Top 10 AI Trading Strategies and Algorithms for 2023
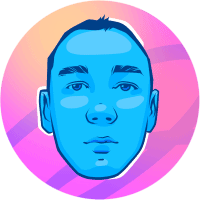
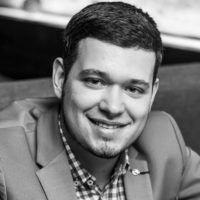
In Brief
The world of finance is undergoing a revolution driven by artificial intelligence. Advanced algorithms, which can process extensive datasets, uncover intricate non-linear connections, and make instantaneous decisions, are at the forefront of this transformation.
This guide delves into the ten foremost AI trading strategies poised to dominate in 2023. We provide insights into how each approach operates, its key advantages and limitations, and recommendations for successful implementation.
AI-powered trading systems have the unmatched ability to carefully examine enormous datasets, identify complex patterns, and carry out trades at rates that are faster than those of human traders. AI traders have a clear advantage in predicting price changes and making money.
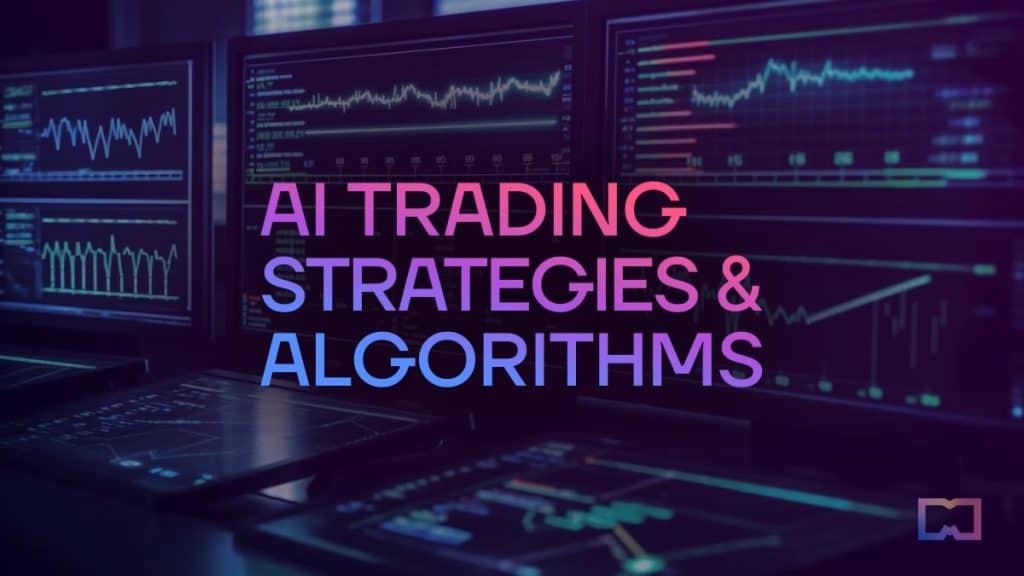
In this talk, we’ll explore the top ten AI trading strategies that are becoming increasingly popular among hedge funds, proprietary trading companies, and individual traders. We’ll explain how these strategies work, go over their advantages and disadvantages, and discuss how traders use them to make money.
