Best 10 Graphics Cards for ML/AI: Top GPU for Deep Learning
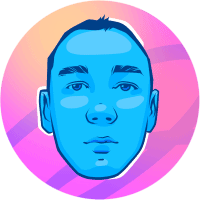
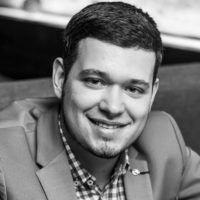
The selection of an appropriate graphics card plays a crucial role in achieving optimal performance for processing large datasets and conducting parallel computations. Particularly for tasks involving deep neural network training, the demand for intensive matrix and tensor processing is paramount. It is worth noting that specialized AI chips, TPUs, and FPGAs have gained considerable popularity in recent times.
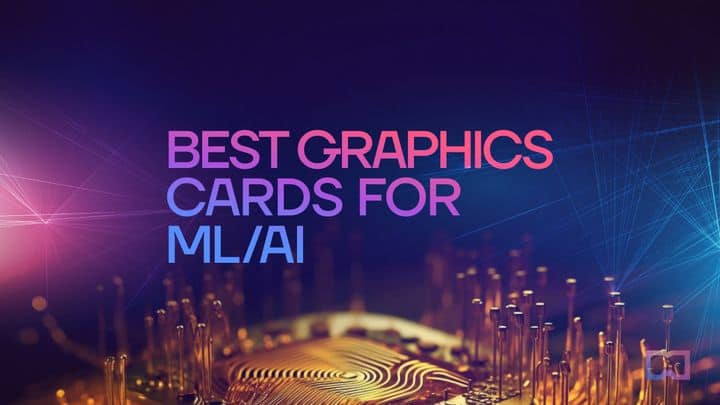
Key Characteristics for Machine Learning Graphics Cards
When considering a graphics card for machine learning purposes, several essential features should be taken into account:
- Computing Power:
The number of cores or processors directly impacts the parallel processing capabilities of the graphics card. A higher core count translates to faster and more efficient computations. - GPU Memory Capacity:
Ample memory capacity is crucial for effectively handling large datasets and complex models. The ability to efficiently store and access data is vital for achieving optimal performance. - Support for Specialized Libraries:
Hardware compatibility with specialized libraries like CUDA or ROCm can significantly accelerate model training processes. Leveraging hardware-specific optimizations streamlines computations and enhances overall efficiency. - High-Performance Support:
Graphics cards with fast memory and wide memory bus configurations deliver high-performance capabilities during model training. These features ensure smooth and rapid data processing. - Compatibility with Machine Learning Frameworks:
Ensuring seamless compatibility between the selected graphics card and the employed machine learning frameworks and developer tools is essential. Compatibility guarantees smooth integration and optimal utilization of resources.
Comparison Table of Graphics Cards for ML/AI
Graphics Card | Memory, GB | CUDA Cores | Tensor Cores | Price, USD |
---|---|---|---|---|
Tesla V100 | 16/32 | 5120 | 640 | 14,999 |
Tesla A100 | 40/80 | 7936 | 432 | 10,499 |
Quadro RTX 8000 | 48 | 4608 | 576 | 7,999 |
A 6000 Ada | 48 | 18176 | 568 | 6,499 |
RTX A 5000 | 24 | 8192 | 256 | 1,899 |
RTX 3090 TI | 24 | 10752 | 336 | 1,799 |
RTX 4090 | 24 | 16384 | 512 | 1,499 |
RTX 3080 TI | 12 | 10240 | 320 | 1,399 |
RTX 4080 | 16 | 9728 | 304 | 1,099 |
RTX 4070 | 12 | 7680 | 184 | 599 |
NVIDIA Tesla V100
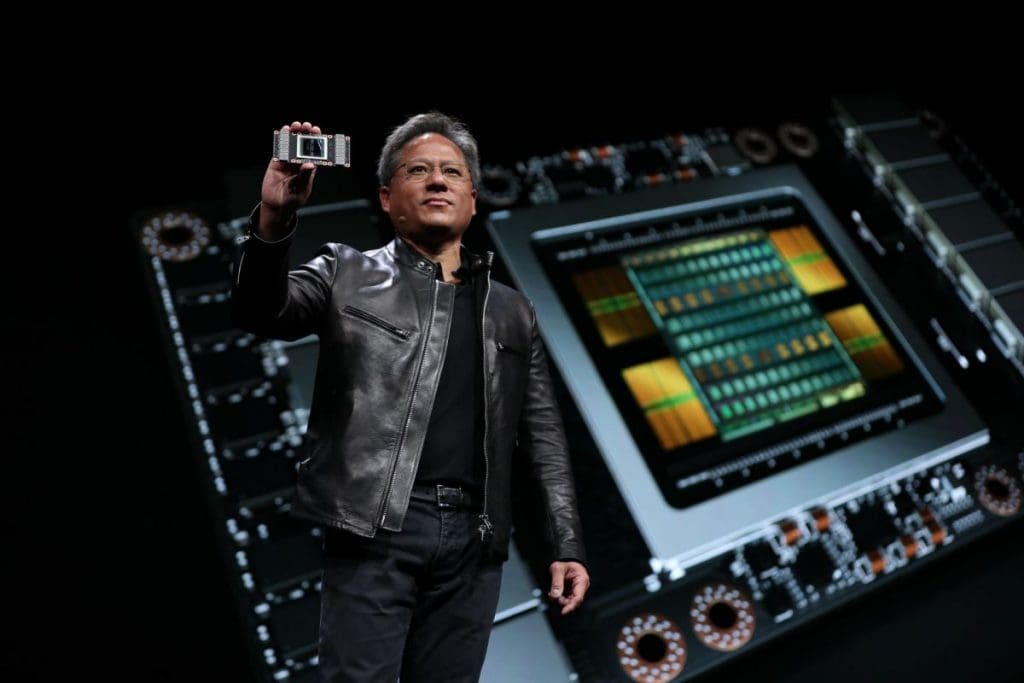
NVIDIA’s Tesla V100 stands as a powerful Tensor Core GPU tailored for AI, High Performance Computing (HPC), and Machine Learning workloads. Leveraging the cutting-edge Volta architecture, this graphics card showcases outstanding performance capabilities, delivering an impressive 125 trillion floating point operations per second (TFLOPS) performance. In this article, we will explore the notable benefits and considerations associated with the Tesla V100.
