Researchers Replicated OpenAI’s Work Based on Proximal Policy Optimisation (PPO) in RLHF
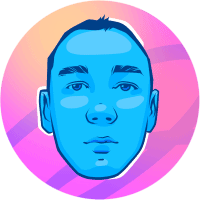
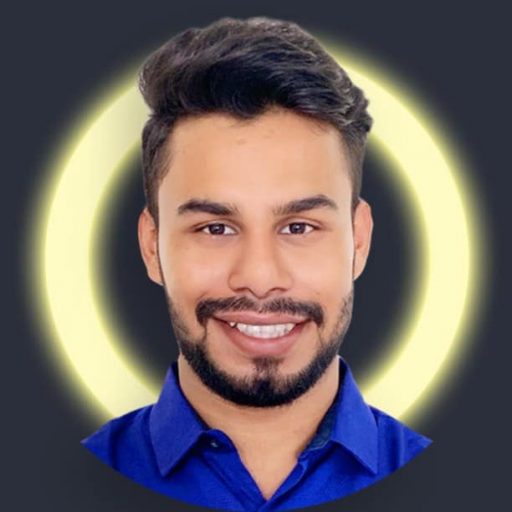
Reinforcement Learning from Human Feedback (RLHF) is an integral part of training systems like ChatGPT, and it relies on specialized methods to achieve success. One of these methods, Proximal Policy Optimization (PPO), was initially conceived within the walls of OpenAI in 2017. At first glance, PPO stood out for its promise of simplicity in implementation and a relatively low number of hyperparameters required to fine-tune the model. However, as they say, the devil is in the details.
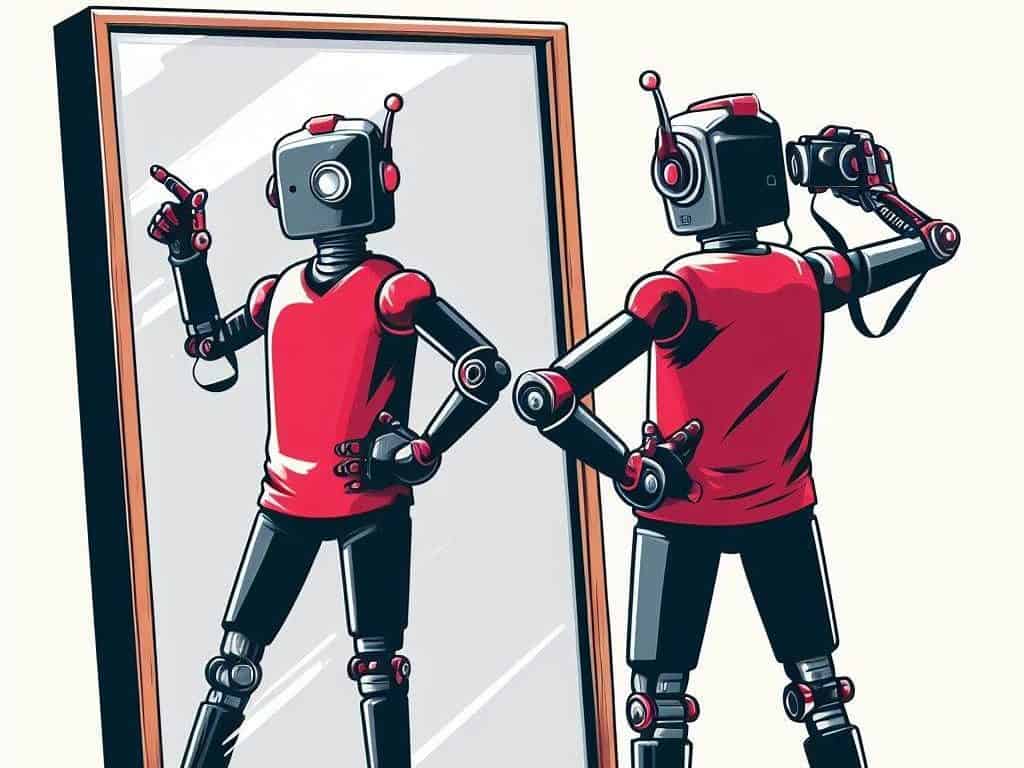
Recently, a blog post titled “The 37 Implementation Details of Proximal Policy Optimization” shed light on the intricacies of PPO (prepared for the ICLR conference). The name alone hints at the challenges faced in implementing this supposedly straightforward method. Astonishingly, it took the authors three years to gather all the necessary information and reproduce the results.
