Nvidia announced eDiff-I: new generative AI for text and image synthesis with instant transfer of styles
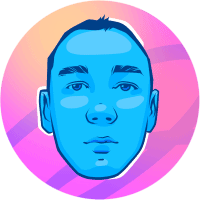
In Brief
Nvidia launches eDiff-I to help businesses create high-quality, engaging images
The eDiff-I technique regularly produces greater synthesis quality than DALL-E2 and Stable diffusion
eDiff-I is a new AI content creation tool that provides unprecedented text-to-image synthesis capabilities for marketers and businesses, as recently announced by Nvidia. With eDiff-I, businesses can quickly and easily create high-quality, engaging visuals without the need for expensive equipment or professional help. eDiff-I uses natural language processing (NLP) to interpret the user’s input and generate corresponding images. The AI then analyzes the images and chooses the most suitable one based on the context. The result is a high-quality, professional-looking image that can be used for a variety of purposes, such as marketing materials, social media posts, email campaigns, and more.
eDiff-I is a next-generation generative AI content creation tool that provides unprecedented text-to-image synthesis, fast style transfer, and intuitive painting with words. As a diffusion model for creating visuals from text, eDiff-I suggests training an ensemble of expert denoising networks, each specialized for a particular noise interval, in response to the empirical finding that the behavior of diffusion models varies at different phases of sampling.
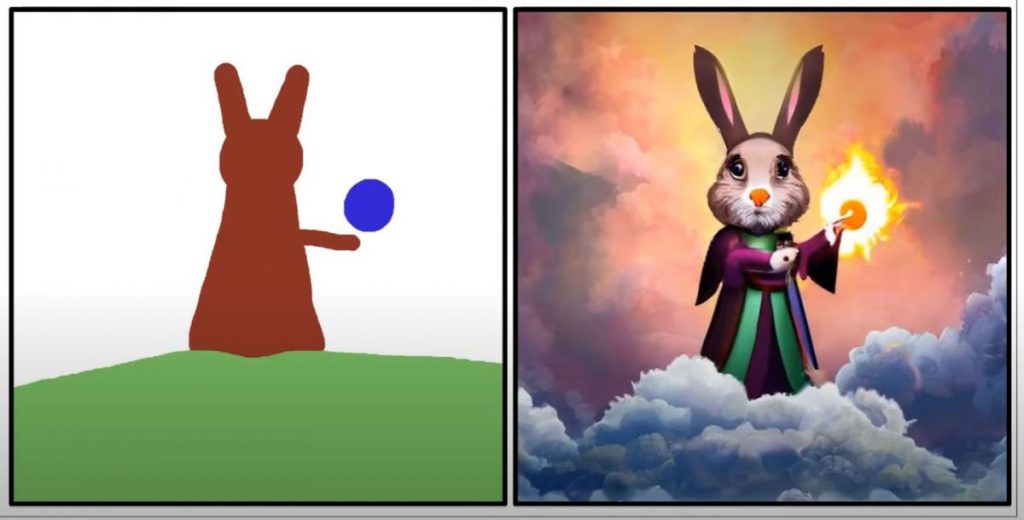
The T5 text embeddings, CLIP image embeddings, and CLIP text embeddings provide the basis for the eDiff-I concept. This methodology can produce photorealistic graphics in response to any text query.
It presents two additional capabilities in addition to text-to-image synthesis: (1) style transfer, which allows us to control the style of the generated sample using a reference style image, and (2) “Paint with Words,” a tool that allows users to create images by painting segmentation maps on canvas.