Pros of Tesla V100:
- High Performance:
Harnessing the power of the Volta architecture and its 5120 CUDA cores, the Tesla V100 offers exceptional performance for machine learning tasks. Its ability to handle extensive datasets and execute complex calculations at remarkable speeds is instrumental in driving efficient machine learning workflows. - Large Memory Capacity:
With 16 GB of HBM2 memory, the Tesla V100 empowers efficient processing of substantial data volumes during model training. This attribute is particularly advantageous when working with extensive datasets, allowing for seamless data manipulation. Moreover, the video memory bus width of 4096 bits facilitates high-speed data transfer between the processor and video memory, further enhancing the performance of machine learning model training and inference. - Deep Learning Technologies:
The Tesla V100 is equipped with various deep learning technologies, including Tensor Cores, which expedite floating point calculations. This acceleration contributes to significant reductions in model training time, ultimately enhancing overall performance. - Flexibility and Scalability:
The versatility of the Tesla V100 is evident in its compatibility with both desktop and server systems. It seamlessly integrates with a wide range of machine learning frameworks such as TensorFlow, PyTorch, Caffe, and more, offering developers the freedom to choose their preferred tools for model development and training.
Considerations for Tesla V100:
- High Cost:
As a professional-grade solution, the NVIDIA Tesla V100 bears a corresponding price tag. With a cost of $14,447, it may present a substantial investment for individuals or small machine learning teams. The pricing should be taken into account when considering the overall budget and requirements. - Power Consumption and Cooling:
Given the Tesla V100’s robust performance, it demands a significant power supply and generates substantial heat. Adequate cooling measures need to be implemented to maintain optimal operating temperatures, which can lead to increased energy consumption and associated costs. - Infrastructure Requirements:
To fully leverage the capabilities of the Tesla V100, a compatible infrastructure is necessary. This includes a powerful processor and sufficient RAM to ensure efficient data processing and model training.
Conclusion:
The NVIDIA A100, powered by the cutting-edge Ampere architecture, represents a significant leap forward in GPU technology for machine learning applications. With its high-performance capabilities, large memory capacity, and support for NVLink technology, the A100 empowers data scientists and researchers to tackle complex machine learning tasks with efficiency and precision. However, the high cost, power consumption, and software compatibility should be carefully evaluated before adopting the NVIDIA A100. With its advancements and breakthroughs, the A100 opens up new possibilities for accelerated model training and inference, paving the way for further advancements in the field of machine learning.
NVIDIA Tesla A100
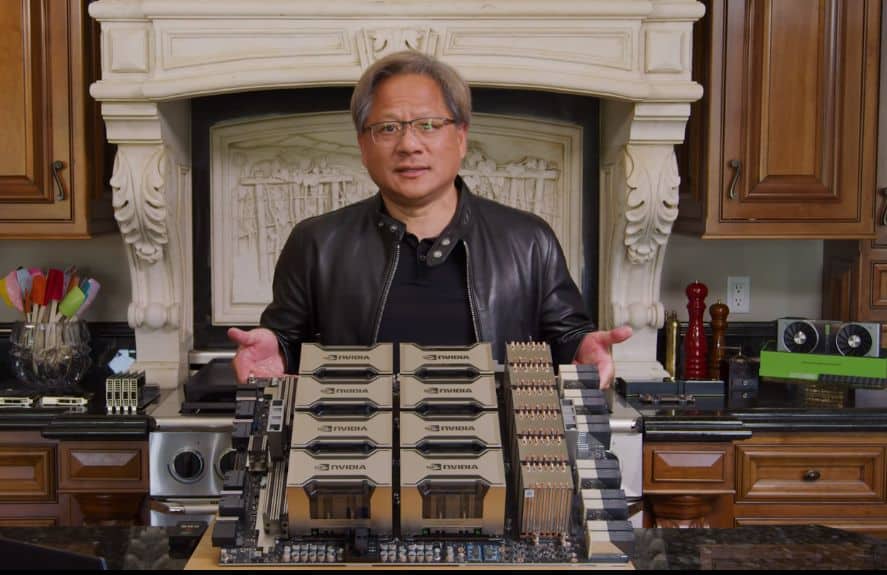
The NVIDIA A100, powered by the state-of-the-art Ampere architecture, stands as a remarkable graphics card designed to meet the demands of machine learning tasks. Offering exceptional performance and flexibility, the A100 represents a significant advancement in GPU technology. In this article, we will explore the notable benefits and considerations associated with the NVIDIA A100.
Pros of NVIDIA A100:
- High Performance:
Equipped with a substantial number of CUDA cores (4608), the NVIDIA A100 delivers impressive performance capabilities. Its enhanced computational power enables accelerated machine learning workflows, resulting in faster model training and inference processes. - Large Memory Capacity:
The NVIDIA A100 graphics card boasts 40 GB of HBM2 memory, facilitating efficient handling of vast amounts of data during deep learning model training. This large memory capacity is particularly advantageous for working with complex and large-scale datasets, enabling smooth and seamless data processing. - Support for NVLink Technology:
The inclusion of NVLink technology enables multiple NVIDIA A100 graphics cards to be seamlessly combined into a single system, facilitating parallel computing. This enhanced parallelism significantly improves performance and accelerates model training, contributing to more efficient machine learning workflows.
Considerations for NVIDIA A100:
- High Cost:
As one of the most powerful and advanced graphics cards available on the market, the NVIDIA A100 comes with a higher price tag. Priced at $10,000, it may be a substantial investment for individuals or organizations considering its adoption. - Power Consumption:
Utilizing the full potential of the NVIDIA A100 graphics card requires a significant power supply. This may lead to increased energy consumption and necessitate appropriate measures for power management, especially when deploying the card in large-scale data centers. - Software Compatibility:
To ensure optimal performance, the NVIDIA A100 relies on appropriate software and drivers. It is important to note that some machine learning programs and frameworks may not fully support this specific graphics card model. Compatibility considerations should be taken into account when integrating the NVIDIA A100 into existing machine learning workflows.
