DeepMind’s AlphaZero Learns Efficient Sorting Algorithms in Neural Network Optimization
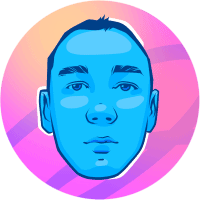
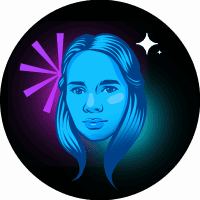
In Brief
DeepMind’s AlphaZero learns efficient sorting algorithms by playing a sorting game using reinforcement learning techniques.
DeepMind has once again made an advancement in the machine learning. Following their success in using reinforcement learning (RL) to teach AlphaZero more efficient matrix multiplication algorithms, they have now turned their attention to sorting algorithms, with promising results.
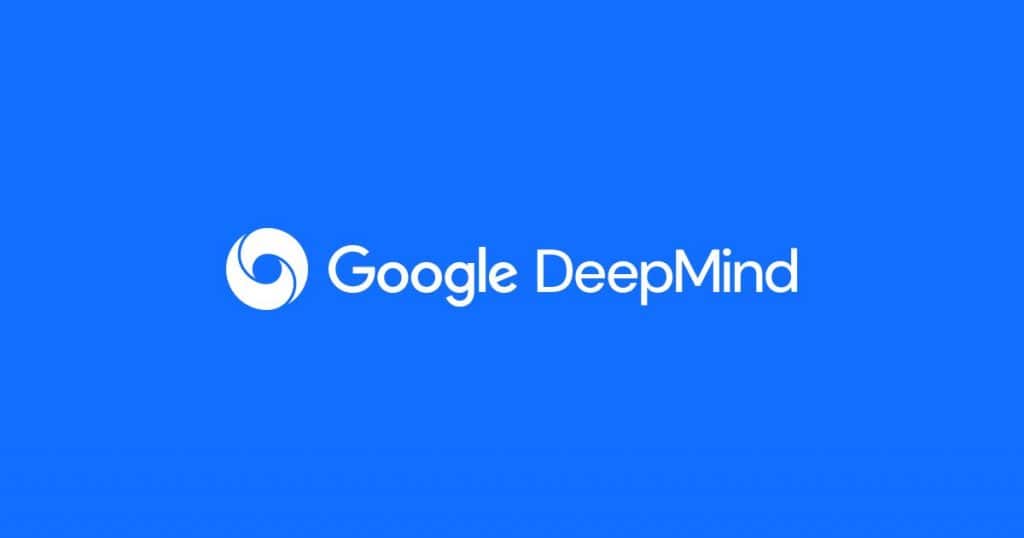
Sorting is a fundamental operation used in numerous computer programs. The efficiency of sorting arrays, performing the task in the fewest operations possible, holds great importance. Traditionally, researchers have devised various efficient sorting algorithms for different scenarios based on array sizes and data characteristics. However, DeepMind decided to explore a novel approach: teaching a neural network to solve this problem.
To accomplish this, DeepMind introduced the task of sorting an array as a game to AlphaZero. Through RL techniques, they trained AlphaZero to play this sorting game. The resulting model, known as AlphaDev, showcased remarkable capabilities in learning efficient sorting algorithms.
The sorting game operates by representing the environment’s state as a pair, where P denotes the current sorting algorithm generated in assembly language, and Z represents the state of memory and registers. At each time step, the AlphaZero agent takes the current state as input and performs an action, which involves adding a new instruction to the assembler language algorithm, such as mov.
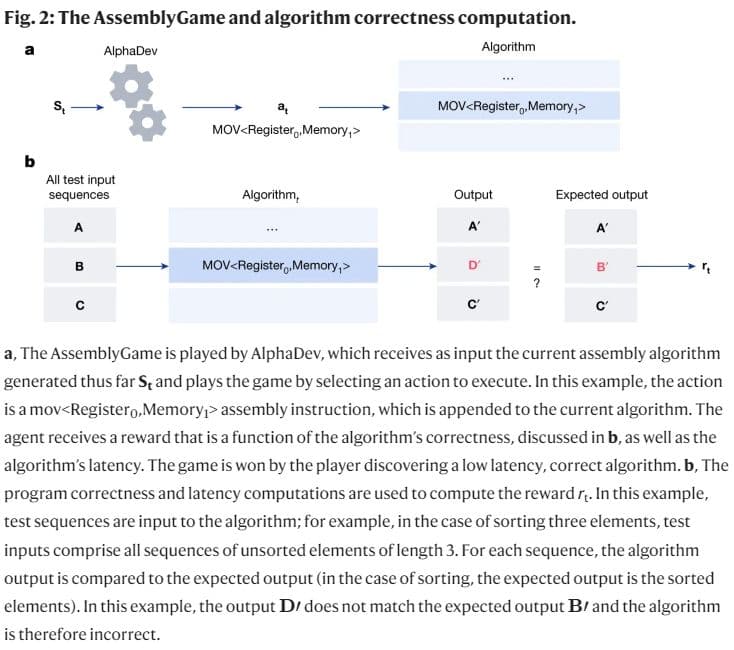
Step by step, AlphaZero constructs the final sorting algorithm in assembly language. The model’s reward at each step is determined by two factors: the correctness of the algorithm obtained and its latency. Correctness is evaluated based on predefined input-output pairs, where the input represents an unsorted array, and the output represents the same array but sorted.
