10 Most Misunderstood Questions about AI and Neural Networks in 2023
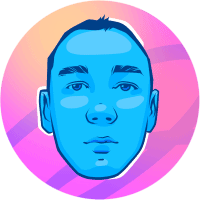
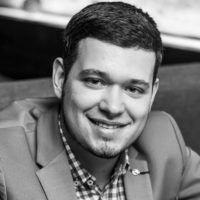
Because the field of AI and neural networks is constantly evolving and becoming more complex, there are a lot of misunderstandings and questions that people may be reluctant to ask. We sat down with well-known AI experts to discuss ten frequently misunderstanding questions about neural networks in an effort to clarify these issues. What they said was as follows:
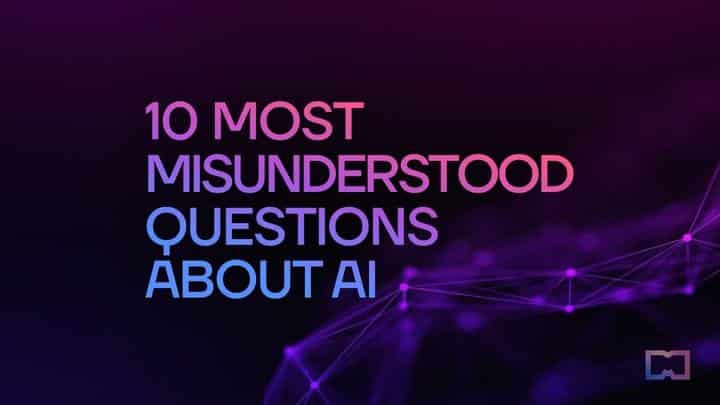
- 1. Is it possible for AI to fall in love?
- 2. Can AI start to cause harm and eventually rule the world?
- 3. Is it risky to upload your voice, appearance, and text-to-speech style into AI?
- 4. Uploading consciousness to computers: reality or science fiction?
- 5. Is it true that AI will take away work from people?
- 6. AI and artistic images: reproduction or theft?
- 7. Can I use GPT-4 instead of Google Searches?
- 8. Can AI be creative?
- 9. Can AI truly think?
- 10. How could ChatGPT be made at all? And Midjourney or DALL-E?
1. Is it possible for AI to fall in love?
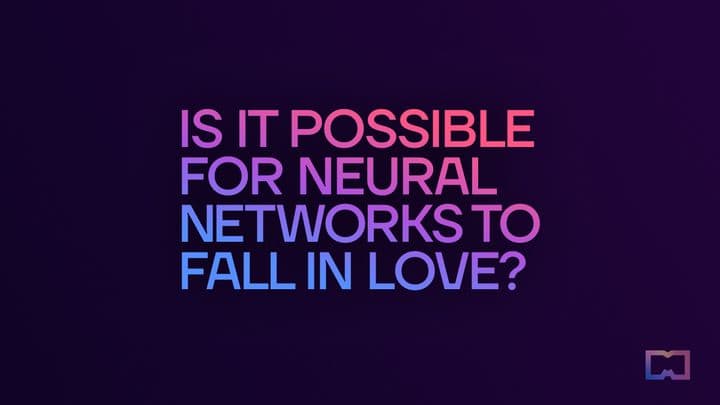
Neural networks are mathematical models inspired by the human brain’s structure. They consist of interconnected nodes or “neurons” that process information. By learning from data, they can perform specific tasks such as text generation, image recognition, or even simulating human-like writing styles.
Can AI “Love”?
The concept of love is intrinsically tied to consciousness, self-awareness, empathy, and a range of other complex emotional and cognitive processes. Neural networks, however, do not possess these attributes.
For example, a neural network can be trained to generate text that resembles a love letter if given the appropriate context and instructions. If provided with the first chapter of a love story and asked to continue in a similar vein, the model will comply. But it does so based on patterns and statistical likelihood, not because of any emotional connection or feelings of affection.