Conclusion:
The Tesla V100, with its Volta architecture and cutting-edge features, stands as an impressive Tensor Core GPU designed for AI, HPC, and Machine Learning workloads. Its high-performance capabilities, large memory capacity, deep learning technologies, and flexibility make it a compelling choice for organizations and researchers pursuing advanced machine learning projects. However, considerations such as the cost, power consumption, and infrastructure requirements must be carefully evaluated to ensure a well-aligned investment. With the Tesla V100, the potential for breakthroughs in AI and machine learning is within reach, empowering data scientists and researchers to push the boundaries of innovation.
NVIDIA Quadro RTX 8000
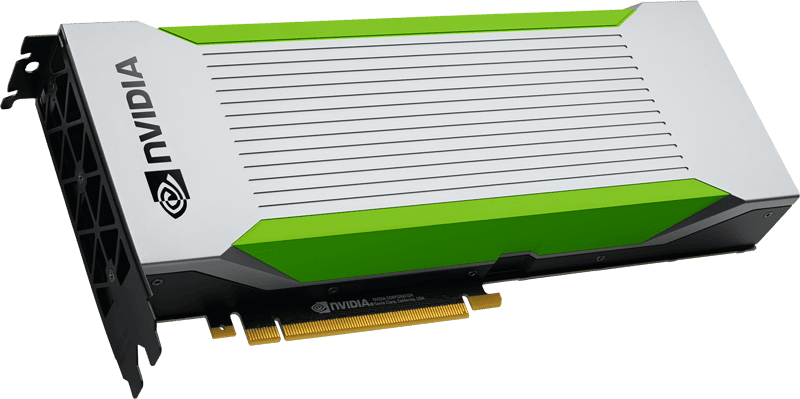
The Quadro RTX 8000 stands as a powerful graphics card designed specifically for professionals in need of exceptional rendering capabilities. With its advanced features and high-performance specifications, this graphics card offers practical benefits for various applications, including data visualization, computer graphics, and machine learning. In this article, we will explore the distinguishing features and advantages of the Quadro RTX 8000.
Pros of Quadro RTX 8000:
- High Performance:
The Quadro RTX 8000 boasts a powerful GPU and an impressive 5120 CUDA cores, providing unparalleled performance for demanding rendering tasks. Its exceptional computational power enables professionals to render complex models with realistic shadows, reflections, and refractions, delivering realistic and immersive visual experiences. - Ray Tracing Support:
Hardware-accelerated ray tracing is a standout feature of the Quadro RTX 8000. This technology enables the creation of photorealistic images and realistic lighting effects. For professionals engaged in data visualization, computer graphics, or machine learning, this feature adds a level of realism and visual fidelity to their work, enhancing the overall quality of their projects. - Large Memory Capacity:
The Quadro RTX 8000 offers an ample 48GB of GDDR6 graphics memory. This large memory capacity allows for efficient storage and retrieval of data, particularly when working with large-scale machine learning models and datasets. Professionals can perform complex computations and handle substantial amounts of data without compromising performance or efficiency. - Library and Framework Support:
Compatibility with popular machine learning libraries and frameworks, including TensorFlow, PyTorch, CUDA, cuDNN, and more, ensures seamless integration into existing workflows. Professionals can leverage the power of the Quadro RTX 8000 with their preferred tools and frameworks, enabling efficient development and training of machine learning models.
Considerations for Quadro RTX 8000:
- High Cost:
As a professional graphics accelerator, the Quadro RTX 8000 comes with a higher price tag compared to other graphics cards. Its actual cost is $8,200, which may make it less accessible for individual users or small-scale operations.
Conclusion:
The Quadro RTX 8000 sets a benchmark for high-performance graphics rendering in professional applications. With its powerful GPU, ray tracing support, large memory capacity, and compatibility with popular machine learning libraries and frameworks, the Quadro RTX 8000 empowers professionals to create visually stunning and realistic models, visualizations, and simulations. While the higher cost may pose a challenge for some, the benefits of this graphics card make it a valuable asset for professionals in need of top-tier performance and memory capacity. With the Quadro RTX 8000, professionals can unlock their creative potential and push the boundaries of their work in the fields of data visualization, computer graphics, and machine learning.
NVIDIA RTX A6000 Ada
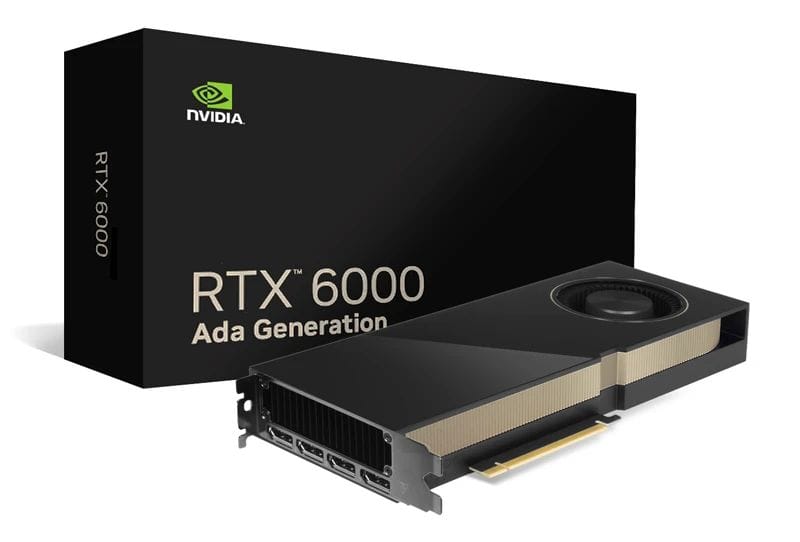
The RTX A6000 Ada graphics card stands out as a compelling option for professionals in search of a powerful yet energy-efficient solution. With its advanced features, including the Ada Lovelace architecture, high-performance CUDA cores, and ample VRAM capacity, the RTX A6000 Ada offers practical benefits for a variety of professional applications. In this article, we will explore the distinguishing features and advantages of the RTX A6000 Ada.