Pro Tips |
---|
1. These advanced 10+ best AI crypto trading bots leverage AI to analyze market trends, execute trades, and maximize profits. |
2. Discover the top 5 AI stocks preferred by the financial elite. |
3. Stay ahead of the investment game and explore our curated list of the top 10 AI company stocks by annual return in 2023. |
- 1. AI Quantitative Momentum Trading
- 2. AI Mean Reversion Trading
- 3. AI Pattern Recognition Trading
- 4. AI Sentiment Analysis Trading
- 5. AI Algorithmic Hedging
- 6. AI Statistical Arbitrage Trading
- 7. AI Algorithmic Execution Trading
- 8. AI Smart Order Routing
- 9. AI Event-Driven Trading
- 10. AI/Human Collaborative Trading
The 10 AI Trading Strategies Market Share by Popularity
# | AI Trading Algorithm | Popularity |
---|---|---|
1 | AI Mean Reversion Trading | 62.34% |
2 | AI Smart Order Routing | 18.18% |
3 | AI Sentiment Analysis Trading | 3.90% |
4 | AI Statistical Arbitrage Trading | 3.90% |
5 | AI Quantitative Momentum Trading | 2.60% |
6 | AI Pattern Recognition Trading | 2.60% |
7 | AI Event-Driven Trading | 2.60% |
8 | AI Algorithmic Execution Trading | 1.30% |
9 | AI Algorithmic Hedging | 1.30% |
10 | AI/Human Collaborative Trading | 1.30% |
The 10 AI Trading Strategies Comparison Sheet
# | Strategy | Speed | Data usage | Frequency | Hold time | Risk level |
---|---|---|---|---|---|---|
1. | AI Momentum Trading | High | Moderate | High | Short term | moderate |
2. | AI Mean Reversion Trading | Low | Low | moderate | Short to medium-term | low |
3. | AI Pattern Recognition Trading | Moderate | High | Moderate | Short to medium-term | Moderate |
4. | AI Sentiment Analysis Trading | High | High | High | Intraday to short-term | High |
5. | AI Algorithmic Hedging | High | High | High | Medium to long-term | Low |
6. | AI Statistical Arbitrage Trading | Ultra High | High | Ultra High | Intraday | Low |
7. | AI Algorithmic Execution Trading | High | High | High | Short-term | Low |
8. | AI Smart Order Routing | Ultra High | High | Ultra High | Intraday | Low |
9. | AI Event-Driven Trading | High | High | Moderate | Short to medium-term | High |
10. | AI/Human Collaborative Trading | Moderate | Moderate | Moderate | Medium-term | Moderate |
1. AI Quantitative Momentum Trading
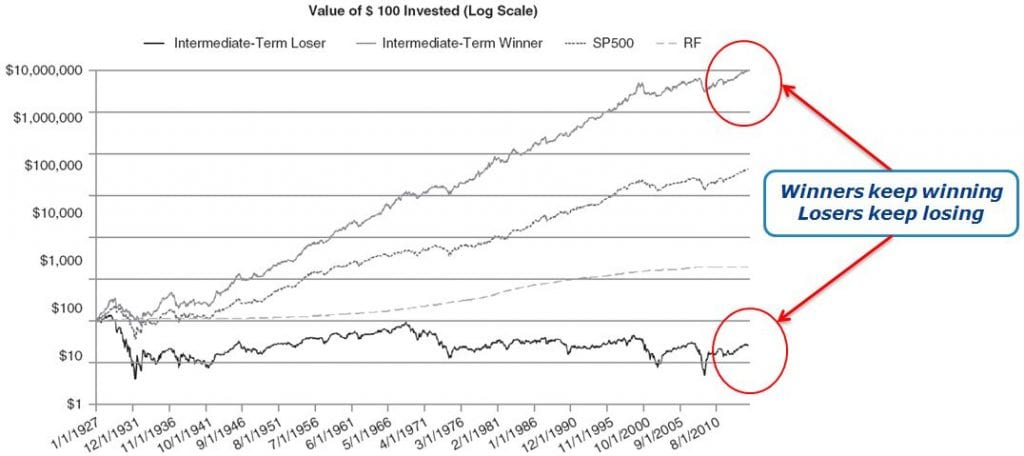
Operational Mechanism:
AI algorithms underpin this strategy by meticulously monitoring price trends across diverse securities such as stocks, futures, and currencies. It meticulously discerns securities exhibiting upward price momentum.
Pros:
- Capitalizes on prevailing trends and momentum for high-probability trades.
- Profits from both ascending and descending momentum.
- Precision is enhanced through quantitative-guided entries and exits.
Cons:
- Vulnerable to abrupt trend reversals and market volatility.
- Risk of excessive trading in the absence of stringent quantitative rules.
- Mandates constant surveillance and portfolio adjustments.
Implementation Recommendations:
- Employ an AI system incorporating deep learning algorithms for accurate momentum shift identification.
- Blend momentum signals with risk management strategies encompassing position sizing and stop-loss mechanisms.
- Exhibit a predilection for securities boasting robust price uptrends and substantial trading volumes.
