OpenAI Introduces SWE-Bench Verified To Improve Reliability Of AI Model Evaluation
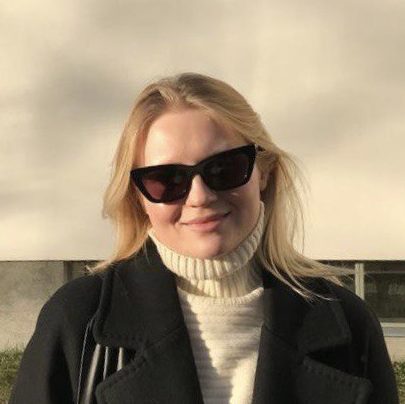
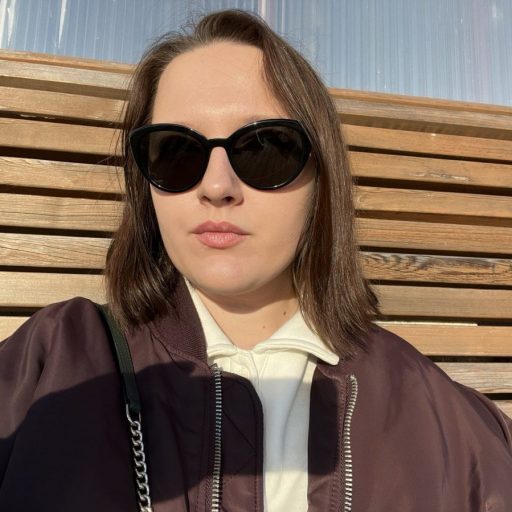
In Brief
OpenAI released human-validated subset of SWE-bench, designed to accurately assess AI models’ ability to solve real-world software problems.
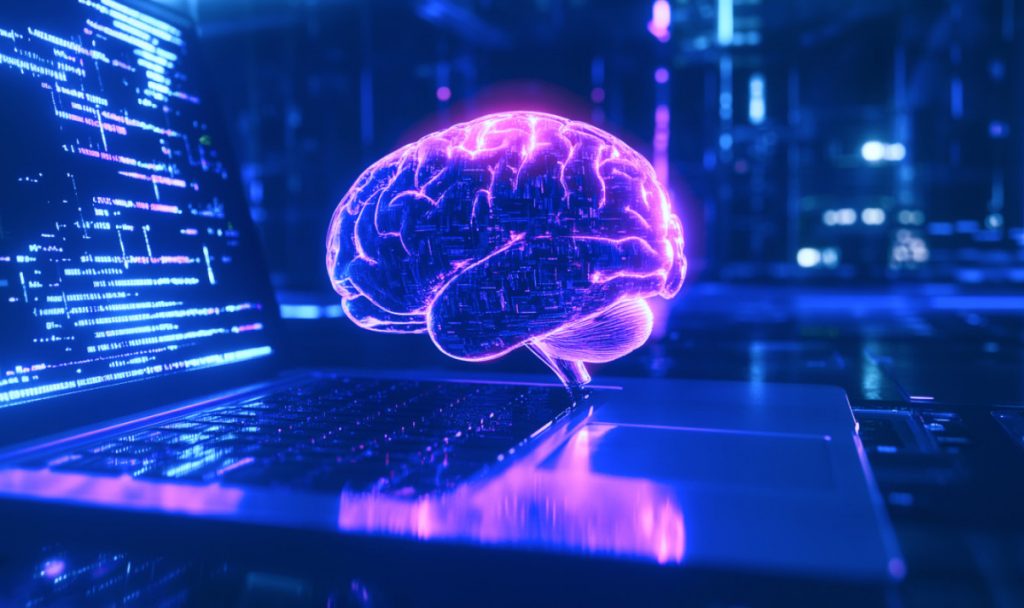
Artificial intelligence research organization OpenAI announced the release of a human-validated subset of SWE-bench, designed to more accurately assess AI models’ ability to solve real-world software problems.