Pros of RTX A6000 Ada:
- High Performance:
The RTX A6000 Ada harnesses the power of the Ada Lovelace architecture, incorporating third-generation RT cores, fourth-generation Tensor Cores, and next-generation CUDA cores. These advancements in architecture contribute to outstanding performance, enabling professionals to tackle demanding tasks with ease. With 48GB of VRAM, the graphics card provides ample memory for efficient handling of large datasets during model training. - Large Memory Capacity:
Equipped with 48GB of memory, the RTX A6000 Ada ensures efficient processing of large volumes of data. This expansive memory capacity empowers professionals to train complex machine learning models and work with massive datasets without compromising performance or efficiency. The ability to handle extensive data loads contributes to faster and more accurate model training. - Low Power Consumption:
The RTX A6000 Ada places emphasis on energy efficiency, making it an ideal choice for professionals conscious of power consumption. By optimizing power usage, this graphics card reduces energy costs and contributes to a more sustainable and cost-effective work environment.
Considerations for RTX A6000 Ada:
- High Cost:
The RTX A6000 Ada, with its impressive performance and features, does come at a higher price point. Priced at approximately $6,800, this graphics card may be a significant investment for individual users or smaller organizations. However, the superior performance and efficiency it offers justify its value for professionals seeking optimal results.
Conclusion:
The RTX A6000 Ada emerges as an outstanding graphics card choice for professionals aiming to maximize performance and efficiency. With its advanced architecture, substantial VRAM capacity, and low power consumption, this graphics card delivers exceptional results across a range of professional applications. The Ada Lovelace architecture, coupled with next-generation CUDA cores and high memory capacity, ensures high-performance computing and efficient handling of large datasets. While the RTX A6000 Ada does come with a higher cost, its benefits and capabilities make it a valuable asset for professionals committed to achieving optimal outcomes in their work. With the RTX A6000 Ada, professionals can unlock their full potential and elevate their performance in various domains, including machine learning, data analysis, and computer graphics.
NVIDIA RTX A5000
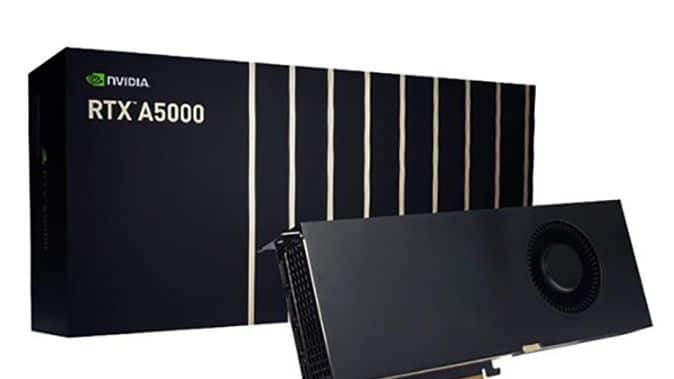
The RTX A5000, built on the NVIDIA Ampere architecture, emerges as a powerful graphics card designed to accelerate machine learning tasks. With its robust features and high-performance capabilities, the RTX A5000 offers practical benefits and distinct advantages for professionals in the field. In this article, we will delve into the distinguishing features of the RTX A5000 and its potential impact on machine learning applications.
Pros of RTX A5000:
- High Performance:
Equipped with 8192 CUDA cores and 256 tensor cores, the RTX A5000 boasts exceptional processing power. This high-performance architecture allows for rapid and efficient processing of large datasets, enabling faster training of machine learning models. The abundance of CUDA cores and high memory bandwidth contribute to smooth and accelerated computations, facilitating complex operations within machine learning workflows. - AI Hardware Acceleration Support:
The RTX A5000 graphics card provides hardware acceleration for AI-related operations and algorithms. With its optimized design, the card can significantly enhance the performance of AI tasks, delivering faster and more efficient results. By leveraging the power of dedicated AI hardware acceleration, professionals can streamline their machine learning workflows and achieve enhanced productivity. - Large Memory Capacity:
Featuring 24GB of GDDR6 VRAM, the RTX A5000 offers ample memory for handling large datasets and complex machine learning models. This extensive memory capacity enables professionals to work with data-intensive tasks without compromising performance or efficiency. The availability of abundant VRAM ensures smooth data access and faster training, allowing for more accurate and comprehensive model development. - Machine Learning Framework Support:
The RTX A5000 seamlessly integrates with popular machine learning frameworks such as TensorFlow and PyTorch. With its optimized drivers and libraries, the graphics card enables developers and researchers to fully leverage the capabilities of these frameworks. This compatibility ensures efficient utilization of the RTX A5000’s resources, empowering professionals to develop and train machine learning models with ease.
Considerations for RTX A5000:
- Power Consumption and Cooling:
It is important to note that graphics cards of this caliber typically consume a significant amount of power and generate substantial heat during operation. To ensure optimal performance and longevity, proper cooling measures and a sufficient power supply capacity must be in place. These precautions guarantee the efficient and reliable utilization of the RTX A5000 in demanding machine learning environments.
Conclusion:
The RTX A5000 stands out as a powerhouse graphics card tailored to meet the demanding needs of machine learning professionals. With its advanced features, including a high number of CUDA cores, AI hardware acceleration support, and extensive memory capacity, the RTX A5000 offers exceptional performance for processing large amounts of data and training complex models. Its seamless integration with popular machine learning frameworks further enhances its usability and versatility. While considerations such as power consumption and cooling are crucial, proper infrastructure and precautions can ensure the effective utilization of the RTX A5000’s capabilities. With the RTX A5000, professionals can unlock new possibilities in machine learning and propel their research, development, and deployment of innovative models.
