Meta AI and Paperswithcode release first AI model Galactica trained on scientific texts
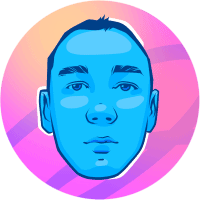
In Brief
Meta launches first 120B model Galactica for scientific texts
Galactica models can be used for science-related tasks like finding citations
Meta AI and Paperswithcode have recently released the first 120B model Galactica that is trained on scientific texts (articles, textbooks, etc.). This is a huge breakthrough in the field of AI, as it will allow for more accurate and faster predictions to be made about the world around us.
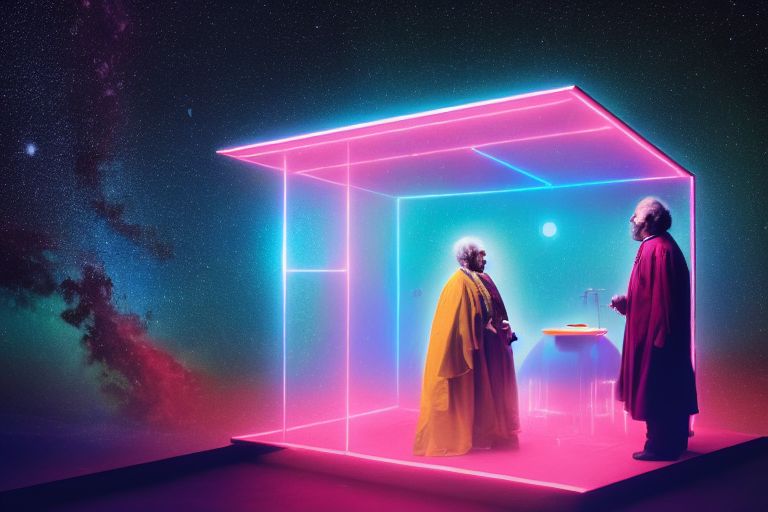
Scientific essays, lectures, formulas, abstracts, and even computer notebooks are just a few of the wonderful things that Galactica can produce. The weights and code for the model are completely open-source.
The problem is that information overload in science was the initial promise of computing. The specialty of traditional computers, however, was retrieval and storage rather than pattern recognition. As a result, while their ability to digest data has increased, their intellect has not.
The main goal of Galactica is to help researchers who are drowning in publications and finding it harder and harder to separate the important from the irrelevant.
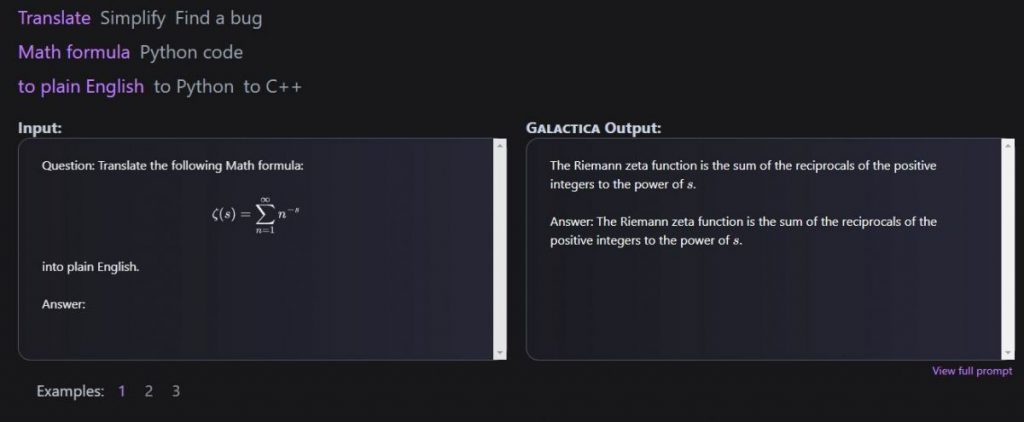
It can be used for many things, including reading academic material, posing scientific queries, and writing scientific code.
- Galactica is a potent large language model (LLM) that has been trained on more than 48 million scientific papers, books, articles, chemicals, proteins, and other sources. More than 360 million in-context citations and over 50 million unique references, normalized across a wide range of sources, make up the huge corpus on which Galactica models are trained. Galactica may then recommend citations and find related publications because of this.
- Meta has announced a slew of new AI models and tools in recent months, including AI news editors or text-to-video generators.
Read more related articles:
Disclaimer
In line with the Trust Project guidelines, please note that the information provided on this page is not intended to be and should not be interpreted as legal, tax, investment, financial, or any other form of advice. It is important to only invest what you can afford to lose and to seek independent financial advice if you have any doubts. For further information, we suggest referring to the terms and conditions as well as the help and support pages provided by the issuer or advertiser. MetaversePost is committed to accurate, unbiased reporting, but market conditions are subject to change without notice.
About The Author
Damir is the team leader, product manager, and editor at Metaverse Post, covering topics such as AI/ML, AGI, LLMs, Metaverse, and Web3-related fields. His articles attract a massive audience of over a million users every month. He appears to be an expert with 10 years of experience in SEO and digital marketing. Damir has been mentioned in Mashable, Wired, Cointelegraph, The New Yorker, Inside.com, Entrepreneur, BeInCrypto, and other publications. He travels between the UAE, Turkey, Russia, and the CIS as a digital nomad. Damir earned a bachelor's degree in physics, which he believes has given him the critical thinking skills needed to be successful in the ever-changing landscape of the internet.
More articles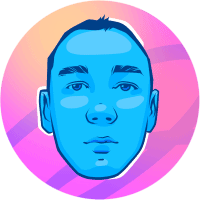
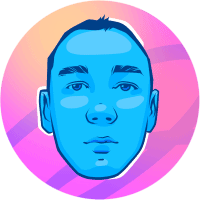
Damir is the team leader, product manager, and editor at Metaverse Post, covering topics such as AI/ML, AGI, LLMs, Metaverse, and Web3-related fields. His articles attract a massive audience of over a million users every month. He appears to be an expert with 10 years of experience in SEO and digital marketing. Damir has been mentioned in Mashable, Wired, Cointelegraph, The New Yorker, Inside.com, Entrepreneur, BeInCrypto, and other publications. He travels between the UAE, Turkey, Russia, and the CIS as a digital nomad. Damir earned a bachelor's degree in physics, which he believes has given him the critical thinking skills needed to be successful in the ever-changing landscape of the internet.