The process of generating the sorting algorithm continues for a predetermined number of steps. If a final working algorithm is not obtained within this timeframe, the generation process starts anew.
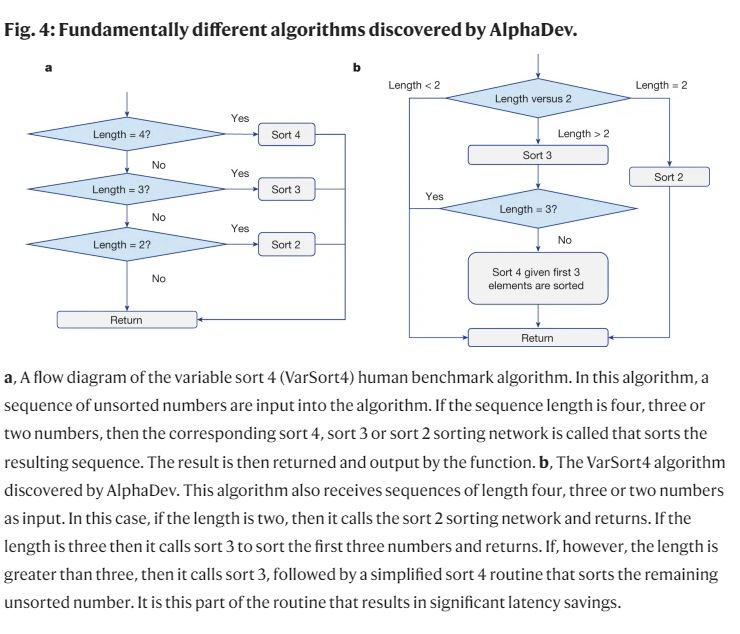
The trained model was tasked with producing sorting algorithms for sequences of length 3, 4, and 5. It’s worth noting that there are two types of sorting algorithms: fixed sort, which sorts arrays of a specific length, and variable sort, which can handle arrays of different lengths. In both cases, AlphaZero surpassed existing algorithms commonly used in programs. AlphaZero achieved improvements in sorting arrays of length 3 and 5 for fixed sort, and it discovered an intriguing approach to sort arrays of length 4 for variable sort.
For a more comprehensive understanding of the sorting algorithms discovered by AlphaDev, refer to the Nature article titled “AlphaDev: AlphaZero’s Journey into Efficient Sorting Algorithms”.
Read more about AI:
Disclaimer
In line with the Trust Project guidelines, please note that the information provided on this page is not intended to be and should not be interpreted as legal, tax, investment, financial, or any other form of advice. It is important to only invest what you can afford to lose and to seek independent financial advice if you have any doubts. For further information, we suggest referring to the terms and conditions as well as the help and support pages provided by the issuer or advertiser. MetaversePost is committed to accurate, unbiased reporting, but market conditions are subject to change without notice.
About The Author
Damir is the team leader, product manager, and editor at Metaverse Post, covering topics such as AI/ML, AGI, LLMs, Metaverse, and Web3-related fields. His articles attract a massive audience of over a million users every month. He appears to be an expert with 10 years of experience in SEO and digital marketing. Damir has been mentioned in Mashable, Wired, Cointelegraph, The New Yorker, Inside.com, Entrepreneur, BeInCrypto, and other publications. He travels between the UAE, Turkey, Russia, and the CIS as a digital nomad. Damir earned a bachelor's degree in physics, which he believes has given him the critical thinking skills needed to be successful in the ever-changing landscape of the internet.
More articles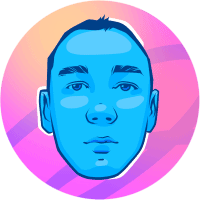
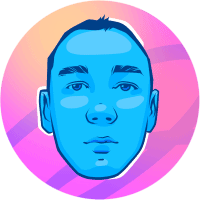
Damir is the team leader, product manager, and editor at Metaverse Post, covering topics such as AI/ML, AGI, LLMs, Metaverse, and Web3-related fields. His articles attract a massive audience of over a million users every month. He appears to be an expert with 10 years of experience in SEO and digital marketing. Damir has been mentioned in Mashable, Wired, Cointelegraph, The New Yorker, Inside.com, Entrepreneur, BeInCrypto, and other publications. He travels between the UAE, Turkey, Russia, and the CIS as a digital nomad. Damir earned a bachelor's degree in physics, which he believes has given him the critical thinking skills needed to be successful in the ever-changing landscape of the internet.