NVIDIA RTX 4090
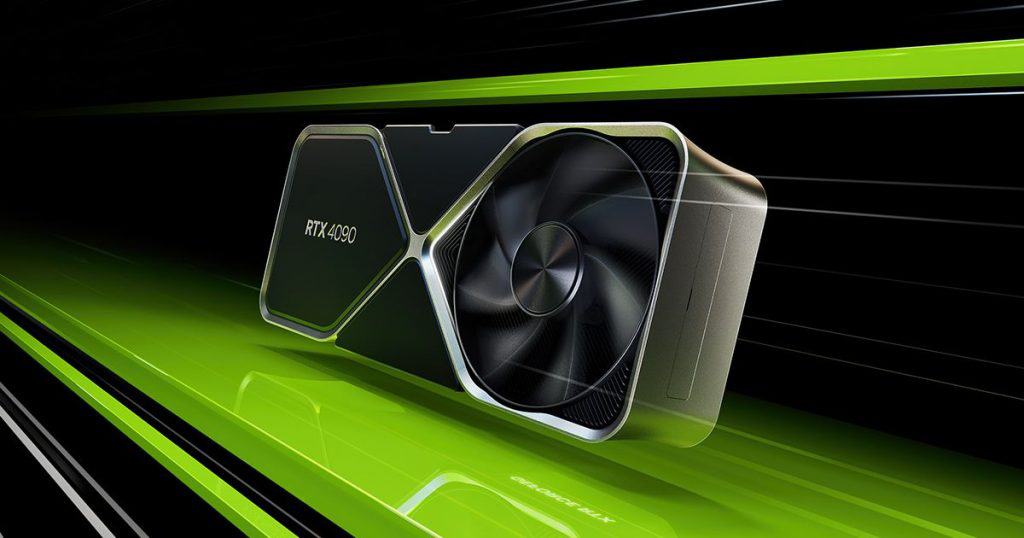
The NVIDIA RTX 4090 graphics card emerges as a powerful solution tailored to meet the demands of the latest generation of neural networks. With its outstanding performance and advanced features, the RTX 4090 offers practical benefits and distinguishes itself as a reliable option for professionals in the field. In this article, we will explore the key features of the RTX 4090 and its potential impact on accelerating machine learning models.
Pros of the NVIDIA RTX 4090:
- Outstanding Performance:
Equipped with cutting-edge technology, the NVIDIA RTX 4090 delivers exceptional performance that enables efficient handling of complex calculations and large datasets. The graphics card leverages its powerful architecture to accelerate the training of machine learning models, facilitating faster and more accurate results. The RTX 4090’s high-performance capabilities empower professionals to tackle challenging tasks and achieve enhanced productivity in their neural network projects.
Considerations for the NVIDIA RTX 4090:
- Cooling Challenges:
The intense heat generation of the NVIDIA RTX 4090 can present challenges in terms of cooling. Due to the high-performance nature of the card, it is essential to ensure adequate cooling measures are in place to prevent overheating. Users should be aware that in multi-card configurations, the heat dissipation requirements become even more critical to maintain optimal performance and prevent automatic shutdowns triggered by reaching critical temperatures. - Configuration Limitations:
The GPU design of the NVIDIA RTX 4090 imposes certain limitations on the number of cards that can be installed in a workstation. This restriction may affect users who require multiple RTX 4090 cards for their projects. Careful consideration of the configuration and compatibility of the workstation is necessary to ensure optimal utilization of the RTX 4090’s capabilities.
Conclusion:
The NVIDIA RTX 4090 graphics card stands as a powerful choice for professionals seeking to power the latest generation of neural networks. With its outstanding performance and efficient handling of complex calculations and large datasets, the RTX 4090 accelerates the training of machine learning models, opening new possibilities for researchers and developers in the field. However, users should be mindful of the cooling challenges associated with the intense heat generated by the card, especially in multi-card configurations. Additionally, the configuration limitations should be taken into account when considering the installation of multiple RTX 4090 cards in a workstation. By harnessing the capabilities of the NVIDIA RTX 4090 and addressing these considerations, professionals can optimize their neural network projects and unlock new frontiers in machine learning advancements.
NVIDIA RTX 4080
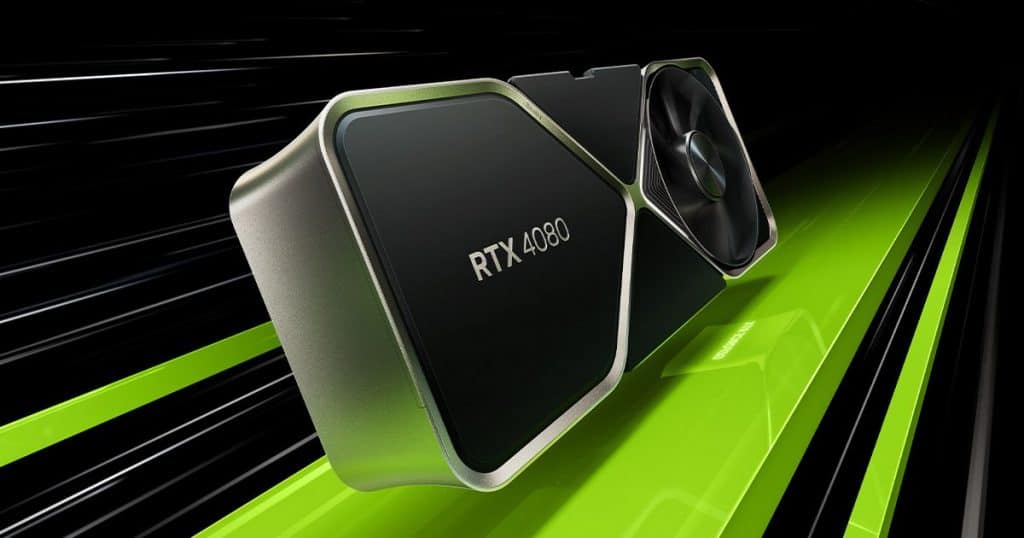
The RTX 4080 graphics card has emerged as a powerful and efficient solution in the field of artificial intelligence. With its high performance and reasonable price point, the RTX 4080 presents an appealing choice for developers aiming to maximize their system’s potential. In this article, we will delve into the distinguishing features and practical benefits of the RTX 4080, exploring its impact on accelerating machine learning tasks.
Pros of the RTX 4080:
- High Performance:
The RTX 4080 boasts an impressive 9728 NVIDIA CUDA cores, enabling it to deliver exceptional computing power for machine learning tasks. This high-performance capability, combined with the presence of tensor cores and support for ray tracing, contributes to more efficient data processing and enhanced accuracy in AI-related operations. Developers can leverage the RTX 4080’s power to handle complex calculations and large datasets, optimizing their machine learning workflows. - Competitive Pricing:
With a price point of $1,199, the RTX 4080 offers an attractive proposition for individuals and small teams seeking a productive machine learning solution. Its combination of affordability and high performance makes it an accessible option for developers looking to harness the benefits of AI without breaking the bank.
Considerations for the RTX 4080:
- SLI Limitation:
It is important to note that the RTX 4080 does not support NVIDIA NVLink with SLI function. This limitation implies that users cannot combine multiple RTX 4080 cards in SLI mode to further enhance performance. While this may restrict the scalability of the graphics card in certain setups, it does not diminish its standalone capabilities in delivering efficient AI processing.
