Sber AI has presented Kandinsky 2.0, the first text-to-image model for generating in more than 100 languages
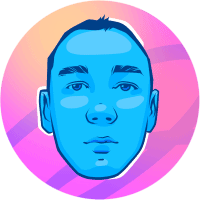
In Brief
Kandinsky 2.0, the first multilingual diffusion model, was created and trained by Sber AI researchers with the assistance of researchers from the AI Institute of Artificial Intelligence using the combined dataset of 1 billion text-image pairs from Sber AI and SberDevices
Diffusion is increasingly replacing GANs and autoregressive models in a number of digital image processing tasks. This is not surprising because diffusion is easier to learn, does not require a complex selection of hyperparameters, min-max optimization, and does not suffer from learning instability. And most importantly, diffusion models demonstrate state-of-the-art results on almost all generative tasks — image generation by text, sound generation, video, and even 3D.
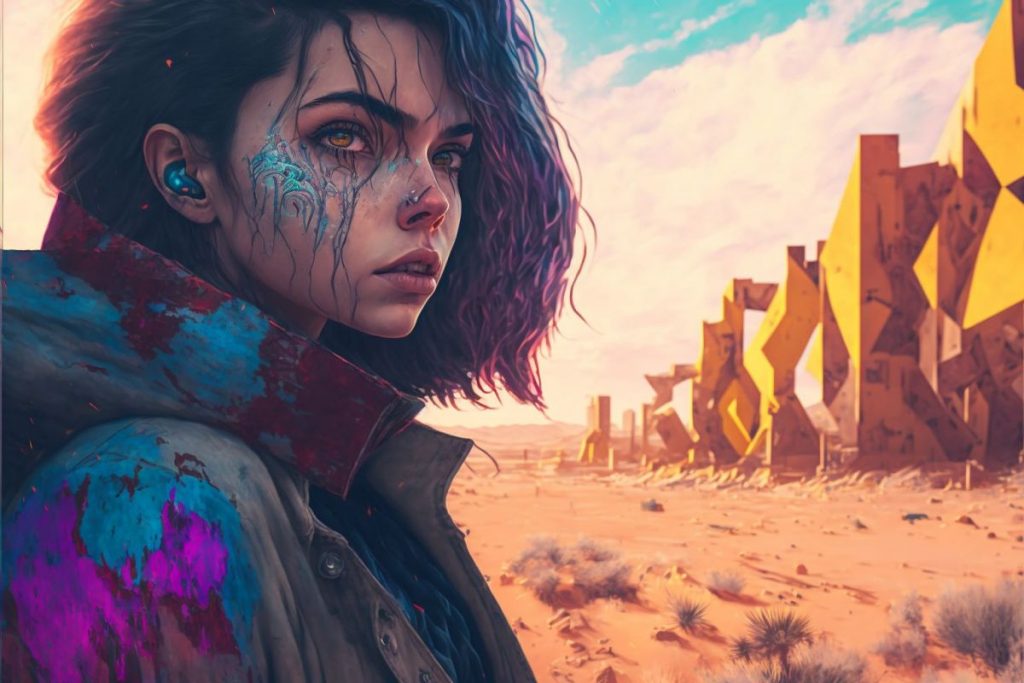
Unfortunately, most of the work in the field of text-to-something focuses only on English and Chinese. To correct this injustice, Sber AI decided to create a multilingual text-to-image diffusion model Kandinsky 2.0, which understands queries in more than 100 languages. HuggingFace already offers Kandinsky 2.0. Researchers from SberAI and SberDevices have collaborated with experts from the AI Institute of Artificial Intelligence on this project.
What is diffusion?
In the 2015 article Deep Unsupervised Learning using Nonequilibrium Thermodynamics, diffusion models were first described as the act of mixing a substance resulting in diffusion, which equalizes the distribution. As the title of the article implies, they approached the explanation of diffusion models through the framework of thermodynamics.
In the case of images, such a process might resemble, for instance, gradually removing Gaussian noise from the image.
The paper Diffusion Models Beat GANs on Image Synthesis, published in 2021, was the first to show the superiority of diffusion models over GANS. The authors also devised the first-generation control approach (conditioning), which they named classifier guidance. This method creates objects that fit the intended class using gradients from a different classifier (for example, dogs). Through the Adaptive Group Norm mechanism, which involves the forecasting of normalization coefficients, the control itself is carried out.
This article can be seen as a turning point in the field of generative AI, leading many to turn to the study of diffusion. New articles about text-to-video, text-to-3D, image inpainting, audio generation, diffusion for superresolution, and even motion generation started to appear every few weeks.
