VToonify: A real-time AI model for generating artistic portrait videos
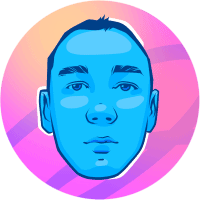
In Brief
A revolutionary VToonify framework was developed by developers to provide controlled, high-resolution portrait video style transfers.
To produce stunning artistic portraits, the framework makes use of StyleGAN’s mid- and high-res layers.
It allows the extension of existing StyleGAN-based image toonification models to video.
Researchers from Nanyang Technological University have introduced a novel VToonify framework to generate controllable high-resolution portrait video style transfer. VToonify leverages the mid- and high-resolution layers of StyleGAN to render high-quality artistic portraits based on the multi-scale content features extracted by an encoder to better preserve frame details. Experimental results show that our framework can generate videos with consistently high quality and desired facial expressions without the need for face alignment or frame-size restrictions.
As a result, a fully convolutional architecture that accepts non-aligned faces in videos of various sizes produces complete faces with organic motions. VToonify framework inherits appealing features of these models for flexible style control on color and intensity. It is compatible with existing StyleGAN-based image toonification models to extend them to video toonification. This work introduces two instantiations of VToonify for collection-based and exemplar-based portrait video style transfer, respectively, built upon Toonify and DualStyleGAN.
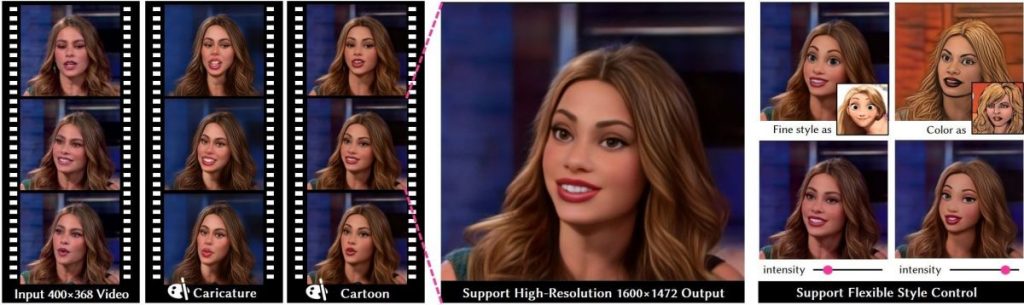
Extensive experimental findings show that proposed VToonify framework outperforms competing approaches in producing artistic portrait films with adjustable style controls that are of excellent quality and temporally consistent. Check GitHub for more details.
Related article: OpenAI is working on creating an AI model for video |
In order to provide a controllable high-resolution portrait video style transfer, VToonify combines the advantages of the image translation framework and the StyleGAN-based framework.
(A) To support variable input size, an image translation system uses fully convolutional networks. It is challenging to impart high-resolution and controlled style, nevertheless, when teaching from scratch.
(B) StyleGAN-based framework, which only supports fixed picture size and detail losses, uses the pre-trained StyleGAN model for high-resolution and controllable style transfer.
(C) In order to create a completely convolutional encoder-generator architecture resembling that of the image translation framework, our hybrid system extends StyleGAN by deleting its fixed-sized input feature and low-resolution layers.
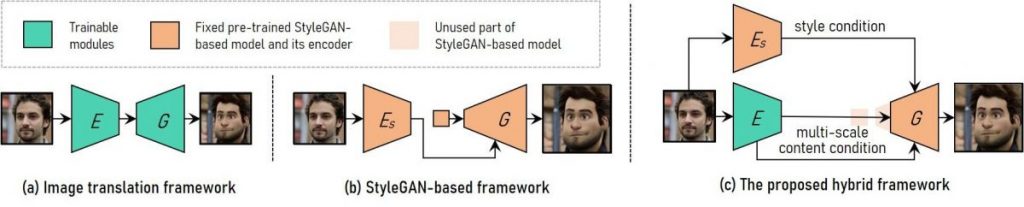
In order to preserve frame details, developers train an encoder to extract multi-scale content features from the input frame as an additional content condition. VToonify inherits the StyleGAN model’s style control flexibility by putting it into the generator to distill both its data and model.
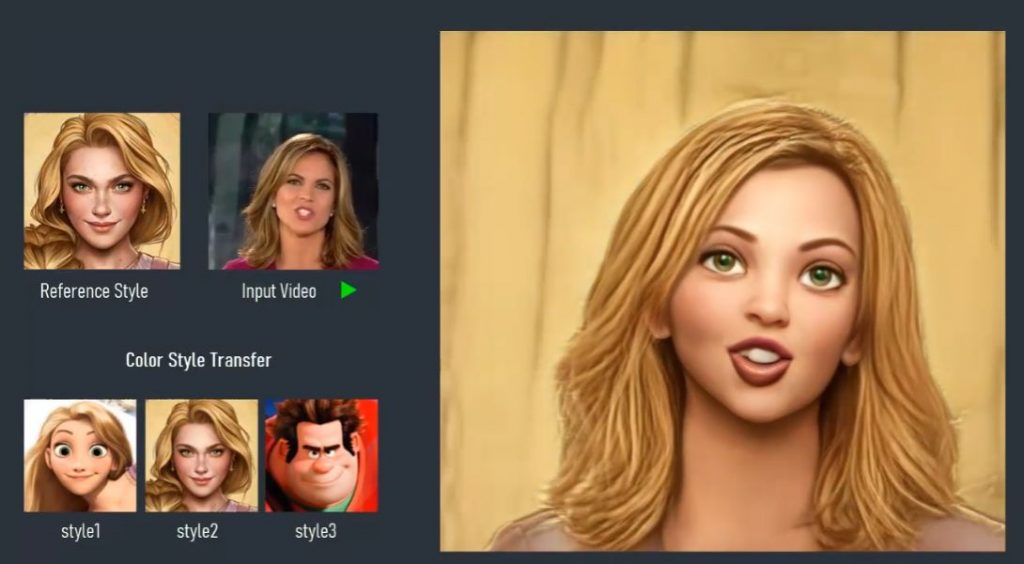
VToonify framework inherits the appealing characteristics for flexible style control from the current StyleGAN-based image toonification models and is compatible with them to expand them to video toonification. Our VToonify offers the following using the DualStyleGAN model as the StyleGAN foundation:
- Transfer of style from exemplar-based structures;
- Modification of style degree;
- Transfer of color style based on exemplars.
