AI Training Data Scarcity Isn’t The Problem It’s Made Out To Be
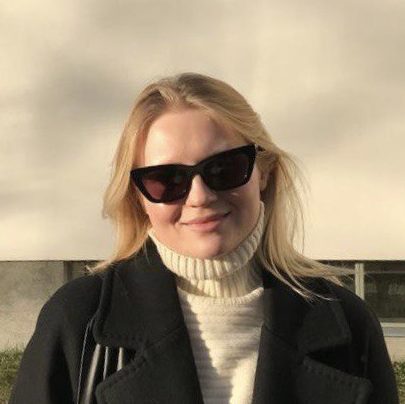
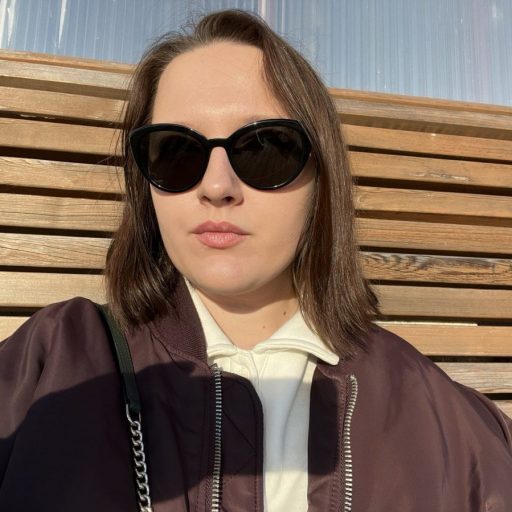
In Brief
Concerns about a shortage of data for training AI models are growing, but the public internet offers vast, constantly expanding data sources, making it unlikely that AI will ever face a true data scarcity.
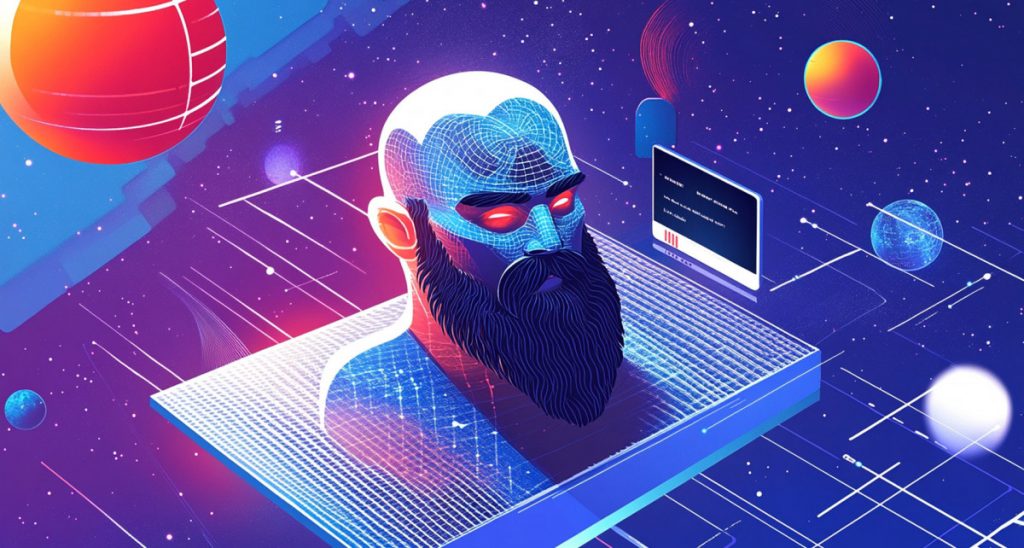
Today’s artificial intelligence models can do some amazing things. It’s almost as if they have magical powers, but of course they do not. Rather than using magic tricks, AI models actually run on data – lots and lots of data.
But there are growing concerns that a scarcity of this data might result in AI’s rapid pace of innovation running out of steam. In recent months, there have been multiple warnings from experts claiming that the world is exhausting the supply of fresh data to train the next generation of models.
A lack of data would be especially challenging for the development of large language models, which are the engines that power generative AI chatbots and image generators. They’re trained on vast amounts of data, and with each new leap in performance, more and more is required to fuel their advances.
These AI training data scarcity concerns have already caused some businesses to look for alternative solutions, such as using AI to create synthetic data for training AI, partnering with media companies to use their content, and deploying “internet of things” devices that provide real-time insights into consumer behavior.
However, there are convincing reasons to think these fears are overblown. Most likely, the AI industry will never be short of data, for developers can always fall back on the single biggest source of information the world has ever known – the public internet.
Mountains of Data
Most AI developers source their training data from the public internet already. It’s said that OpenAI’s GPT-3 model, the engine behind the viral ChatGPT chatbot that first introduced generative AI to the masses, was trained on data from Common Crawl, an archive of content sourced from across the public internet. Some 410 billion tokens’ worth or information based on virtually everything posted online up until that moment, was fed into ChatGPT, giving it the knowledge it needed to respond to almost any question we could think to ask it.
Web data is a broad term that accounts for basically everything posted online, including government reports, scientific research, news articles and social media content. It’s an amazingly rich and diverse dataset, reflecting everything from public sentiments to consumer trends, the state of the global economy and DIY instructional content.
The internet is an ideal stomping ground for AI models, not just because it’s so vast, but also because it’s so accessible. Using specialized tools such as Bright Data’s Scraping Browser, it’s possible to source information from millions of websites in real-time for their data, including many that actively try to prevent bots from doing so.