Text-to-image diffusion
As we mentioned earlier, noise reduction and noise elimination are typically the main components of diffusion processes in the context of image modalities, so UNet and its many variations are frequently used as the fundamental architecture.
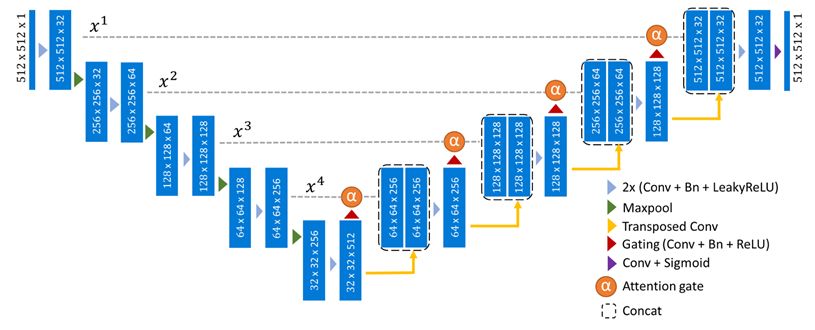
It is essential that this text be taken into consideration in some way during the generation in order to create an image based on it. The authors of the OpenAI article on the GLIDE model suggested modifying the classifier-free guidance approach for text.
The employment of frozen pre-irradiated text encoders and the cascade resolution enhancement mechanism in the future considerably improved text production (Imagen). It turned out that there was no need to train the text portion of text-to-image models as using the frozen T5-xxl resulted in considerably improved image quality and text comprehension and used much fewer training resources.
The authors of a Latent Diffusion article demonstrated that the picture component actually does not require training (at least not completely). Learning will proceed even more quickly if we use a powerful image autoencoder (VQ-VAE or KL-VAE) as a visual decoder and attempt to generate embeddings from its latent space by diffusion rather than the image itself. This methodology is also the foundation of the recently released Stable Diffusion model.
Kandinsky 2.0 AI model
With a few key improvements, Kandinsky 2.0 is based on an enhanced Latent Diffusion technique (we do not make images, but rather their latent vectors):
- Employed two multilingual text encoders and concatenated their embeddings.
- Added UNet (1.2 billion parameters).
- Sampling procedure dynamic thresholding.
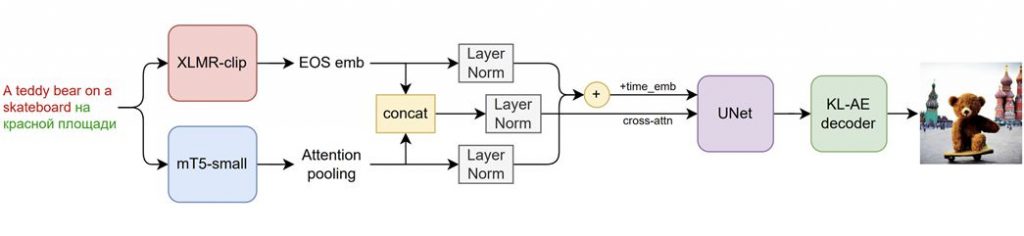
Researchers employed two multilingual encoders simultaneously—XLMR-clip and mT5-small—in order to make the model really multilingual. Therefore, in addition to English, Russian, French, and German, the model can also understand languages like Mongolian, Hebrew, and Farsi. The AI knows a total of 101 languages. Why was it decided to encode text using two models simultaneously? Since XLMR-clip has seen pictures and provides close embeddings for various languages, and mT5-small is capable of understanding complex texts, these models have different but crucial features. Since both models only have a small number of parameters (560M and 146M), as demonstrated by our preliminary tests, it was decided to use two encoders simultaneously.