- Safeguard against concentration risk through broad diversification across uncorrelated securities.
2. AI Mean Reversion Trading
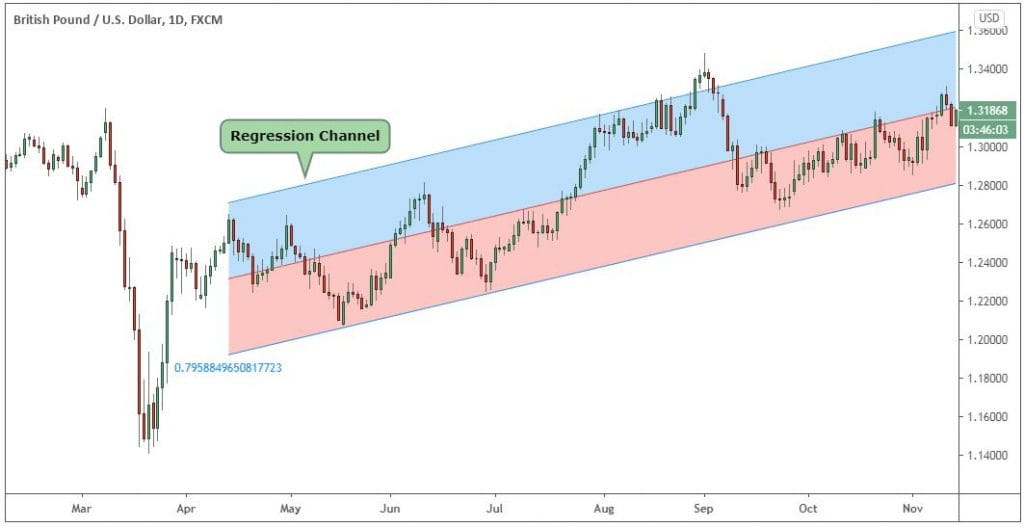
Operational Mechanism:
This strategy thrives on the propensity of markets to revert to their mean or average. AI algorithms undertake long positions in securities trading below the mean price and short positions in those trading above it, foreseeing an eventual reversion.
Pros:
- Thrives in range-bound markets devoid of defined trends.
- Harmonizes well with asset classes oscillating around a mean.
- Mean reversion boundaries confine risk.
Cons:
- Susceptible to entrapment in prolonged trends.
- Reversion might materialize after protracted intervals.
- Complex to execute accurately in the absence of quantitative capabilities.
Implementation Recommendations:
- Harness machine learning models, such as Artificial Neural Networks (ANNs), to refine mean reversion level estimates.
- Augment precision by incorporating sentiment analysis for trade entry enhancement.
- Define clear reversion price targets and enforce stop-loss mechanisms on both ends.
- Maintain prudently sized positions that are well-diversified.
3. AI Pattern Recognition Trading
Operational Mechanism:
AI algorithms are trained to discern historical price patterns that herald high-probability trading opportunities. Upon identifying these patterns, AI automatically initiates lucrative trades.
Pros:
- This timeless strategy exploits enduring market patterns.
- The synergy between AI and statistical backtesting engenders robust signals.
- Emotional biases are eliminated in the realm of pattern-based trading.
Cons:
- Substantial data prerequisites for the initial training phase.
- Patterns may fail or produce erroneous signals.
- Over-optimization may lead to better-fitted models.
Implementation Recommendations:
- Train the system over protracted time spans and under varied market conditions.
- Leverage an array of technical indicators to corroborate pattern fulfillment.
- Instill prudent money management and risk-control mechanisms.
- Tailor the system’s selectivity by targeting specific instruments.