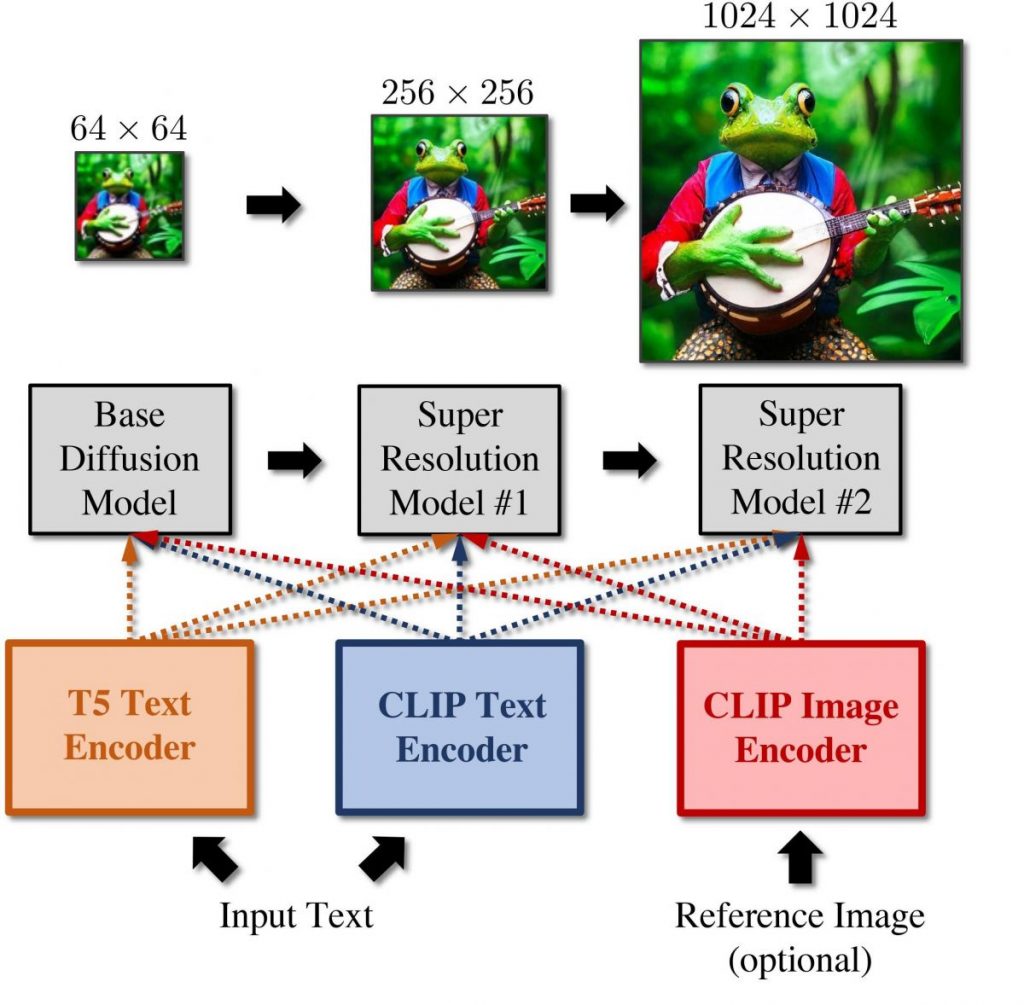
The pipeline consists of a cascade of three diffusion models: a base model that can create samples with a resolution of 64×64 and two super-resolution stacks that can gradually upsample the pictures to resolutions of 256×256 and 1024×1024, respectively. Models compute T5 XXL and text embedding after receiving a caption as input. These picture embeddings may be used as a vector of style. Then, feed these embeddings into our cascaded diffusion models, which gradually produce images with a resolution of 1024 x 1024.
eDiff-I approach consistently results in better synthesis quality when compared to the open-source text-to-image algorithms (Stable diffusion) and (DALL-E2).
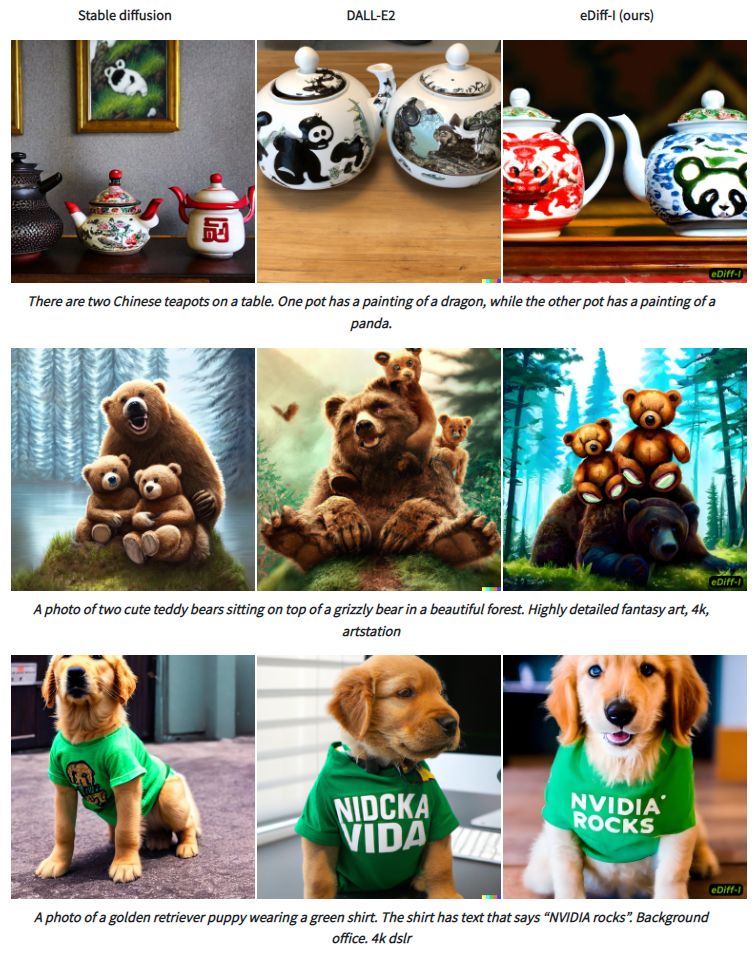
When the CLIP image embeddings are employed, the eDiff-I approach facilitates style transfer. eDiff-I first extracts the CLIP image embeddings from a reference style image, which can be utilized as a style reference vector. A stylistic reference can be seen in the figure below’s left panel. The outcomes when style conditioning is turned on are displayed in the center panel. The outcomes when style conditioning is turned off are displayed in the panel on the right. When style conditioning is applied, eDiff-I model creates outputs that are true to the input caption’s style as well. When style conditioning is turned off, natural-looking photos are produced.