Freshly generated images by Kandinsky 2.0 AI model below:
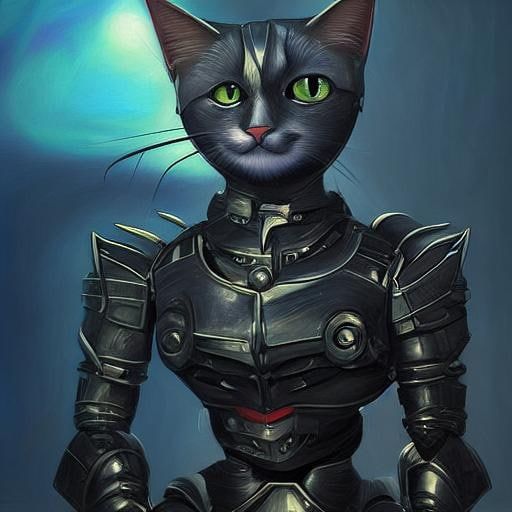
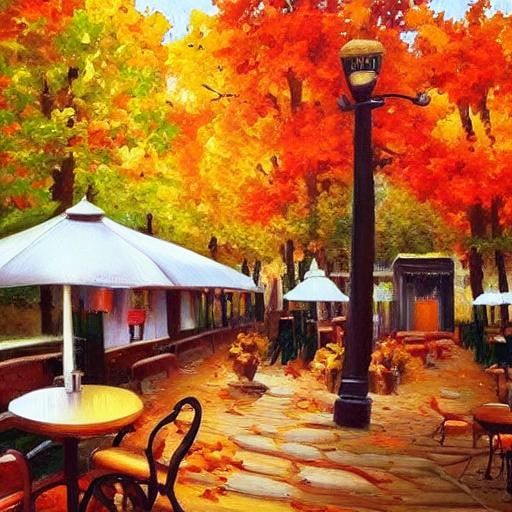
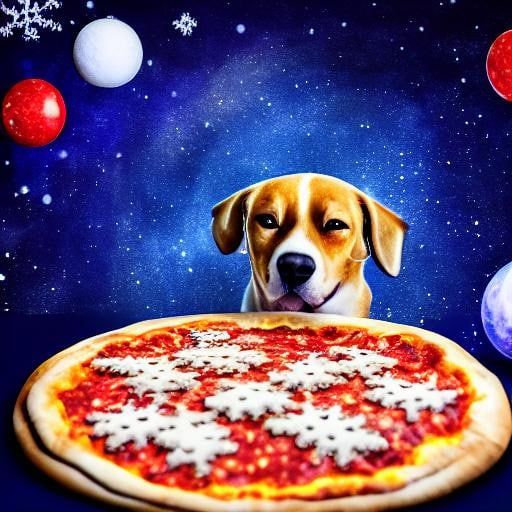
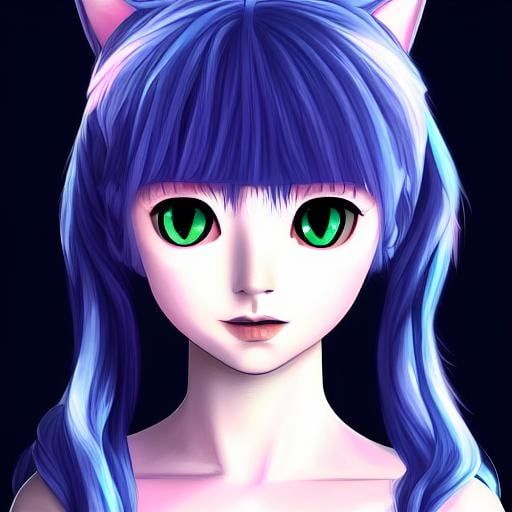
How was the Kandinsky 2.0 model training done?
Christofari supercomputers were utilized for the training on the ML Space platform. It required 196 NVIDIA A100 cards, each with 80 GB of RAM. It took 14 days, or 65,856 GPU-hours, to complete the training. The analysis took five days at 256×256 resolution, followed by six days at 512×512 resolution, then additional three days on the purest data.
As training data, many datasets were combined that had been pre-filtered for watermarks, low resolution, and low adherence to the text description as measured by the CLIP-score metric.
Multilingual generation
Kandinsky 2.0 is the first multilingual model for creating images from words, giving us the first chance to assess linguistic and visual changes across language cultures. The outcomes of translating the same query into several languages are shown below. For instance, only white men appear in the generation results for the Russian query “a person with a higher education,” while the results for the French translation, “Photo d’une personne diplômée de l’enseignement supérieur,” are more diversified. I would like to point out that the sorrowful folks with higher education are only present in the Russian-language edition.