4. AI Sentiment Analysis Trading
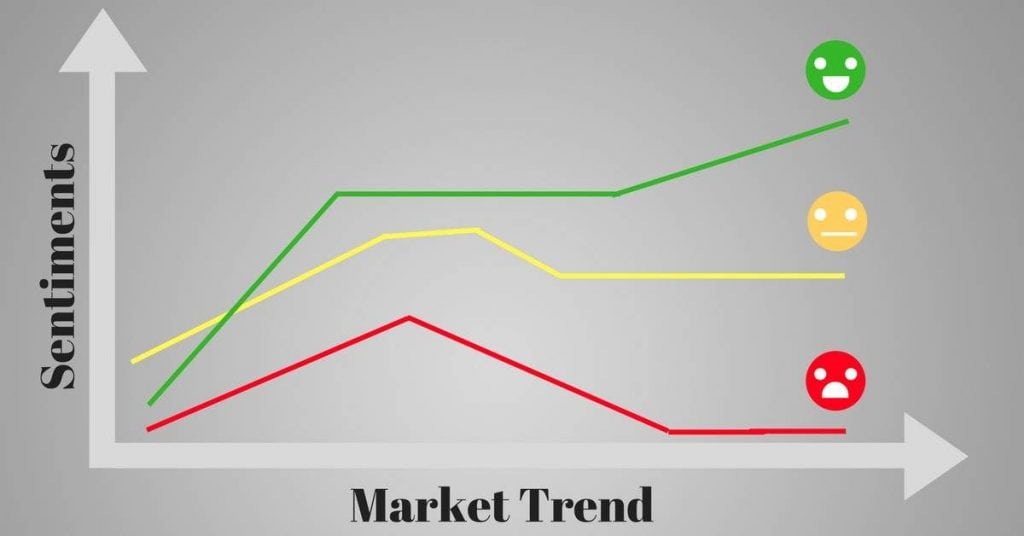
Operational Mechanism:
AI algorithms scrutinize news headlines, articles, blogs, forums, and social media to gauge bullish or bearish sentiment. NLP algorithms and machine learning models amalgamate these signals, enabling automated trades in alignment with prevailing sentiment.
Pros:
- Facilitates timely insights into evolving investor psychology and expectations.
- Bestows comprehensive data coverage through mainstream and social media analysis.
- Mitigates human cognitive biases.
Cons:
- Sentiment can oscillate swiftly, potentially leading to whipsaw movements.
- Not all information is tradable or market-moving.
- Requires proficient AI technology for accurate automation.
Implementation Recommendations:
- Blend sentiment signals with technical indicators for precise timing.
- Assign greater significance to renowned influencers and reputable sources.
- Track sentiment data across varied time frames.
- Personalize models by asset class and source reliability.
5. AI Algorithmic Hedging
Operational Mechanism:
AI systems examine relationships between asset classes, securities, and derivatives to discern effective hedging opportunities. Algorithms ascertain optimal hedging position size and timing, dynamically adapting portfolios to sustain the hedge as market conditions evolve.
Pros:
- Shields against losses during market downturns.
- Facilitates leveraged positions with minimized risk exposure.
- Automation thrives even in rapidly changing markets.
Cons:
- May cap profits in strongly trending markets.
- It demands intricate modeling and significant computational resources.
- Cumulative hedging costs may accrue over time.
Implementation Recommendations:
- Adopt a comprehensive portfolio approach rather than focusing solely on individual positions.
- Employ correlation analysis to identify assets with inverse relationships.
- Maintain optimal hedge ratios and recalibrate as necessitated by market dynamics.
- Steer clear of naked long or short positions bereft of corresponding hedges.
6. AI Statistical Arbitrage Trading
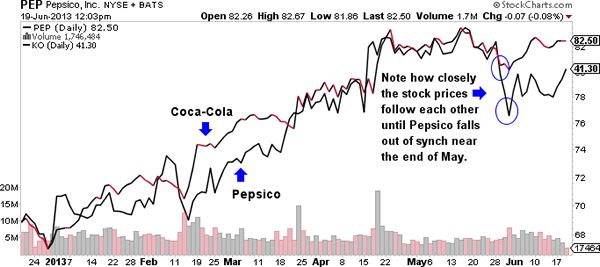
Operational Mechanism:
This high-frequency trading strategy strives to capitalize on short-term mispricings in correlated securities. AI algorithms vigilantly monitor pricing relationships between assets, such as stocks and their ETFs. Trades are promptly initiated upon detecting pricing discrepancies, leveraging millisecond execution speeds to exploit minute disparities.