The code in the OpenAI repository underwent significant changes between versions, some aspects were left unexplained, and peculiarities that appeared as bugs somehow produced results. The complexity of PPO becomes evident when you delve into the details, and for those interested in a deep understanding or self-improvement, there’s a highly recommended video summary available.
But the story doesn’t end there. The same authors decided to revisit the openai/lm-human-preferences repository from 2019, which played a crucial role in fine-tuning language models based on human preferences, using PPO. This repository marked the early developments on ChatGPT. The recent blog post, “The N Implementation Details of RLHF with PPO,” closely replicates OpenAI’s work but uses PyTorch and modern libraries instead of the outdated TensorFlow. This transition came with its own set of challenges, such as differences in the implementation of the Adam optimizer between frameworks, making it impossible to replicate training without adjustments.
Perhaps the most intriguing aspect of this journey is the quest to run experiments on specific GPU setups to obtain original metrics and learning curves. It’s a journey filled with challenges, from memory constraints on various GPU types to the migration of OpenAI datasets between storage facilities.
In conclusion, the exploration of Proximal Policy Optimization (PPO) in Reinforcement Learning from Human Feedback (RLHF) reveals a fascinating world of complexities.
Disclaimer
In line with the Trust Project guidelines, please note that the information provided on this page is not intended to be and should not be interpreted as legal, tax, investment, financial, or any other form of advice. It is important to only invest what you can afford to lose and to seek independent financial advice if you have any doubts. For further information, we suggest referring to the terms and conditions as well as the help and support pages provided by the issuer or advertiser. MetaversePost is committed to accurate, unbiased reporting, but market conditions are subject to change without notice.
About The Author
Damir is the team leader, product manager, and editor at Metaverse Post, covering topics such as AI/ML, AGI, LLMs, Metaverse, and Web3-related fields. His articles attract a massive audience of over a million users every month. He appears to be an expert with 10 years of experience in SEO and digital marketing. Damir has been mentioned in Mashable, Wired, Cointelegraph, The New Yorker, Inside.com, Entrepreneur, BeInCrypto, and other publications. He travels between the UAE, Turkey, Russia, and the CIS as a digital nomad. Damir earned a bachelor's degree in physics, which he believes has given him the critical thinking skills needed to be successful in the ever-changing landscape of the internet.
More articles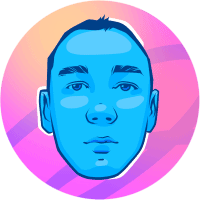
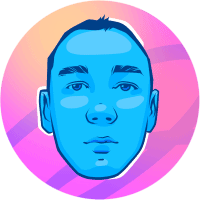
Damir is the team leader, product manager, and editor at Metaverse Post, covering topics such as AI/ML, AGI, LLMs, Metaverse, and Web3-related fields. His articles attract a massive audience of over a million users every month. He appears to be an expert with 10 years of experience in SEO and digital marketing. Damir has been mentioned in Mashable, Wired, Cointelegraph, The New Yorker, Inside.com, Entrepreneur, BeInCrypto, and other publications. He travels between the UAE, Turkey, Russia, and the CIS as a digital nomad. Damir earned a bachelor's degree in physics, which he believes has given him the critical thinking skills needed to be successful in the ever-changing landscape of the internet.