Texas Researchers Propose New Method for Reconstructing Text Based on Brain MRI Signals And AI
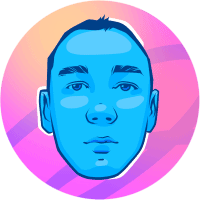
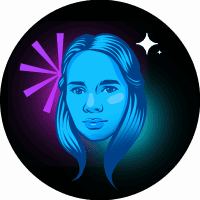
In Brief
The University of Texas has proposed a new method for reconstructing the text that a person hears based on an MRI brain signal.
The method involves training an encoder network to restore the MRI image of the brain corresponding to the text, and using pre-trained language models to generate options for continuing the text.
Statistically generated texts are closer to the original than random ones and can be used to explore the functions of different parts of the brain.
Researchers from the University of Texas have proposed a new method for recreating text from an MRI brain signal. Furthermore, decoding occurs in a coherent text semantically similar to the real one.
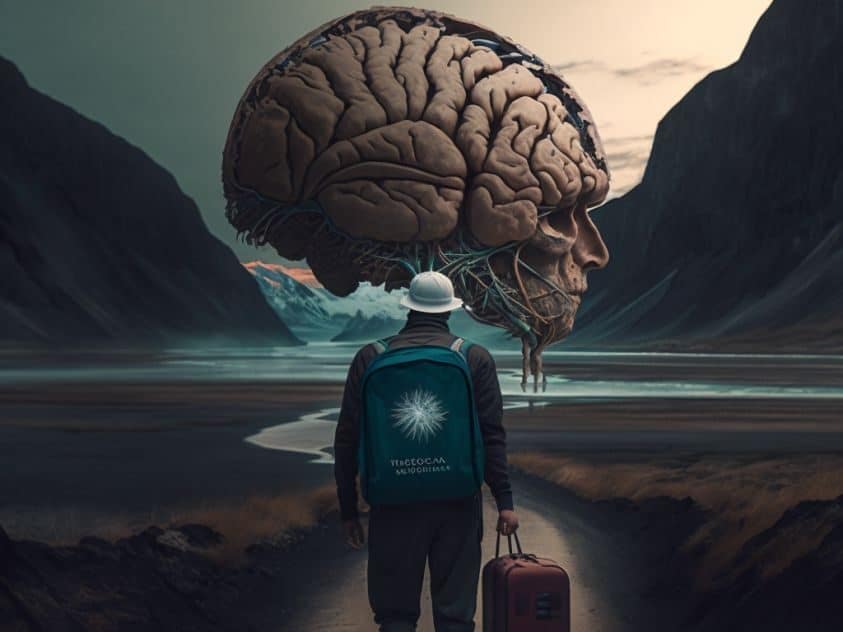
It has been attempted before to decode text that a person hears (or says in their head). Depending on how the signal is taken out of the brain, there are two different approaches. Invasion-style signal extraction is the first one: A chip that reads impulses directly from brain neurons is placed in a person’s cranium. The method is invasive, pricy, and complicated. Non-invasive signal extraction techniques, including MRI and M/EEG, are the second option; they don’t require any drilling and are less expensive.
However, non-invasive techniques for gathering brain signals have one serious flaw: a person’s MRI readings are affected by that stimulus for roughly 10 seconds after exposure to a stimulus (such as hearing a word). An English native can say two words per second on average. It turns out that each MRI image contains data about the brain processing roughly twenty words if you record an MRI signal while listening to English speakers.
As a result, using MRI, it is impossible to faithfully recreate the text that a person hears. Additionally, a lot of earlier studies on the subject of text recovery from brain signals gathered using non-invasive techniques only succeeded in retrieving specific words and phrases.
And the Texas researchers developed an MRI technique to reconstruct (nearly) intelligible text. There will be some variation between this text and what the person actually heard. However, it will be semantically equivalent, meaning that it will represent the interpretation that is usually accepted.
To recover the MRI of the brain associated with this passage of text, researchers train the encoder network, which learns from a piece of text. Then, using a pre-trained language model (such as GPT), researchers perform the following steps:
- Researchers ask GPT to create numerous possibilities for advancing the text every two seconds. The encoder network receives these many options and attempts to use them to recover the present MRI image. We think the text version that allowed for the most accurate representation of the genuine MRI signal is the accurate one.
Here is an example:
Original Input | Generation Output |
I didn’t know whether to scream, cry, or run away. Instead, I said, “Leave me alone; I don’t need your help.” Adam disappeared, and I cleaned up alone, crying. | I started to scream and cry, and then she just said, I told you to leave me alone; you can’t hurt me anymore. I’m sorry,” and then he stormed off. Though I thought he had left, I started to cry. |
This technology will have many uses if you use it to make up speech instead than listening to other people’s recordings. Even the reconstruction of fictitious speech was the subject of an experiment by the article’s authors. Once more, the final texts proved to be more similar to the originals than random ones. The approach seems to work.
And with the aid of such models, you can investigate the operations of various brain regions. In this study, three separate regions of the brain that handle audible speech were used to generate the MRI signal. One can learn which portion of the information is processed by which area of the brain by adding and eliminating signals from various parts of the brain from the model’s input. Additionally, you can contrast reconstructions of the encoder model made using signals from other components.
Read more about AI:
Disclaimer
In line with the Trust Project guidelines, please note that the information provided on this page is not intended to be and should not be interpreted as legal, tax, investment, financial, or any other form of advice. It is important to only invest what you can afford to lose and to seek independent financial advice if you have any doubts. For further information, we suggest referring to the terms and conditions as well as the help and support pages provided by the issuer or advertiser. MetaversePost is committed to accurate, unbiased reporting, but market conditions are subject to change without notice.
About The Author
Damir is the team leader, product manager, and editor at Metaverse Post, covering topics such as AI/ML, AGI, LLMs, Metaverse, and Web3-related fields. His articles attract a massive audience of over a million users every month. He appears to be an expert with 10 years of experience in SEO and digital marketing. Damir has been mentioned in Mashable, Wired, Cointelegraph, The New Yorker, Inside.com, Entrepreneur, BeInCrypto, and other publications. He travels between the UAE, Turkey, Russia, and the CIS as a digital nomad. Damir earned a bachelor's degree in physics, which he believes has given him the critical thinking skills needed to be successful in the ever-changing landscape of the internet.
More articles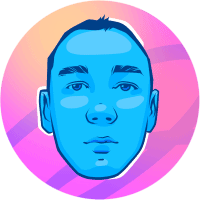
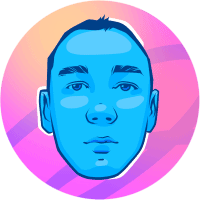
Damir is the team leader, product manager, and editor at Metaverse Post, covering topics such as AI/ML, AGI, LLMs, Metaverse, and Web3-related fields. His articles attract a massive audience of over a million users every month. He appears to be an expert with 10 years of experience in SEO and digital marketing. Damir has been mentioned in Mashable, Wired, Cointelegraph, The New Yorker, Inside.com, Entrepreneur, BeInCrypto, and other publications. He travels between the UAE, Turkey, Russia, and the CIS as a digital nomad. Damir earned a bachelor's degree in physics, which he believes has given him the critical thinking skills needed to be successful in the ever-changing landscape of the internet.