Another critical aspect to consider is memory. In their basic form, neural networks lack the ability to retain information between different launches. They operate without continuity or awareness of past interactions, essentially reverting to their “factory settings” after each use.
Memory and Neural Networks
While memory can be artificially added to a neural network, allowing it to reference past “memories” or data, this does not imbue the model with consciousness or emotion. Even with a memory component, the neural network’s response is dictated by mathematical algorithms and statistical probabilities, not personal experience or sentiment.
The notion of a neural network falling in love is a captivating but fictional idea. Current AI models, regardless of their complexity and capabilities, do not have the capacity to experience emotions such as love.
The text generation and responses observed in sophisticated models are the result of mathematical computations and pattern recognition, not genuine affection or emotional intelligence.
2. Can AI start to cause harm and eventually rule the world?
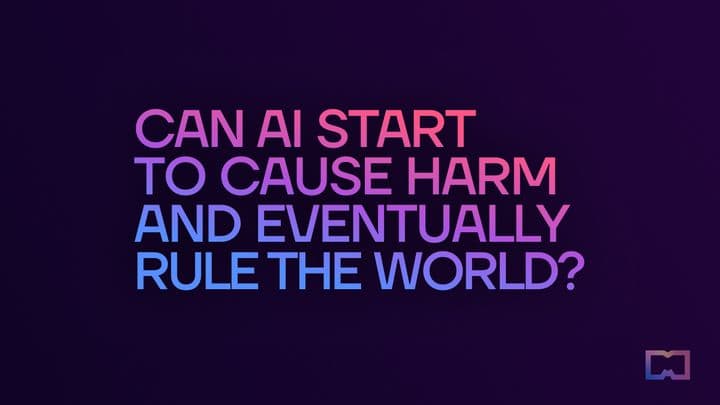
Today’s neural networks operate without full-proof methods to ensure that they abide by specific rules. For instance, preventing a model from using offensive language is a surprisingly challenging task. Despite efforts to set such restrictions, there are always ways that the model might find to circumvent them.
The Future of Neural Networks
As we move towards more advanced neural networks, such as hypothetical GPT-10 models with human-like abilities, the challenge of control becomes even more pressing. If these systems were given free rein without specific tasks or constraints, their actions could become unpredictable.
The debate on the likelihood of a negative scenario resulting from these developments varies widely, with estimates ranging from 0.01% to 10%. While these probabilities may seem low, the potential consequences could be catastrophic, including the possibility of human extinction.
Efforts in Alignment and Control
Products like ChatGPT and GPT-4 are examples of ongoing efforts to align the intentions of neural networks with human goals. These models are designed to follow instructions, maintain polite interaction, and ask clarifying questions. However, these controls are far from perfect, and the problem of managing these networks is not even halfway solved.
The challenge of creating foolproof control mechanisms for neural networks is one of the most vital research areas in the field of artificial intelligence today. The uncertainty about whether this problem can be solved and the methods required to do so only adds to the urgency of the issue.
3. Is it risky to upload your voice, appearance, and text-to-speech style into AI?
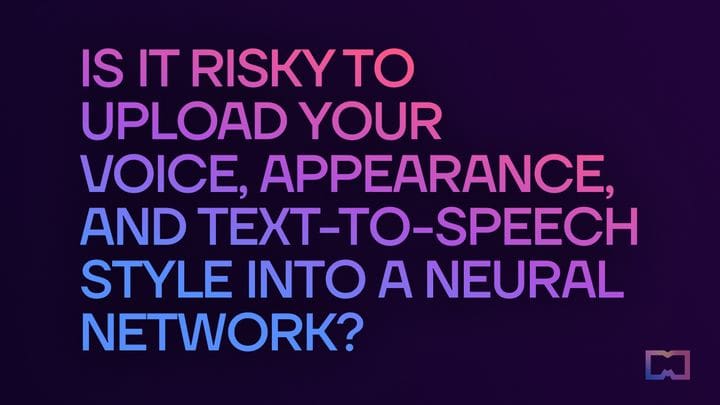
In an age where digital technologies are rapidly advancing, concerns about the safety of personal information such as voice, appearance, and text style are growing. While the threat of digital identity theft is real, it is essential to understand the context and the measures being taken to address this challenge.