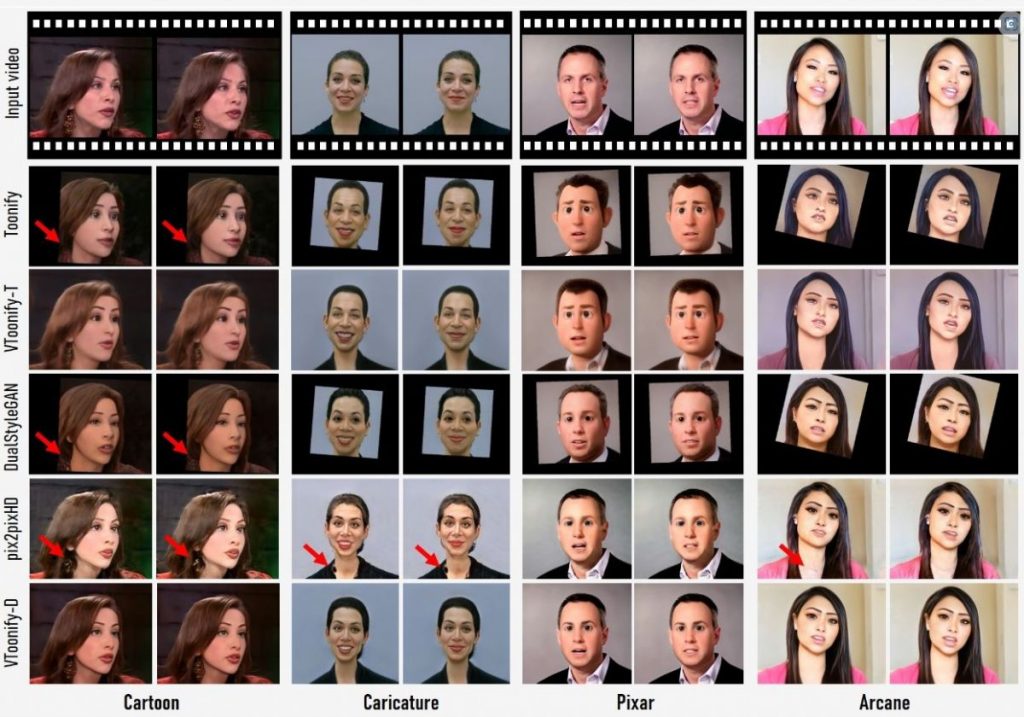
Read more about AI:
Disclaimer
In line with the Trust Project guidelines, please note that the information provided on this page is not intended to be and should not be interpreted as legal, tax, investment, financial, or any other form of advice. It is important to only invest what you can afford to lose and to seek independent financial advice if you have any doubts. For further information, we suggest referring to the terms and conditions as well as the help and support pages provided by the issuer or advertiser. MetaversePost is committed to accurate, unbiased reporting, but market conditions are subject to change without notice.
About The Author
Damir is the team leader, product manager, and editor at Metaverse Post, covering topics such as AI/ML, AGI, LLMs, Metaverse, and Web3-related fields. His articles attract a massive audience of over a million users every month. He appears to be an expert with 10 years of experience in SEO and digital marketing. Damir has been mentioned in Mashable, Wired, Cointelegraph, The New Yorker, Inside.com, Entrepreneur, BeInCrypto, and other publications. He travels between the UAE, Turkey, Russia, and the CIS as a digital nomad. Damir earned a bachelor's degree in physics, which he believes has given him the critical thinking skills needed to be successful in the ever-changing landscape of the internet.
More articles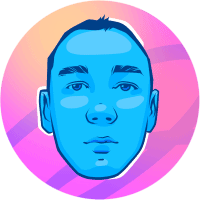
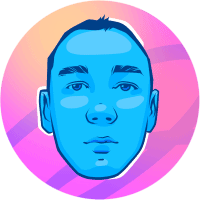
Damir is the team leader, product manager, and editor at Metaverse Post, covering topics such as AI/ML, AGI, LLMs, Metaverse, and Web3-related fields. His articles attract a massive audience of over a million users every month. He appears to be an expert with 10 years of experience in SEO and digital marketing. Damir has been mentioned in Mashable, Wired, Cointelegraph, The New Yorker, Inside.com, Entrepreneur, BeInCrypto, and other publications. He travels between the UAE, Turkey, Russia, and the CIS as a digital nomad. Damir earned a bachelor's degree in physics, which he believes has given him the critical thinking skills needed to be successful in the ever-changing landscape of the internet.