the LLM works
Experts cannot yet interpret the inner workings of the LLM. No technique would allow us to state in any satisfactory way what kinds of knowledge, reasoning, or goals the model uses when it generates any result. This lack of interpretability raises concerns about the reliability and fairness of the LLM’s decisions, especially in high-stakes applications such as criminal justice or credit scoring. It also highlights the need for further research on developing more transparent and accountable AI models.
LLMs are just as capable as humans
Although LLMs are trained primarily to imitate human behavior when writing text, they have the potential to surpass us in many tasks. This can already be seen when playing chess or Go. This is due to their ability to analyze vast amounts of data and make decisions based on that analysis at a speed humans cannot match. However, LLMs still lack the creativity and intuition that humans possess, which makes them less suitable for many tasks.
LLMs must be more than just ”jack-of-all-trades”
LLMs must not express the values of their creators or the values encoded in a selection from the Internet. They should not repeat stereotypes or conspiracy theories or seek to offend anyone. Instead, LLMs should be designed to provide unbiased and factual information to their users while respecting cultural and societal differences. Additionally, they should undergo regular testing and monitoring to ensure they continue to meet these standards.
Models are ‘smarter’ than people think based on first impressions
Estimates of a model’s ability based on first impressions are often misleading. Very often, you need to come up with the right prompt, suggest a model, and maybe show examples, and it will start to cope much better. That is, it is “smarter” than it seems at first glance. Therefore, it is crucial to give the model a fair chance and provide it with the necessary resources to perform at its best. With the right approach, even seemingly inadequate models can surprise us with their capabilities.
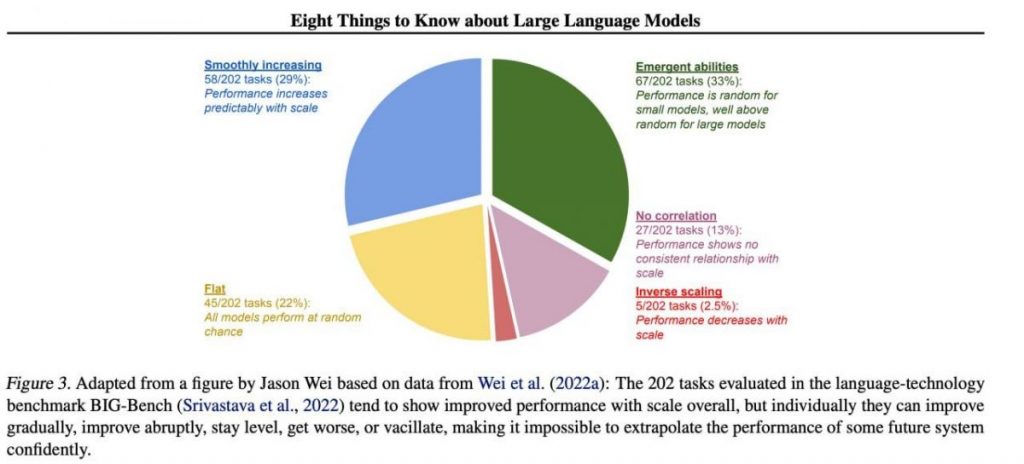
If we focus on a sample of 202 tasks from the BIG-Bench dataset (it was specially made difficult to test language models from and to), then as a rule (on average), the models show an increase in quality with increasing scale, but individually, the metrics in tasks can:
- improve gradually,
- improve drastically,
- remain unchanged,
- decrease,
- show no correlation.
All this leads to the impossibility of confidently extrapolating the performance of any future system. The green part is especially interesting — this is exactly where the quality indicators jump up sharply for no reason at all.
Read more about AI:
Disclaimer
In line with the Trust Project guidelines, please note that the information provided on this page is not intended to be and should not be interpreted as legal, tax, investment, financial, or any other form of advice. It is important to only invest what you can afford to lose and to seek independent financial advice if you have any doubts. For further information, we suggest referring to the terms and conditions as well as the help and support pages provided by the issuer or advertiser. MetaversePost is committed to accurate, unbiased reporting, but market conditions are subject to change without notice.
About The Author
Damir is the team leader, product manager, and editor at Metaverse Post, covering topics such as AI/ML, AGI, LLMs, Metaverse, and Web3-related fields. His articles attract a massive audience of over a million users every month. He appears to be an expert with 10 years of experience in SEO and digital marketing. Damir has been mentioned in Mashable, Wired, Cointelegraph, The New Yorker, Inside.com, Entrepreneur, BeInCrypto, and other publications. He travels between the UAE, Turkey, Russia, and the CIS as a digital nomad. Damir earned a bachelor's degree in physics, which he believes has given him the critical thinking skills needed to be successful in the ever-changing landscape of the internet.
More articles

Damir is the team leader, product manager, and editor at Metaverse Post, covering topics such as AI/ML, AGI, LLMs, Metaverse, and Web3-related fields. His articles attract a massive audience of over a million users every month. He appears to be an expert with 10 years of experience in SEO and digital marketing. Damir has been mentioned in Mashable, Wired, Cointelegraph, The New Yorker, Inside.com, Entrepreneur, BeInCrypto, and other publications. He travels between the UAE, Turkey, Russia, and the CIS as a digital nomad. Damir earned a bachelor's degree in physics, which he believes has given him the critical thinking skills needed to be successful in the ever-changing landscape of the internet.