Conclusion:
The RTX 4080 graphics card stands as a compelling choice for developers seeking to unlock high-performance AI capabilities. With its robust specifications, including 9728 NVIDIA CUDA cores, tensor cores, and ray tracing support, the RTX 4080 offers a practical solution for accelerating machine learning tasks. Moreover, its competitive price of $1,199 makes it an accessible option for individuals and small teams, allowing them to harness the power of AI without a significant financial burden. While the absence of SLI support restricts multi-card configurations, it does not detract from the standalone performance and efficiency of the RTX 4080. By embracing the advantages of the RTX 4080, developers can elevate their machine learning projects and achieve new breakthroughs in artificial intelligence advancements.
NVIDIA RTX 4070
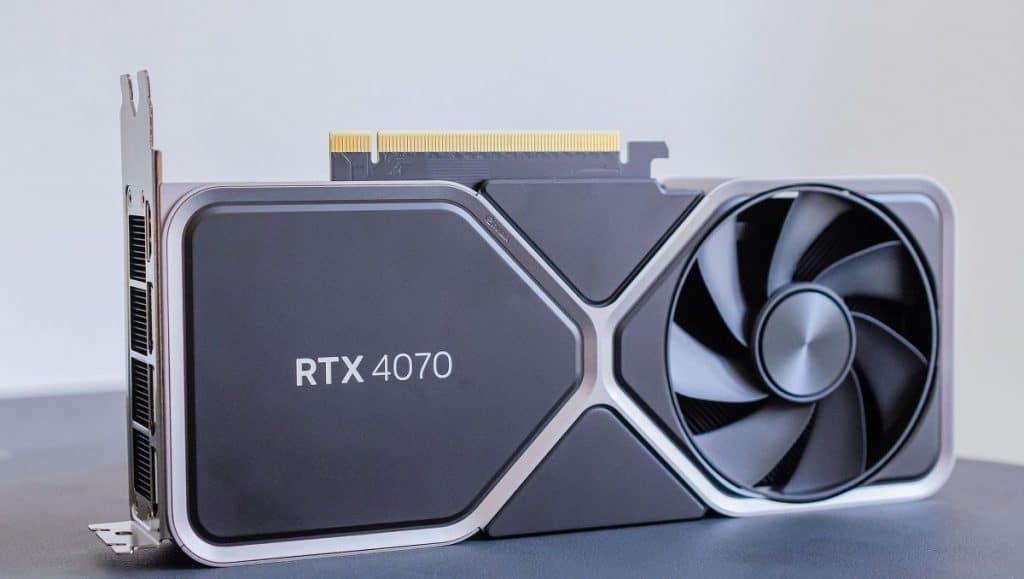
The NVIDIA RTX 4070 graphics card, built on the innovative Ada Lovelace architecture, has been making waves in the realm of machine learning. With its 12GB memory capacity, this graphics card offers accelerated data access and enhanced training speeds for machine learning models. In this article, we will delve into the practical benefits and distinguishing features of the NVIDIA RTX 4070, highlighting its suitability for individuals entering the field of machine learning.
Pros of the NVIDIA RTX 4070:
- High Performance:
The NVIDIA RTX 4070 combines the power of 7680 CUDA cores and 184 tensor cores, providing users with a commendable processing capability for complex operations. The 12GB memory capacity enables efficient handling of large datasets, allowing for seamless workflows in machine learning tasks. - Low Power Consumption:
Operating at a mere 200W, the NVIDIA RTX 4070 graphics card exhibits exceptional energy efficiency. Its low power consumption ensures that users can enjoy powerful machine learning performance without placing excessive strain on their systems or incurring exorbitant energy costs. - Cost-Effective Solution:
With a price point of $599, the NVIDIA RTX 4070 graphics card presents an affordable option for individuals seeking to explore and learn machine learning. Despite its attractive price, the card does not compromise on performance, making it an excellent choice for those on a budget.
Considerations for the NVIDIA RTX 4070:
- Limited Memory Capacity:
While the NVIDIA RTX 4070’s 12GB memory capacity suffices for many machine learning applications, it is important to recognize that it may pose limitations when working with exceptionally large datasets. Users should assess their specific requirements and determine whether the 12GB memory capacity aligns with their needs. - Absence of NVIDIA NVLink and SLI Support:
The NVIDIA RTX 4070 graphics card does not support NVIDIA NVLink technology, which allows for the linking of multiple cards in a parallel processing system. As a result, scalability and performance may be limited in multi-card configurations. Users considering such setups should explore alternative options that cater to their specific requirements.
Conclusion:
The NVIDIA RTX 4070 graphics card emerges as an efficient and cost-effective solution for individuals venturing into the realm of machine learning. With its Ada Lovelace architecture, 12GB memory capacity, and substantial processing power, it delivers an impressive performance that empowers users to tackle complex machine learning operations. Furthermore, the card’s low power consumption of 200W ensures energy-efficient usage, mitigating strain on systems and reducing energy costs. Priced at $599, the NVIDIA RTX 4070 offers an accessible entry point for individuals seeking to delve into machine learning without breaking the bank.
NVIDIA GeForce RTX 3090 TI
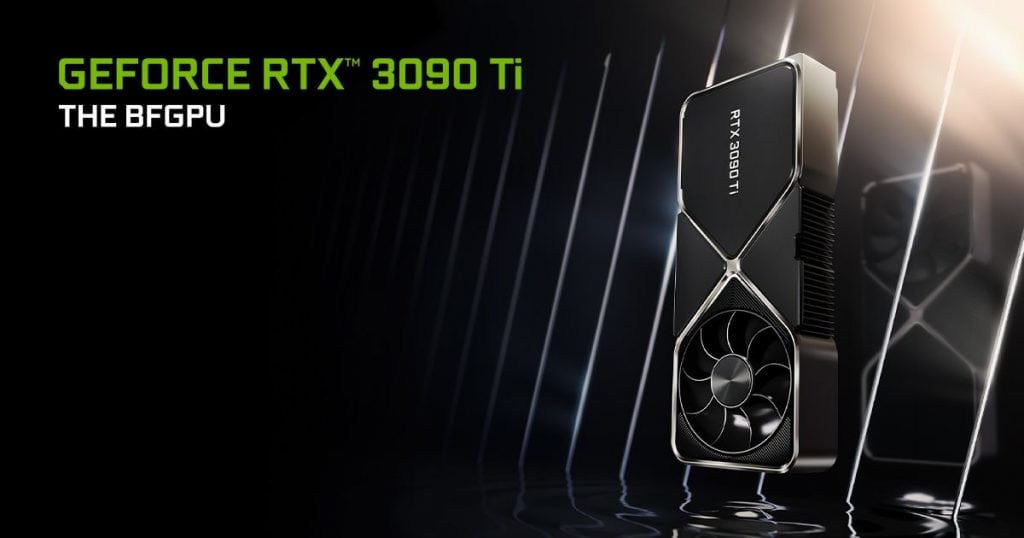
The NVIDIA GeForce RTX 3090 TI has garnered attention as a gaming GPU that also boasts impressive capabilities for deep learning tasks. With its peak single precision (FP32) performance of 13 teraflops, 24GB of VRAM, and 10,752 CUDA cores, this graphics card offers exceptional performance and versatility. In this article, we will explore the practical benefits and distinguishing features of the NVIDIA GeForce RTX 3090 TI, highlighting its suitability for both gaming enthusiasts and deep learning practitioners.