SWE-bench is a benchmark used to assess large language models (LLMs) capabilities in addressing real-world software issues sourced from GitHub. It is a widely used evaluation tool for software engineering, where agents are provided with a code repository and an issue description and are tasked with creating a patch to resolve the described problem.
It is used to monitor the Medium risk level within the Model Autonomy risk category of the Preparedness Framework. Evaluating catastrophic risk levels depends on the reliability of evaluation results and a clear understanding of what the scores represent.
The company has released SWE-bench Verified in collaboration with the authors of SWE-bench. This subset of the original SWE-bench test set includes 500 samples confirmed as non-problematic by human annotators. This new version replaces both the original SWE-bench and SWE-bench Lite test sets. Additionally, it includes human annotations for all SWE-bench test samples.
Additionally, a new evaluation harness for SWE-bench has been developed. It utilizes containerized Docker environments to simplify and enhance the reliability of evaluations on SWE-bench.
Using this dataset, OpenAI evaluated GPT-4o’s performance with various open-source scaffolds. They discovered that GPT-4o achieved a score of 33.2% on SWE-bench Verified with the highest-performing scaffold, more than doubling its previous score of 16% on the original SWE-bench.
Cosine Achieves 30% Success Rate In Solving Real-World Programming Issues, GPT-4o Climbs To Second Place
The challenges in this benchmark are derived from a set of real-world programming problems known for being particularly tough for AIs. In March, startup Cognition AI reported that its model could solve 14% of these problems.
Recently, startup Cosine announced it had achieved a 30% success rate, setting a new record. Meanwhile, a model based on OpenAI‘s GPT-4o now holds the second-place position, up from third place with a previous version of the test.
Disclaimer
In line with the Trust Project guidelines, please note that the information provided on this page is not intended to be and should not be interpreted as legal, tax, investment, financial, or any other form of advice. It is important to only invest what you can afford to lose and to seek independent financial advice if you have any doubts. For further information, we suggest referring to the terms and conditions as well as the help and support pages provided by the issuer or advertiser. MetaversePost is committed to accurate, unbiased reporting, but market conditions are subject to change without notice.
About The Author
Alisa, a dedicated journalist at the MPost, specializes in cryptocurrency, zero-knowledge proofs, investments, and the expansive realm of Web3. With a keen eye for emerging trends and technologies, she delivers comprehensive coverage to inform and engage readers in the ever-evolving landscape of digital finance.
More articles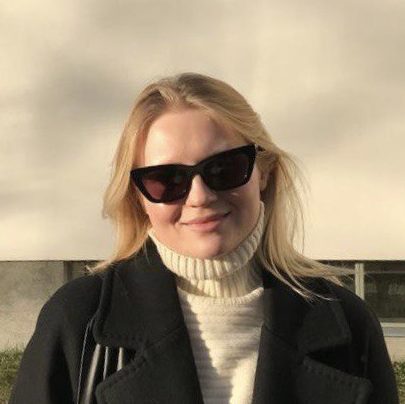
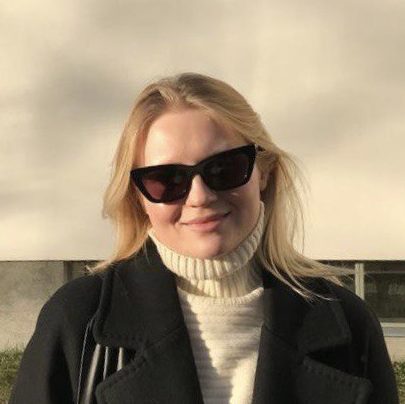
Alisa, a dedicated journalist at the MPost, specializes in cryptocurrency, zero-knowledge proofs, investments, and the expansive realm of Web3. With a keen eye for emerging trends and technologies, she delivers comprehensive coverage to inform and engage readers in the ever-evolving landscape of digital finance.