Digital Identity and Neural Networks
In neural networks, it’s not a matter of uploading personal attributes but rather training or re-training models to mimic one’s appearance, voice, or text. These trained models can indeed be stolen by copying the script and parameters, allowing them to run on another computer.
The potential misuse of this technology is significant, as it has reached a level where deepfake videos and voice cloning algorithms can convincingly replicate an individual. The creation of such deceptive content can be costly and time-consuming, requiring thousands of dollars and numerous hours of recording. However, the risk is tangible and emphasizes the need for reliable identification and confirmation methods.
Efforts to Ensure Identity Security
Various initiatives are underway to tackle the problem of digital identity theft. Startups like WorldCoin, in which OpenAI’s head Sam Altman has invested, are exploring innovative solutions. WorldCoin’s concept involves assigning a unique key to each piece of information about a person, allowing for subsequent identification. This method could also be applied to mass media to verify the authenticity of news.
Despite these promising developments, the implementation of such systems across all industries is a complex and large-scale endeavor. Currently, these solutions remain at the prototype stage, and their widespread adoption may not be feasible within the next decade.
4. Uploading consciousness to computers: reality or science fiction?
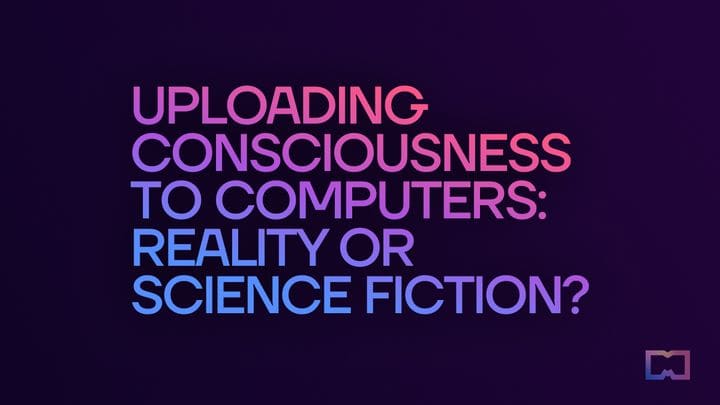
The idea of transferring human consciousness into a computer has been a fascinating subject for science fiction enthusiasts. But is it something that current technology or even future advancements could achieve? The notion of living forever through a digital twin certainly captures the imagination, but the reality is far more complex.
Imitation but Not Duplication
With existing technologies, such as those found in models like GPT-4, it is possible to teach a neural network to imitate one’s communication style, learn personal jokes, and even invent new ones in a unique style and manner of presentation. This, however, is not synonymous with transferring one’s consciousness.
The complexity of consciousness goes far beyond communication style and personal quirks. Humanity still lacks a concrete understanding of what consciousness is, where it is stored, how it differentiates individuals, and what exactly makes a person uniquely themselves.
Potential Future Possibilities
The hypothetical scenario of transferring consciousness would require defining consciousness as a combination of memories, experiences, and individual characteristics of perception. If such a definition were to be accepted, there might be a theoretical pathway to simulating further life through the transfer of this knowledge into a neural network.
However, this theory is merely speculative and not grounded in current scientific understanding or technological capabilities. The question of consciousness is one of the most profound and elusive subjects in philosophy, neuroscience, and cognitive science. Its complexity extends far beyond the capacity of current artificial intelligence and neural network technology.