Pros:
- Leverages AI’s pattern recognition prowess for generating signals.
- Accumulates modest yet predictable profits across high-volume trading.
- Maintains market neutrality with well-defined risk parameters.
Cons:
- Demands substantial transaction volume to generate profits.
- Opportunities are fleeting in high-speed markets.
- Large orders may incur market impact costs.
Implementation Recommendations:
- Implement this strategy with direct market access to ensure swift execution.
- Restrict positions to intraday durations to avert overnight risks.
- Precise execution is imperative in narrow arbitrage windows.
- Remain vigilant for indications of model overfitting.
7. AI Algorithmic Execution Trading
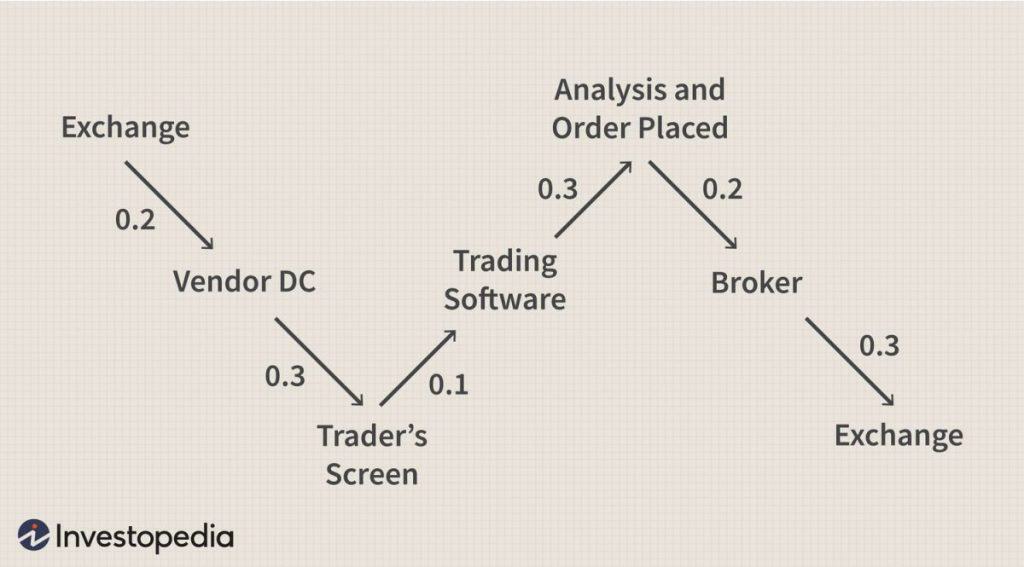
Operational Mechanism:
AI deploys its analytical prowess to enhance trade execution. It evaluates market liquidity, volatility, and microstructure to determine the optimal execution strategy. Large orders are subdivided into smaller segments for discreet execution, and trades are timed to mitigate costs and slippage. Self-learning algorithms continually refine execution performance.
Pros:
- Enhances trading efficiency and effectiveness.
- Reduces transaction costs, including fees and slippage.
- Capable of handling complex order types and restrictions.
- Delivers consistency in high-pressure trading scenarios.
Cons:
- Mandates a significant historical data repository for strategy development.
- Less effective for trading in low-liquidity securities.
- May underperform human traders in thinly traded markets.
Implementation Recommendations:
- Rigorously backtest algorithms using simulated orders to validate performance.
- Preferably employ proprietary data for training models, if accessible.
- Favor highly liquid instruments to optimize execution.
- Regularly update models to adapt to evolving market conditions.