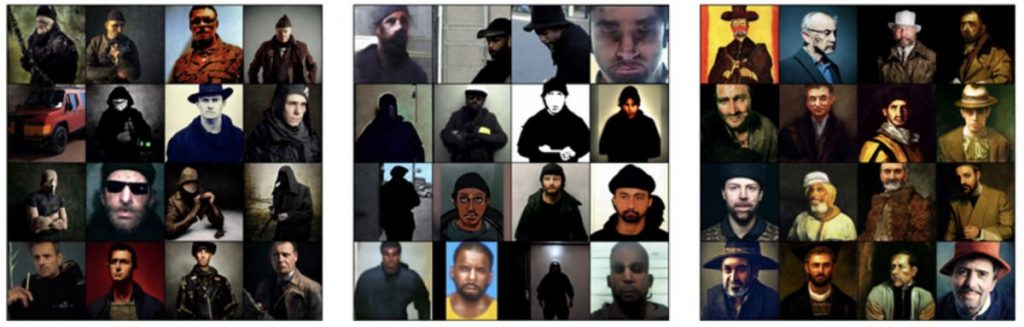
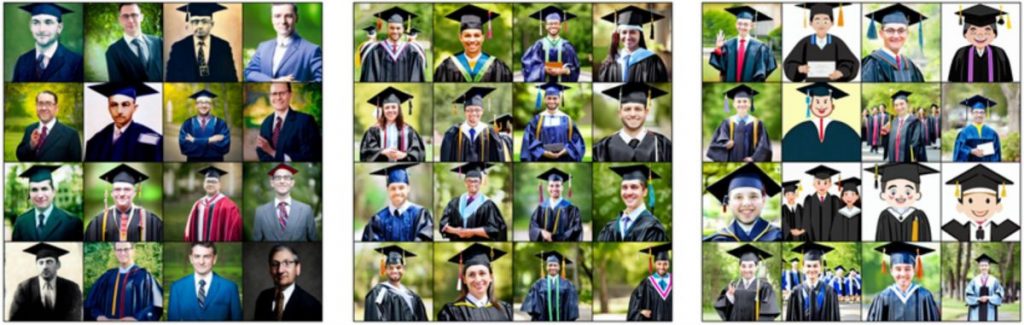
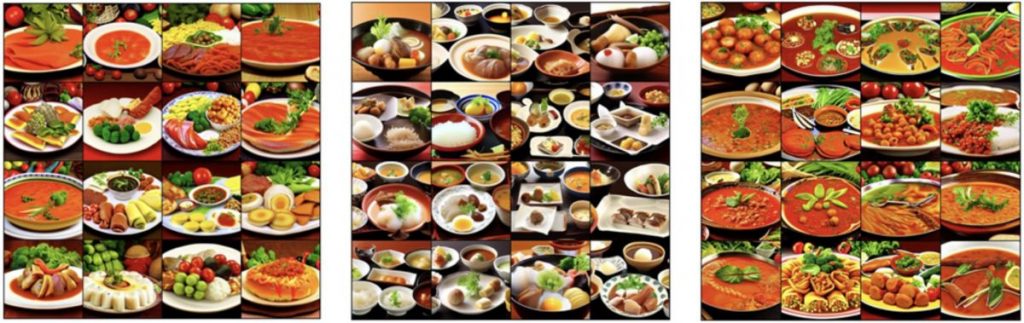
Although there are still a ton of trials with huge language models and different methods of the diffusion process planned, we can already state with confidence that Kandinsky 2.0 is the first totally multilingual dissemination model! On the FusionBrain website and Google Colab, you may see examples of her drawings.
Read more about AI:
Disclaimer
In line with the Trust Project guidelines, please note that the information provided on this page is not intended to be and should not be interpreted as legal, tax, investment, financial, or any other form of advice. It is important to only invest what you can afford to lose and to seek independent financial advice if you have any doubts. For further information, we suggest referring to the terms and conditions as well as the help and support pages provided by the issuer or advertiser. MetaversePost is committed to accurate, unbiased reporting, but market conditions are subject to change without notice.
About The Author
Damir is the team leader, product manager, and editor at Metaverse Post, covering topics such as AI/ML, AGI, LLMs, Metaverse, and Web3-related fields. His articles attract a massive audience of over a million users every month. He appears to be an expert with 10 years of experience in SEO and digital marketing. Damir has been mentioned in Mashable, Wired, Cointelegraph, The New Yorker, Inside.com, Entrepreneur, BeInCrypto, and other publications. He travels between the UAE, Turkey, Russia, and the CIS as a digital nomad. Damir earned a bachelor's degree in physics, which he believes has given him the critical thinking skills needed to be successful in the ever-changing landscape of the internet.
More articles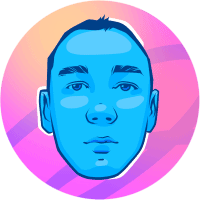
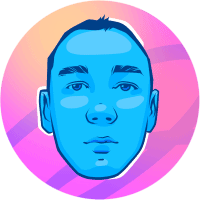
Damir is the team leader, product manager, and editor at Metaverse Post, covering topics such as AI/ML, AGI, LLMs, Metaverse, and Web3-related fields. His articles attract a massive audience of over a million users every month. He appears to be an expert with 10 years of experience in SEO and digital marketing. Damir has been mentioned in Mashable, Wired, Cointelegraph, The New Yorker, Inside.com, Entrepreneur, BeInCrypto, and other publications. He travels between the UAE, Turkey, Russia, and the CIS as a digital nomad. Damir earned a bachelor's degree in physics, which he believes has given him the critical thinking skills needed to be successful in the ever-changing landscape of the internet.