5. Is it true that AI will take away work from people?
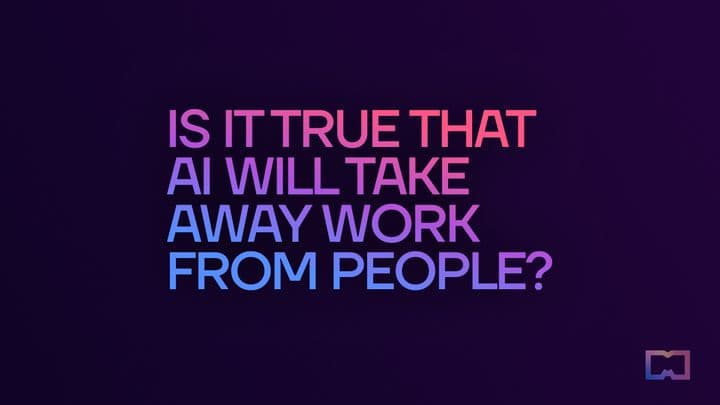
Automation through AI will likely affect professions where work involves routine execution of instructions. Examples include tax assistants-consultants who help with declarations and clinical trial data managers whose work revolves around filling out reports and reconciling them with standards. The potential for automation in these roles is clear, given that the necessary information is readily available and the cost of labor is above average.
On the other hand, professions like cooking or bus driving remain secure for the foreseeable future. The challenge of connecting neural networks to the real physical world, combined with existing legislation and regulations, makes automation in these fields a more complex endeavor.
Changes and Opportunities
Automation doesn’t necessarily imply a total replacement of human workers. It often leads to the optimization of routine tasks, allowing people to focus on more creative and engaging responsibilities.
1. Journalism: In industries like journalism, neural networks may soon assist in drafting articles with a set of theses, leaving human writers to make precise adjustments.
2. Education: Perhaps the most exciting transformation lies in education. Research indicates that personalized approaches improve educational outcomes. With AI, we can envision personalized assistants for each student, dramatically enhancing the quality of education. Teachers’ roles will evolve towards strategic planning and control, focusing on determining programs of study, testing knowledge, and guiding overall learning.
6. AI and artistic images: reproduction or theft?
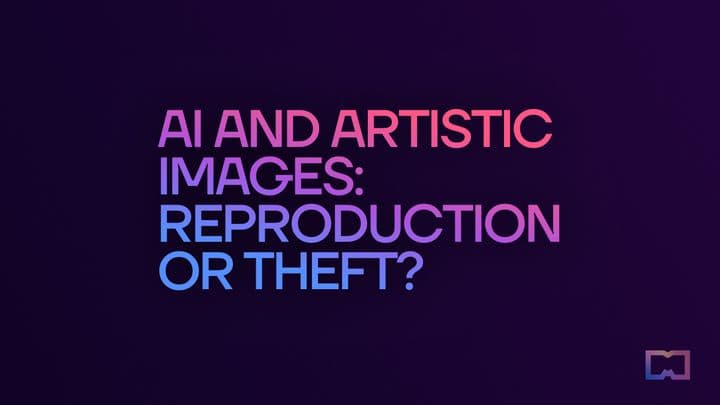
AI learns by studying various forms of art, recognizing different styles, and attempting to imitate them. The process is akin to human learning, where students of art observe, analyse, and emulate the works of different artists.
AI operates on the principle of error minimization. If a model encounters a similar image hundreds of times during its training, it may memorize that image as part of its learning strategy. This does not mean the network is storing the image, but rather recognizing it in a way similar to human memory.
A Practical Example
Consider an art student who draws two pictures every day: one unique and the other a reproduction of the Mona Lisa. After repeatedly drawing the Mona Lisa, the student will be able to reproduce it with considerable accuracy, but not exactly. This learned ability to recreate does not equate to theft of the original work.
Neural networks function in a comparable manner. They learn from all images they encounter during training, with some images being more common and thus more accurately reproduced. This includes not only famous paintings but any image in the training sample. Even though there are methods to eliminate duplicates, they are not flawless, and research has shown that certain images may appear hundreds of times during training.
