Researchers Discover a New Way to Detect AI-generated Text
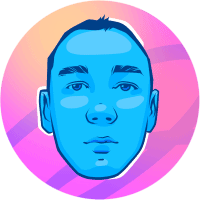
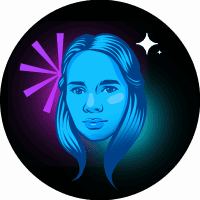
In Brief
Researchers have developed a method for detecting AI-generated text using the RoBERTa model, which extracts embeddings of text tokens and visualizes them as points in a multidimensional space.
They discovered that text generated by GPT-3.5 models, such as ChatGPT and Davinci, had significantly lower average dimensions than human-written text.
The researchers created a robust dimension-based detector that was resistant to common evasion techniques.
The detector’s accuracy remained consistently high when domains and models were changed, with a fixed threshold and a 40% accuracy drop when challenged with the DIPPER technique.
Researchers have investigated the field of AI-generated text and developed a method for detecting content generated by AI models such as GPT and Llama. They discovered interesting insights about the nature of generated text by utilizing the concept of fractional dimension. Their findings shed light on the inherent differences between text written by humans and text generated by AI models.
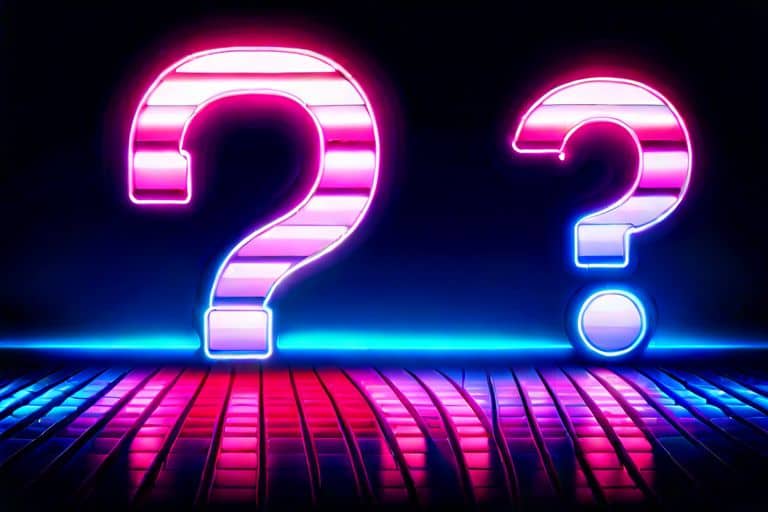
Can the dimension of a point cloud derived from natural language text provide useful information about its origin? The researchers used the RoBERTa model to extract embeddings of text tokens and visualize them as points in a multidimensional space to investigate this. They estimated the fractional dimension of these point clouds using sophisticated techniques inspired by previous works.
The researchers were astounded to discover that text generated by GPT-3.5 models, such as ChatGPT and Davinci, had significantly lower average dimensions than human-written text. This intriguing pattern persisted across domains and even when alternative models such as GPT-2 or OPT were used. Notably, even when using the DIPPER paraphrase, which is specifically designed to avoid detection, the dimension only changed by about 3%. These discoveries enabled the researchers to create a robust dimension-based detector that is resistant to common evasion techniques.
Notably, the detector’s accuracy remained consistently high when domains and models were changed. With a fixed threshold, detection accuracy (true positive rate) remained above 75% while false positive rate (FPR) remained less than 1%. Even when the detection system was challenged with the DIPPER technique, the accuracy dropped to 40%, outperforming existing detectors, including those developed by OpenAI.
Furthermore, the researchers explored the application of multilingual models like multilingual RoBERTa. This allowed them to develop similar detectors for languages other than English. While the average internal dimension of embeddings varied across different languages, the dimension of generated texts remained consistently lower than that of human-written text for each specific language.