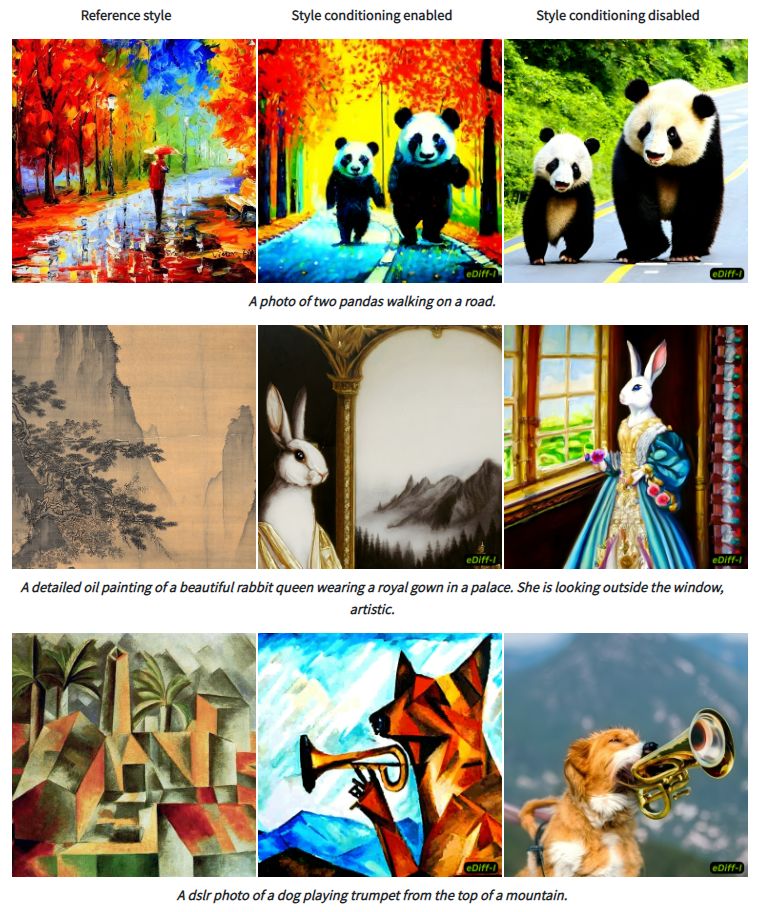
By choosing phrases and scribbling them on the image, users of the eDiff-I method can change the placement of things that are listed in the text prompt. After that, the model uses the prompt and the maps to create images that are compatible with both the caption and the input map.
Read related articles:
Disclaimer
In line with the Trust Project guidelines, please note that the information provided on this page is not intended to be and should not be interpreted as legal, tax, investment, financial, or any other form of advice. It is important to only invest what you can afford to lose and to seek independent financial advice if you have any doubts. For further information, we suggest referring to the terms and conditions as well as the help and support pages provided by the issuer or advertiser. MetaversePost is committed to accurate, unbiased reporting, but market conditions are subject to change without notice.
About The Author
Damir is the team leader, product manager, and editor at Metaverse Post, covering topics such as AI/ML, AGI, LLMs, Metaverse, and Web3-related fields. His articles attract a massive audience of over a million users every month. He appears to be an expert with 10 years of experience in SEO and digital marketing. Damir has been mentioned in Mashable, Wired, Cointelegraph, The New Yorker, Inside.com, Entrepreneur, BeInCrypto, and other publications. He travels between the UAE, Turkey, Russia, and the CIS as a digital nomad. Damir earned a bachelor's degree in physics, which he believes has given him the critical thinking skills needed to be successful in the ever-changing landscape of the internet.
More articles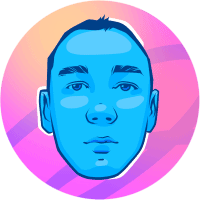
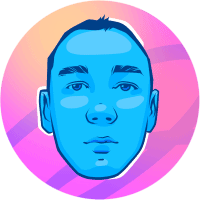
Damir is the team leader, product manager, and editor at Metaverse Post, covering topics such as AI/ML, AGI, LLMs, Metaverse, and Web3-related fields. His articles attract a massive audience of over a million users every month. He appears to be an expert with 10 years of experience in SEO and digital marketing. Damir has been mentioned in Mashable, Wired, Cointelegraph, The New Yorker, Inside.com, Entrepreneur, BeInCrypto, and other publications. He travels between the UAE, Turkey, Russia, and the CIS as a digital nomad. Damir earned a bachelor's degree in physics, which he believes has given him the critical thinking skills needed to be successful in the ever-changing landscape of the internet.