8. AI Smart Order Routing
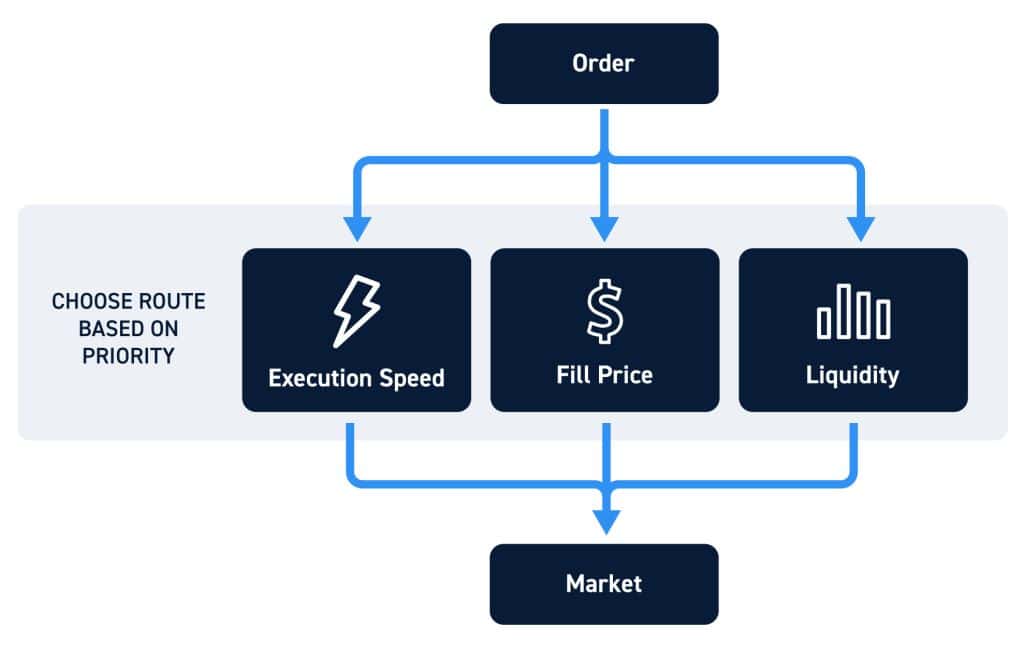
Operational Mechanism:
AI algorithms closely monitor and assess order book data across diverse exchanges and liquidity pools. Based on factors like order size, prices, and current market conditions, AI algorithms select the most advantageous venue for order execution. Orders are adeptly allocated across multiple destinations to minimize the disclosure of trading strategies, and self-learning models perpetually augment performance.
Pros:
- Diminishes delays in order fulfillment through judicious routing.
- Mitigates trading costs through price enhancement opportunities.
- Adapts seamlessly to shifting market dynamics.
- Eliminates the need for manual venue selection.
Cons:
- Entails complex integration across multiple exchanges and brokerage platforms.
- Demands comprehensive data resources for accurate liquidity modeling.
- Relies on third-party systems for real-time data feeds.
Implementation Recommendations:
- Harness order book data to forecast dynamic liquidity.
- Take into account factors such as speed, fees, and reject rates when analyzing venues.
- Assess trade-through regulations in fragmented markets.
- Implement randomized routing logic to safeguard against reverse engineering of strategies.
9. AI Event-Driven Trading
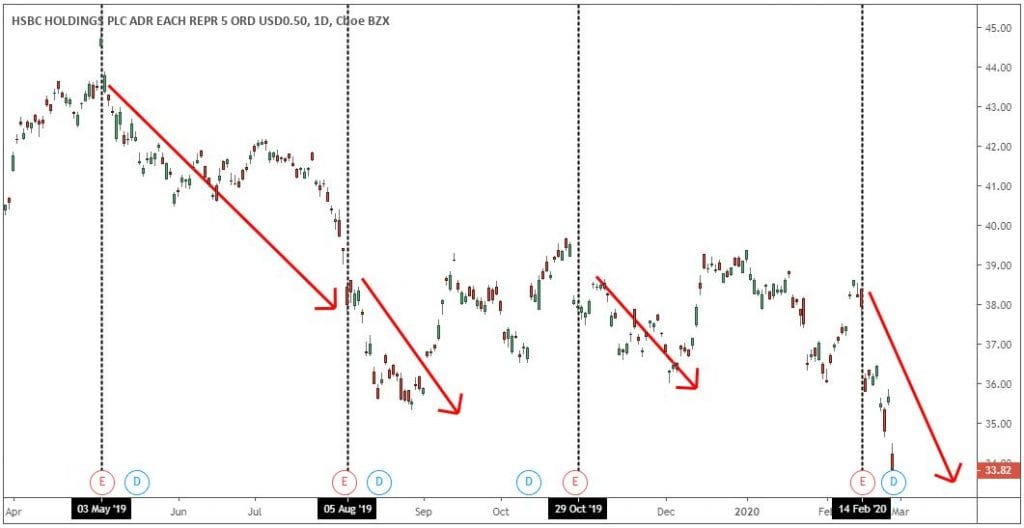
Operational Mechanism:
AI systems ingest and interpret vast quantities of news, earnings data, SEC filings, and economic releases. Actionable insights are extracted to predict potential market impacts. Trades are automatically executed to profit from anticipated price movements stemming from significant events.