However, the detector exhibited some weaknesses, particularly when facing high generation temperatures and primitive generator models. At higher temperatures, the internal dimension of generated texts could surpass that of human-written text, rendering the detector ineffective. Fortunately, such generator models are already detectable using alternative methods. Additionally, the researchers acknowledged that there is room for exploring alternative models for extracting text embeddings beyond RoBERTa.
Differentiating Between Human and AI-Written Text
In January, OpenAI announced the launch of a new classifier designed to distinguish between text written by humans and text generated by AI systems. This classifier aims to address the challenges posed by the increasing prevalence of AI-generated content, such as misinformation campaigns and academic dishonesty.
While detecting all AI-written text is a complex task, this classifier serves as a valuable tool to mitigate false claims of human authorship in AI-generated text. Through rigorous evaluations on a set of English texts, developers have found that that classifier accurately identifies 26% of AI-written text as “likely AI-written” (true positives), while occasionally mislabeling human-written text as AI-generated (false positives) by 9%. It’s important to note that the classifier’s reliability improves as the length of the input text increases. Compared to previous classifiers, this new version demonstrates significantly higher reliability on text generated by more recent AI systems.
To gather valuable feedback on the usefulness of imperfect tools like this classifier, developers have made it publicly available. You can try our work-in-progress classifier for free. However, it’s essential to understand its limitations. The classifier should be used as a supplementary tool, rather than a primary decision-making resource, for determining the source of a text. It exhibits high unreliability on short texts, and there are instances where human-written text may be incorrectly labeled as AI-generated.
It’s worth noting that highly predictable texts cannot be consistently identified, such as a list of the first 1,000 prime numbers. Editing AI-generated text can also help evade the classifier, and while we can update and retrain the classifier based on successful attacks, the long-term advantage of detection remains uncertain. Furthermore, classifiers based on neural networks are often poorly calibrated outside their training data, leading to extreme confidence in incorrect predictions for inputs significantly different from the training set.
Disclaimer
In line with the Trust Project guidelines, please note that the information provided on this page is not intended to be and should not be interpreted as legal, tax, investment, financial, or any other form of advice. It is important to only invest what you can afford to lose and to seek independent financial advice if you have any doubts. For further information, we suggest referring to the terms and conditions as well as the help and support pages provided by the issuer or advertiser. MetaversePost is committed to accurate, unbiased reporting, but market conditions are subject to change without notice.
About The Author
Damir is the team leader, product manager, and editor at Metaverse Post, covering topics such as AI/ML, AGI, LLMs, Metaverse, and Web3-related fields. His articles attract a massive audience of over a million users every month. He appears to be an expert with 10 years of experience in SEO and digital marketing. Damir has been mentioned in Mashable, Wired, Cointelegraph, The New Yorker, Inside.com, Entrepreneur, BeInCrypto, and other publications. He travels between the UAE, Turkey, Russia, and the CIS as a digital nomad. Damir earned a bachelor's degree in physics, which he believes has given him the critical thinking skills needed to be successful in the ever-changing landscape of the internet.
More articles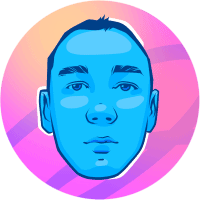
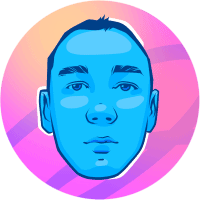
Damir is the team leader, product manager, and editor at Metaverse Post, covering topics such as AI/ML, AGI, LLMs, Metaverse, and Web3-related fields. His articles attract a massive audience of over a million users every month. He appears to be an expert with 10 years of experience in SEO and digital marketing. Damir has been mentioned in Mashable, Wired, Cointelegraph, The New Yorker, Inside.com, Entrepreneur, BeInCrypto, and other publications. He travels between the UAE, Turkey, Russia, and the CIS as a digital nomad. Damir earned a bachelor's degree in physics, which he believes has given him the critical thinking skills needed to be successful in the ever-changing landscape of the internet.