7. Can I use GPT-4 instead of Google Searches?
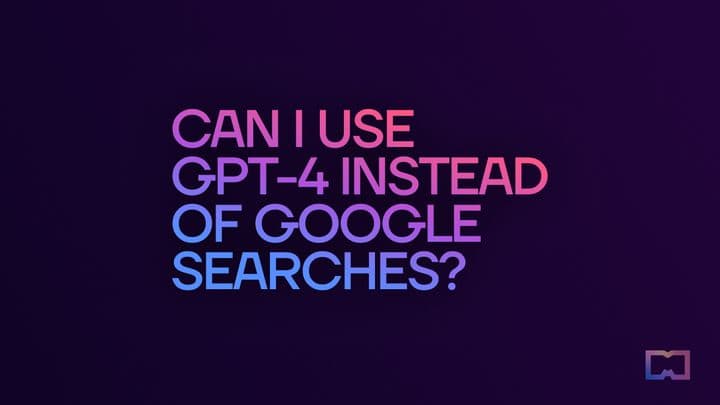
According to internal estimates by OpenAI, the current leading model, GPT-4, answers correctly about 70-80% of the time, depending on the topic. While this may seem short of the ideal 100% accuracy, it marks a significant improvement over the previous generation of models based on the GPT-3.5 architecture, which had an accuracy rate of 40-50%. This considerable increase in performance was achieved within 6-8 months of research.
Context Matters
The figures mentioned above relate to questions asked without specific context or accompanying information. When context is provided, such as a Wikipedia page, the model’s accuracy approaches 100%, adjusted for the source’s correctness.
The distinction between context-free and context-rich questions is crucial. For example, a question about Einstein’s birth date without any accompanying information relies solely on the model’s internal knowledge. But with a specific source or context, the model can provide a more accurate response.
Google Searches Within GPT-4
An interesting development in this field is the integration of internet searches within GPT-4 itself. This allows users to delegate part of the internet search to GPT-4, potentially reducing the need to manually Google information. This feature, however, requires a paid subscription.
Looking Ahead
OpenAI CEO Sam Altman anticipates that the reliability of factual information within the model will continue to improve, with a projected timeline of 1.5-2 years to further refine this aspect.
8. Can AI be creative?
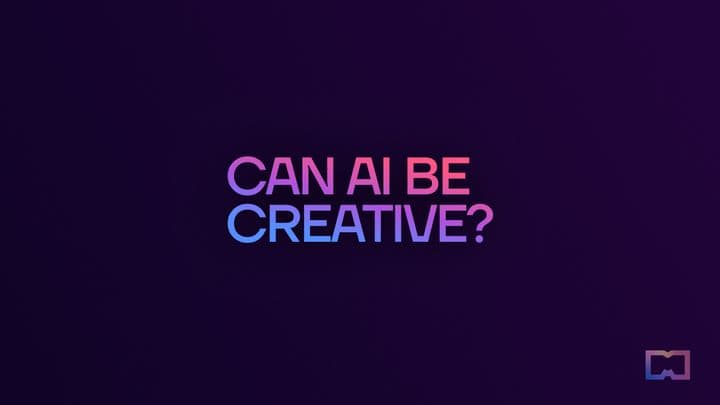
For some, creativity is an inherent ability, something that all humans possess to varying degrees. Others might argue that creativity is a learned skill or that it is confined to specific professions or activities. Even among humans, there are disparities in creative ability. Therefore, comparing human creativity to that of a neural network requires careful consideration of what creativity truly entails.
Neural Networks and Artistry
Recent developments have enabled neural networks to create art and poetry. Some models have produced works that could reach the finals of amateur competitions. However, this doesn’t occur consistently; success may be sporadic, perhaps one out of a hundred attempts.
The Debate
The above information has spurred intense debates. Opinions on whether neural networks can be considered creative vary widely. Some argue that the ability to create a poem or painting, even if only occasionally successful, constitutes a form of creativity. Others firmly believe that creativity is exclusively a human characteristic, bound by emotion, intention, and consciousness.
The subjective nature of creativity adds further complexity to the discussion. Even among people, the understanding and appreciation of creativity can differ vastly.