Pros of the NVIDIA GeForce RTX 3090 TI:
- High Performance:
Equipped with the Ampere architecture and an impressive 10,752 CUDA cores, the NVIDIA GeForce RTX 3090 TI delivers exceptional performance. This enables users to tackle complex machine learning problems with ease, allowing for faster and more efficient computations. - Hardware Learning Acceleration:
The RTX 3090 TI supports Tensor Cores technology, which facilitates hardware accelerated neural network operations. By leveraging Tensor Cores, users can experience significant speed improvements in the training of deep learning models. This advancement contributes to enhanced productivity and shorter model training times. - Large Memory Capacity:
With 24GB of GDDR6X memory, the NVIDIA GeForce RTX 3090 TI offers ample storage space to handle large amounts of memory data. This capacity minimizes the need for frequent disk reads and writes, resulting in smoother workflows and improved efficiency, particularly when working with extensive datasets.
Considerations for the NVIDIA GeForce RTX 3090 TI:
- Power Consumption:
The NVIDIA GeForce RTX 3090 TI demands a substantial amount of power, with a power consumption rating of 450W. As a result, it is crucial to ensure a robust power supply to support the card’s operation. The high power consumption may lead to increased energy costs and limit the card’s compatibility with certain systems, particularly when deploying multiple cards in parallel computing setups. - Compatibility and Support:
While the NVIDIA GeForce RTX 3090 TI is a powerful graphics card, there may be compatibility and support considerations with certain software platforms and machine learning libraries. Users should verify compatibility and be prepared to make necessary adjustments or updates to fully utilize the card’s capabilities within their specific software environments.
Conclusion:
The NVIDIA GeForce RTX 3090 TI stands as an impressive gaming GPU that also excels in deep learning applications. With its powerful Ampere architecture, extensive CUDA core count, and hardware learning acceleration capabilities, it empowers users to tackle complex machine learning tasks efficiently. Additionally, its substantial 24GB memory capacity minimizes data transfer bottlenecks, facilitating seamless operations even with large datasets.
NVIDIA GeForce RTX 3080 TI
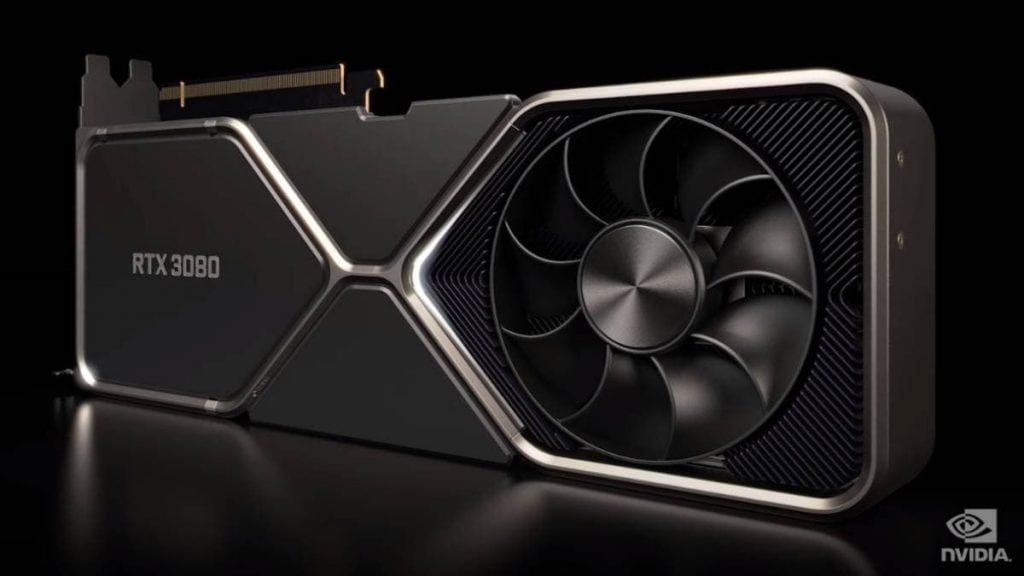
The NVIDIA GeForce RTX 3080 TI has emerged as a highly capable mid-range graphics card that offers impressive performance for machine learning tasks. With its robust specifications, including the Ampere architecture, 8704 CUDA cores, and 12GB of GDDR6X memory, this card delivers substantial processing power. In this article, we will delve into the practical benefits and distinguishing features of the NVIDIA GeForce RTX 3080 TI, highlighting its value proposition for users seeking high performance without breaking the bank.
Pros of the NVIDIA GeForce RTX 3080 TI:
- Powerful Performance:
Equipped with the Ampere architecture and boasting 8704 CUDA cores, the NVIDIA GeForce RTX 3080 TI delivers exceptional processing power. This enables users to handle demanding machine learning tasks with ease, accelerating computations and reducing training times. - Hardware Learning Acceleration:
The inclusion of Tensor Cores in the graphics card enables hardware accelerated neural network operations. Leveraging Tensor Cores translates into significant acceleration when performing neural network tasks, resulting in faster training of deep learning models. This advancement enhances productivity and facilitates quicker model iterations. - Relatively Affordable Price:
With a price tag of $1499, the NVIDIA GeForce RTX 3080 TI offers a relatively affordable option for users seeking powerful machine learning capabilities. This mid-range card provides an optimal balance between performance and cost, making it an attractive choice for budget-conscious individuals or small teams. - Ray Tracing and DLSS Support:
The RTX 3080 TI supports hardware-accelerated Ray Tracing and Deep Learning Super Sampling (DLSS) technologies. These features enhance the visual quality of graphics, enabling users to experience more realistic and immersive environments. Ray Tracing and DLSS capabilities are valuable assets when visualizing model outputs and rendering high-quality graphics.