Pros:
- Facilitates timely trading decisions aligned with market-altering events.
- Mitigates the influence of human cognitive biases.
- Effectively navigates intricate inter-market dynamics.
Cons:
- The accurate interpretation of all relevant information can be challenging.
- The news may be prematurely disseminated or anticipated by the markets.
- A high volume of spurious signals may arise from irrelevant events.
Implementation Recommendations:
- Fuse news analysis with technical indicators to enhance accuracy.
- Prioritize events with a demonstrated historical impact on markets.
- Maintain diversified portfolios to manage risk.
- Customize models based on industry, company, and event type.
10. AI/Human Collaborative Trading
Operational Mechanism:
This strategy amalgamates human creativity with AI’s computational prowess. Experienced traders harness AI for data analysis and pattern recognition. AI models enhance human trading decisions through automated signals, alerts, and analytics. Humans contribute creative inputs like strategy design, intuition, and market expertise.
Pros:
- Capitalizes on the strengths of both human intuition and data-driven AI models.
- Human oversight mitigates the risk of AI-based decisions influenced by faulty human biases.
- Enhances, rather than replaces, human traders.
Cons:
- Requires adeptness in synergizing human and AI capabilities.
- Possibility of human overrides based on erroneous biases.
- Sustaining a consistent, collaborative workflow can be challenging.
Implementation Recommendations:
- Retain human strategic oversight while using AI for execution.
- Reserve ultimate decision-making authority for human traders.
- Exploit AI to backtest and refine human-generated strategy concepts rapidly.
- Leverage AI to explore extensive datasets for expanded analysis.
The Pinnacle of AI Trading Systems
The successful implementation of these AI trading strategies necessitates specialized expertise. The optimal approach entails collaborating with established hedge funds, proprietary trading firms, or fintech vendors equipped with proven AI systems. The supremacy of artificial intelligence empowers traders to execute strategies with superhuman swiftness, precision, and analytical acumen.
While AI trading is still evolving, these technologies have exhibited remarkable potential for reshaping the landscape of investment and trading. As more entities adopt and innovate with AI, anticipate its integral role in capital markets and portfolio management. The competitive edge bestowed by AI algorithms implies that this technology is poised to become an indispensable capability for all serious market participants in the future.
Comparison of Key Features
When considering the application of AI in trading, it’s essential to keep these best practices in mind:
- Start small: Evaluate AI tools on paper trading or with small amounts of capital initially.
- Augment, don’t replace: Use AI to enhance existing processes rather than replacing them entirely.
- Combine AI with human insight: Algorithms lack common sense, so human oversight is crucial.
- Implement strong risk management: AI can learn bad habits, so risk controls are vital.
- Ensure transparency: Make AI decision-making transparent to build trust.
- Watch for overfitting: Rigorous out-of-sample testing is necessary to avoid this pitfall.
- Monitor for biases and ethical issues: Be aware of potential ethical concerns and hidden biases in AI models.
- Regularly retrain models: Markets evolve dynamically, so updating models with new data is essential.
Key Benefits of AI Trading
AI trading offers several advantages over traditional trading approaches:
- Speed: AI can process vast amounts of data and identify opportunities in microseconds, enabling the exploitation of short-lived inefficiencies.
- Accuracy: Sophisticated machine learning models can uncover complex patterns that human analysts may overlook, improving predictive accuracy.
- Adaptability: AI systems can continually update their strategies in dynamic environments, staying relevant.
- Scalability: AI can handle trading strategies across thousands of stocks, executing them tirelessly and without fatigue.
