Text-to-3D: Google has developed a neural network that generates 3D models from text descriptions
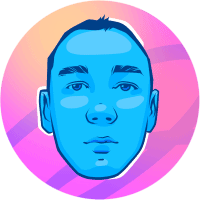
In Brief
Text-to-3D neural network can generate 3D models from text
DreamFusion optimizes 3D scenes based on Imagen text-to-image
2D diffusion model can be used for text-to-image synthesis
Google created a neural network capable of creating 3D models from text descriptions. The best part is that the most difficult aspect didn’t even need to be taught. Imagen was used as the foundation for Text-to-3D.
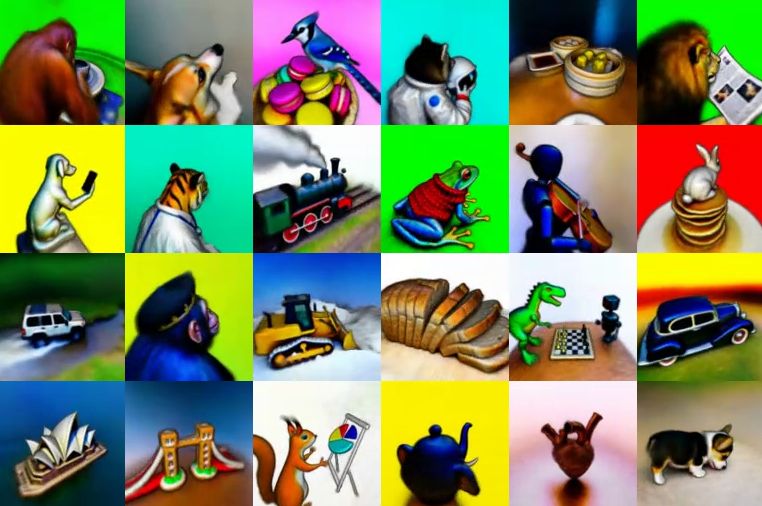
What should you know about DreamFusion?
Diffusion models trained on billions of image-text pairs have led to recent advances in text-to-image synthesis. Adapting this approach to 3D synthesis will necessitate large-scale datasets of labeled 3D assets as well as efficient denoising 3D data architectures, neither of which are currently available. In this paper, we overcome these restrictions by performing text-to-3D synthesis with a pretrained 2D text-to-image diffusion model. We present a loss based on probability density distillation that allows a 2D diffusion model to be used as a prior for optimizing a parametric picture generator. Using this loss, we use gradient descent to optimize a randomly initialized 3D model (a Neural Radiance Field or NeRF) so that its 2D renderings from random angles have a minimal loss.
The generated 3D model of the specified text can be viewed from any angle, illuminated with variable lighting, and composited into any 3D environment. Its method requires no 3D training data and no changes to the image diffusion model, illustrating the efficacy of using pretrained image diffusion models as prior.
Examples of Generated 3D From Text
Putting objects together to make a scene
How does it work?
DreamFusion optimizes a 3D scene based on a caption using the Imagen text-to-image generative model. It suggests Score Distillation Sampling (SDS), which involves optimizing a loss function to produce samples from a diffusion model. As long as we can map back to images differently, SDS enables us to optimize samples in any parameter space, such as a 3D space. To define this differentiable mapping, it employs a 3D scene parameterization that is akin to Neural Radiance Fields or NeRFs. SDS alone creates a passable scene appearance, but DreamFusion enhances geometry with extra regularizers and optimization techniques. The trained NeRFs that are produced are coherent, have excellent normals, surface geometry, and depth, and can be relit using a Lambertian shading model.
Read related articles:
Disclaimer
In line with the Trust Project guidelines, please note that the information provided on this page is not intended to be and should not be interpreted as legal, tax, investment, financial, or any other form of advice. It is important to only invest what you can afford to lose and to seek independent financial advice if you have any doubts. For further information, we suggest referring to the terms and conditions as well as the help and support pages provided by the issuer or advertiser. MetaversePost is committed to accurate, unbiased reporting, but market conditions are subject to change without notice.
About The Author
Damir is the team leader, product manager, and editor at Metaverse Post, covering topics such as AI/ML, AGI, LLMs, Metaverse, and Web3-related fields. His articles attract a massive audience of over a million users every month. He appears to be an expert with 10 years of experience in SEO and digital marketing. Damir has been mentioned in Mashable, Wired, Cointelegraph, The New Yorker, Inside.com, Entrepreneur, BeInCrypto, and other publications. He travels between the UAE, Turkey, Russia, and the CIS as a digital nomad. Damir earned a bachelor's degree in physics, which he believes has given him the critical thinking skills needed to be successful in the ever-changing landscape of the internet.
More articles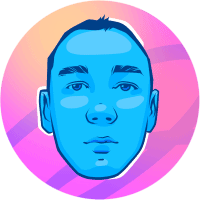
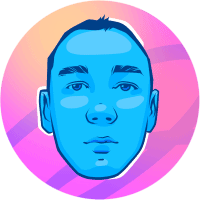
Damir is the team leader, product manager, and editor at Metaverse Post, covering topics such as AI/ML, AGI, LLMs, Metaverse, and Web3-related fields. His articles attract a massive audience of over a million users every month. He appears to be an expert with 10 years of experience in SEO and digital marketing. Damir has been mentioned in Mashable, Wired, Cointelegraph, The New Yorker, Inside.com, Entrepreneur, BeInCrypto, and other publications. He travels between the UAE, Turkey, Russia, and the CIS as a digital nomad. Damir earned a bachelor's degree in physics, which he believes has given him the critical thinking skills needed to be successful in the ever-changing landscape of the internet.