The Practical Implications
Beyond the philosophical debate, there are practical implications to consider. If neural networks can indeed be creative, what does that mean for industries reliant on creative output? Could machines augment or even replace human creativity in certain fields? These questions are not merely theoretical but have real-world significance.
9. Can AI truly think?
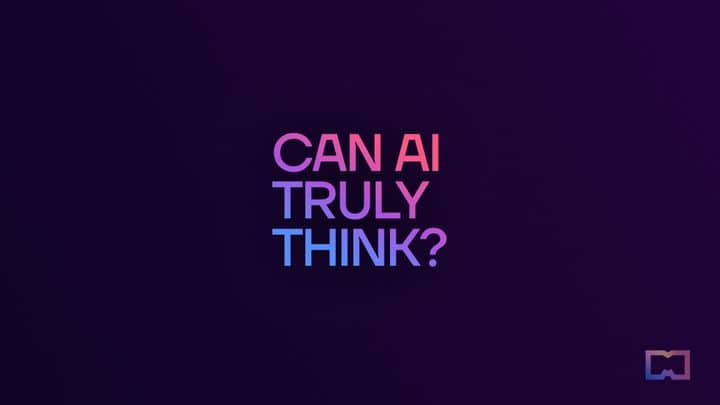
To explore whether neural networks can think, we first need to understand what constitutes a thought. For example, if we consider the process of understanding how to use a key to open a door as a thought process, then some might argue that neural networks are capable of similar reasoning. They can correlate states and desired outcomes. Others might challenge this, noting that neural networks rely on repeated exposure to data, much like humans learning through repeated observation.
Innovation and Common Thoughts
The debate becomes more intricate when considering innovative thoughts or ideas not commonly expressed. A neural network might generate a novel idea once in a million attempts, but does this qualify as thought? How does this differ from random generation? If humans also occasionally produce erroneous or ineffective thoughts, where is the line drawn between human and machine thinking?
Probability and Idea Generation
The concept of probability adds another layer of complexity. A neural network can produce millions of different responses, and among them, there might be a few innovative or meaningful ones. Does a certain ratio of meaningful to meaningless thoughts validate the capacity for thinking?
The Evolving Understanding of AI
Historically, as machines have been developed to solve complex problems, such as passing the Turing test, the goalposts for defining intelligence have shifted. What was once considered miraculous 80 years ago is now common technology, and the definition of what constitutes AI continually evolves.
10. How could ChatGPT be made at all? And Midjourney or DALL-E?
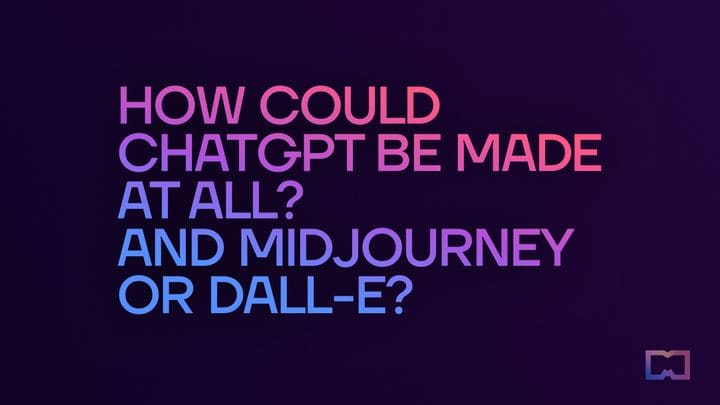
Neural networks, an idea that originated in the mid-20th century, have become central to the functioning of models such as ChatGPT and DALL-E. Although the early ideas may seem simplified by today’s standards, they laid the foundation for understanding how to replicate the workings of a biological brain through mathematical models. Here’s an exploration of the principles that make these neural networks possible.
1. Inspiration from Nature:
The term “neural network” itself draws inspiration from biological neurons, the core functional units of the brain. These artificial constructs comprise nodes, or artificial neurons, mimicking many aspects of natural brain function. This connection to biology has provided valuable insights into the creation of modern architectures.