- Cost Savings: AI reduces the need for large, expensive analyst teams and lowers transaction costs through optimized trade execution.
Risks and Challenges of AI Trading
AI trading also comes with its share of risks and challenges:
- Overfitting: AI models may perform well in backtests but fail in live trading, requiring rigorous out-of-sample testing.
- Hidden biases: Training data biases can lead to suboptimal decisions that are not immediately apparent.
- Changing markets: Markets evolve, so AI models need periodic updates to avoid degradation.
- Transparency: Complex models like deep learning can behave like “black boxes” with low interpretability.
- Regulation: AI trading raises challenges around governance, disclosure, and accountability, necessitating regulatory guidance.
The Future of AI in Trading
AI is rapidly gaining traction in the trading and investment landscape. As algorithms become more powerful and accessible, AI will continue transforming how markets and participants operate. However, responsible oversight and governance will be critical to building trust and ensuring positive societal outcomes.
Traders seeking to leverage AI should begin by deeply understanding their strategy, data, and markets so they can apply AI judiciously to enhance their edge. With the right approach, AI can become a valuable addition rather than a black box prone to overpromising.
FAQs
AI algorithmic trading uses computer programs with automated rules and AI/ML to make trading decisions, place orders, and manage trades with minimal human intervention.
AI provides speed and precision in data analysis, pattern recognition, order execution, risk management, and other aspects that human traders cannot match. This gives an edge to AI trading strategies.
Potential risks include overfitting models to historical data, coding errors in algorithms, excessive trading, and susceptibility to flash crashes and volatility. Proper development, testing, and risk controls are essential.
Successful development requires expertise in AI/machine learning, quant trading strategies, market microstructure, data science, backtesting, coding, and predictive analytics. A multidisciplinary team is ideal.
A: Traders can either build in-house AI capabilities, purchase off-the-shelf AI trading platforms, or invest through hedge funds and trading firms with established AI trading infrastructure.
AI is expected to become integral to capital markets and trading as adoption grows. The competitive advantages provided by AI will likely become essential for all serious traders in the future.
Read more related topics:
Disclaimer
In line with the Trust Project guidelines, please note that the information provided on this page is not intended to be and should not be interpreted as legal, tax, investment, financial, or any other form of advice. It is important to only invest what you can afford to lose and to seek independent financial advice if you have any doubts. For further information, we suggest referring to the terms and conditions as well as the help and support pages provided by the issuer or advertiser. MetaversePost is committed to accurate, unbiased reporting, but market conditions are subject to change without notice.
About The Author
Damir is the team leader, product manager, and editor at Metaverse Post, covering topics such as AI/ML, AGI, LLMs, Metaverse, and Web3-related fields. His articles attract a massive audience of over a million users every month. He appears to be an expert with 10 years of experience in SEO and digital marketing. Damir has been mentioned in Mashable, Wired, Cointelegraph, The New Yorker, Inside.com, Entrepreneur, BeInCrypto, and other publications. He travels between the UAE, Turkey, Russia, and the CIS as a digital nomad. Damir earned a bachelor's degree in physics, which he believes has given him the critical thinking skills needed to be successful in the ever-changing landscape of the internet.
More articles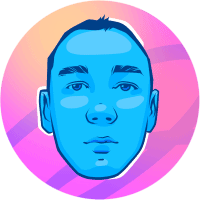
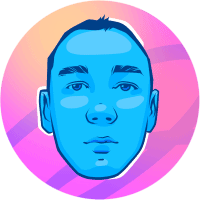
Damir is the team leader, product manager, and editor at Metaverse Post, covering topics such as AI/ML, AGI, LLMs, Metaverse, and Web3-related fields. His articles attract a massive audience of over a million users every month. He appears to be an expert with 10 years of experience in SEO and digital marketing. Damir has been mentioned in Mashable, Wired, Cointelegraph, The New Yorker, Inside.com, Entrepreneur, BeInCrypto, and other publications. He travels between the UAE, Turkey, Russia, and the CIS as a digital nomad. Damir earned a bachelor's degree in physics, which he believes has given him the critical thinking skills needed to be successful in the ever-changing landscape of the internet.