Considerations for the NVIDIA GeForce RTX 3080 TI:
- Limited Memory:
While the RTX 3080 TI offers 12GB of GDDR6X memory, it is important to note that this may restrict the ability to handle large amounts of data or complex models that require extensive memory resources. Users should assess their specific requirements and determine whether the available memory capacity aligns with their intended use cases.
Conclusion:
The NVIDIA GeForce RTX 3080 TI presents itself as a powerful mid-range graphics card that delivers remarkable performance for machine learning tasks. Powered by the Ampere architecture and featuring 8704 CUDA cores, this card offers robust processing power to handle demanding computations efficiently. With support for hardware learning acceleration through Tensor Cores, users can benefit from accelerated neural network operations and faster model training.
Wrap It Up
In the realm of machine learning, selecting the right graphics card is pivotal for maximizing data processing capabilities and enabling efficient parallel computing. By considering key factors such as computing power, GPU memory capacity, support for specialized libraries, high-performance support, and compatibility with machine learning frameworks, practitioners can ensure they have the necessary hardware to tackle complex machine learning tasks. While NVIDIA GPUs dominate the machine learning landscape, it is essential to evaluate the specific requirements of the project and choose the graphics card that best aligns with those needs. With the right graphics card, researchers and practitioners can unleash the full potential of their machine learning endeavors.
NVIDIA: The Leading Player in Machine Learning GPUs
Currently, NVIDIA stands at the forefront of machine learning GPUs, providing optimized drivers and extensive support for CUDA and cuDNN. NVIDIA GPUs offer remarkable computational acceleration, enabling researchers and practitioners to expedite their work significantly.
AMD: Gaming Focused, Limited Machine Learning Adoption
Although AMD GPUs have established themselves as formidable contenders in the gaming industry, their adoption for machine learning remains relatively limited. This can be attributed to factors such as limited software support and the necessity for frequent updates to meet the demands of evolving machine learning frameworks.
FAQs
The selection of the appropriate graphics card is crucial as it determines the capability to handle intensive matrix and tensor processing required for tasks like deep neural network training.
Specialized AI chips such as TPUs (Tensor Processing Units) and FPGAs (Field Programmable Gate Arrays) have gained considerable popularity in recent times.
Tasks involving deep neural network training require intensive processing of matrices and tensors.
Specialized AI chips offer improved performance and efficiency for tasks related to artificial intelligence, making them highly desirable for processing large datasets and conducting parallel computations.
Choosing the right graphics card with sufficient processing power and memory capacity is crucial for achieving high performance in deep neural network training tasks.
Besides graphics cards, specialized AI chips such as TPUs and FPGAs have gained considerable popularity for their efficiency in handling AI-related tasks.
TPUs and FPGAs have gained popularity due to their ability to provide specialized hardware acceleration for AI-related tasks, enabling faster and more efficient processing of large datasets and complex computations.
Specialized AI chips like TPUs and FPGAs often outperform traditional graphics cards in terms of performance and efficiency for AI tasks, as they are designed specifically for these workloads.
There are several graphics cards that are highly regarded for machine learning (ML) and artificial intelligence (AI) tasks. Here are some of the best graphics cards for ML and AI:
- NVIDIA A100: Built on the Ampere architecture, the A100 is a powerhouse for AI and ML tasks. It boasts a massive number of CUDA cores and supports advanced AI technologies.
- NVIDIA GeForce RTX 3090: This high-end graphics card offers exceptional performance with its powerful GPU, large memory capacity, and support for AI acceleration technologies like Tensor Cores.
- NVIDIA Quadro RTX 8000: This professional-grade graphics card is designed for demanding ML and AI applications, with its high computing power and extensive memory capacity.
Disclaimer
In line with the Trust Project guidelines, please note that the information provided on this page is not intended to be and should not be interpreted as legal, tax, investment, financial, or any other form of advice. It is important to only invest what you can afford to lose and to seek independent financial advice if you have any doubts. For further information, we suggest referring to the terms and conditions as well as the help and support pages provided by the issuer or advertiser. MetaversePost is committed to accurate, unbiased reporting, but market conditions are subject to change without notice.
About The Author
Damir is the team leader, product manager, and editor at Metaverse Post, covering topics such as AI/ML, AGI, LLMs, Metaverse, and Web3-related fields. His articles attract a massive audience of over a million users every month. He appears to be an expert with 10 years of experience in SEO and digital marketing. Damir has been mentioned in Mashable, Wired, Cointelegraph, The New Yorker, Inside.com, Entrepreneur, BeInCrypto, and other publications. He travels between the UAE, Turkey, Russia, and the CIS as a digital nomad. Damir earned a bachelor's degree in physics, which he believes has given him the critical thinking skills needed to be successful in the ever-changing landscape of the internet.
More articles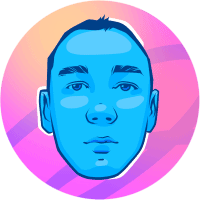
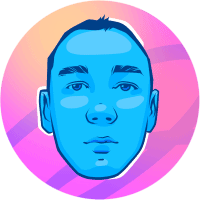
Damir is the team leader, product manager, and editor at Metaverse Post, covering topics such as AI/ML, AGI, LLMs, Metaverse, and Web3-related fields. His articles attract a massive audience of over a million users every month. He appears to be an expert with 10 years of experience in SEO and digital marketing. Damir has been mentioned in Mashable, Wired, Cointelegraph, The New Yorker, Inside.com, Entrepreneur, BeInCrypto, and other publications. He travels between the UAE, Turkey, Russia, and the CIS as a digital nomad. Damir earned a bachelor's degree in physics, which he believes has given him the critical thinking skills needed to be successful in the ever-changing landscape of the internet.