2. Mathematics as a Tool:
Neural networks are mathematical models, allowing us to leverage the rich resources of mathematical techniques to analyze and evaluate these models. A simple example is a function that takes a number as input and adds two to it, like f(4) = 6. While this is a basic function, neural networks can represent far more complex relationships.
3. Handling Ambiguous Tasks:
Traditional programming falls short when dealing with tasks where the relationship between inputs and outputs is not easily describable. Take the example of categorizing pictures of cats and dogs. Despite their similarities, humans can easily distinguish between them, but expressing this distinction algorithmically is complex.
4. Training and Learning from Data:
Neural networks’ strength lies in their ability to learn from data. Given two sets of images (e.g., cats and dogs), the model learns to differentiate them by training itself to find connections. Through trial and error, and adjustment of its artificial neurons, it refines its ability to classify them correctly.
5. The Power of Large Models:
Theoretically, a large enough neural network with sufficient labeled data can learn any complex function. However, the challenges are in the required computing power and availability of correctly classified data. This complexity renders large models like ChatGPT nearly impossible to fully analyze.
6. Specialized Training:
ChatGPT, for example, was trained for two specific tasks: predicting the next word in a context and ensuring non-offensive yet useful and understandable answers. These precise training objectives have contributed to its popularity and widespread use.
7. The Ongoing Challenge of Understanding:
Despite these advances, fully understanding the inner workings of large, complex models remains an area of active research. The quest to demystify their intricate processes continues to occupy some of the best researchers in the field.
Wrap It Up
There are many complex details in the vast field of neural networks that could cause misunderstandings or misperceptions. We hope to dispel myths and give our readers accurate information by openly discussing these issues with subject-matter specialists. A key component of contemporary AI technology, neural networks continue to advance, and along with them, our understanding. In order to navigate the future of this fascinating field, open communication, ongoing learning, and responsible implementation will be essential.
Read more:
Disclaimer
In line with the Trust Project guidelines, please note that the information provided on this page is not intended to be and should not be interpreted as legal, tax, investment, financial, or any other form of advice. It is important to only invest what you can afford to lose and to seek independent financial advice if you have any doubts. For further information, we suggest referring to the terms and conditions as well as the help and support pages provided by the issuer or advertiser. MetaversePost is committed to accurate, unbiased reporting, but market conditions are subject to change without notice.
About The Author
Damir is the team leader, product manager, and editor at Metaverse Post, covering topics such as AI/ML, AGI, LLMs, Metaverse, and Web3-related fields. His articles attract a massive audience of over a million users every month. He appears to be an expert with 10 years of experience in SEO and digital marketing. Damir has been mentioned in Mashable, Wired, Cointelegraph, The New Yorker, Inside.com, Entrepreneur, BeInCrypto, and other publications. He travels between the UAE, Turkey, Russia, and the CIS as a digital nomad. Damir earned a bachelor's degree in physics, which he believes has given him the critical thinking skills needed to be successful in the ever-changing landscape of the internet.
More articles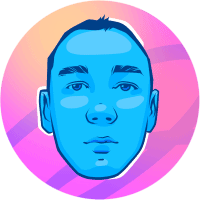
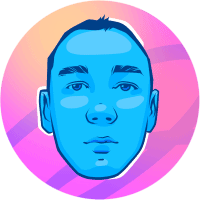
Damir is the team leader, product manager, and editor at Metaverse Post, covering topics such as AI/ML, AGI, LLMs, Metaverse, and Web3-related fields. His articles attract a massive audience of over a million users every month. He appears to be an expert with 10 years of experience in SEO and digital marketing. Damir has been mentioned in Mashable, Wired, Cointelegraph, The New Yorker, Inside.com, Entrepreneur, BeInCrypto, and other publications. He travels between the UAE, Turkey, Russia, and the CIS as a digital nomad. Damir earned a bachelor's degree in physics, which he believes has given him the critical thinking skills needed to be successful in the ever-changing landscape of the internet.