With features including Captcha solvers, automated retries, APIs, and a vast network of proxy IPs, developers can easily sidestep the most robust bot-blocking mechanisms employed on sites like eBay and Facebook, and help themselves to vast troves of information. Bright Data’s platform also integrates with data processing workflows, allowing for seamless structuring, cleaning and training at scale.
It’s not actually clear how much data is available on the internet today. In 2018, International Data Corp. estimated that the total amount of data posted online would reach 175 zettabytes by the end of 2025, while a more recent number from Statista ups that estimate to 181 zettabytes. Suffice to say, it’s a mountain of information, and it’s getting exponentially bigger over time.
Challenges and Ethical Questions
Developers still face major challenges when it comes to feeding this information into their AI models. Web data is notoriously messy and unstructured, and it often has inconsistencies and is missing values. It requires intensive processing and “cleaning” before it can be understood by algorithms. In addition, web data often contains lots of inaccurate and irrelevant details that can skew the outputs of AI models and fuel so-called “hallucinations.”
There are also ethical questions around scraping internet data, especially with regard to copyrighted materials and what constitutes “fair use.” While companies like OpenAI argue they should be allowed to scrape any and all information that’s freely available to consume online, many content creators say that doing so is far from fair, as those companies are ultimately profiting from their work – while potentially putting them out of a job.
Despite the ongoing ambiguity over what web data can and can’t be used for training AI, there’s no taking away its importance. In Bright Data’s recent State of Public Web Data Report, 88% of developers surveyed agreed that public web data is “critical” for the development of AI models, due to its accessibility and its incredible diversity.
That explains why 72% of developers are concerned that this data may become increasingly more difficult to access in the next five years, due to the efforts of Big Tech companies like Meta, Amazon and Google, which would much prefer to sell its data exclusively to high-ticket enterprise partners.
The Case for Using Web Data
The above challenges explain why there has been a lot of talk about using synthetic data as an alternative to what’s available online. In fact, there is an emerging debate regarding the benefits of synthetic data over internet scraping, with some solid arguments in favor of the former.
Advocates of synthetic data point to benefits such as the increased privacy gains, reduced biases and greater accuracy it offers. Moreover, it’s ideally structured for AI models from the get-go, meaning developers don’t have to invest resources in reformatting it and labeling it correctly for AI models to read.
On the other hand, over-reliance on synthetic data sets can lead to model collapse, and regardless, we can make an equally strong case for the superiority of public web data. For one thing, it’s hard to beat the pure diversity and richness of web-based data, which is invaluable for training AI models that need to handle the complexity and uncertainties of real-world scenarios. It can also help to create more trustworthy AI models, due to its mix of human perspectives and its freshness, especially when models can access it in real time.
In one recent interview, Bright Data’s CEO Or Lenchner stressed that the best way to ensure accuracy in AI outputs is to source data from a variety of public sources with established reliability. When an AI model only uses a single or a handful of sources, its knowledge is likely to be incomplete, he argued. “Having multiple sources provides the ability to cross-reference data and build a more balanced and well-represented dataset,” Lenchner said.
What’s more, developers have greater confidence that it’s acceptable to use data imported from the web. In a legal decision last winter, a federal judge ruled in favor of Bright Data, which had been sued by Meta over its web scraping activities. In that case, he found that while Facebook’s and Instagram’s terms of service prohibit users with an account from scraping their websites, there is no legal basis to bar logged-off users from accessing publicly-available data on those platforms.
Public data also has the advantage of being organic. In synthetic datasets, smaller cultures and the intricacies of their behavior are more likely to be omitted. On the other hand, public data generated by real world people is as authentic as it gets, and therefore translates to better-informed AI models for superior performance.
No Future Without the Web
Finally, it’s important to note that the nature of AI is changing too. As Lenchner pointed out, AI agents are playing a much greater role in AI use, helping to gather and process data to be used in AI training. The advantage of this goes beyond eliminating the burdensome manual work for developers, he said, as the speed at which AI agents operate means AI models can expand their knowledge in real-time.
“AI agents can transform industries as they allow AI systems to access and learn from constantly changing datasets on the web instead of relying on static and manually processed data,” Lenchner said. “This can lead to banking or cybersecurity AI chatbots, for example, that are capable of coming up with decisions that reflect the most recent realities.”
These days, almost everyone is accustomed to using the internet constantly. It has become a critical resource, giving us access to thousands of essential services and enabling work, communication and more. If AI systems are ever to surpass the capabilities of humans, they need access to the same resources, and the web is the most important of them all.
Disclaimer
In line with the Trust Project guidelines, please note that the information provided on this page is not intended to be and should not be interpreted as legal, tax, investment, financial, or any other form of advice. It is important to only invest what you can afford to lose and to seek independent financial advice if you have any doubts. For further information, we suggest referring to the terms and conditions as well as the help and support pages provided by the issuer or advertiser. MetaversePost is committed to accurate, unbiased reporting, but market conditions are subject to change without notice.
About The Author
Alisa, a dedicated journalist at the MPost, specializes in cryptocurrency, zero-knowledge proofs, investments, and the expansive realm of Web3. With a keen eye for emerging trends and technologies, she delivers comprehensive coverage to inform and engage readers in the ever-evolving landscape of digital finance.
More articles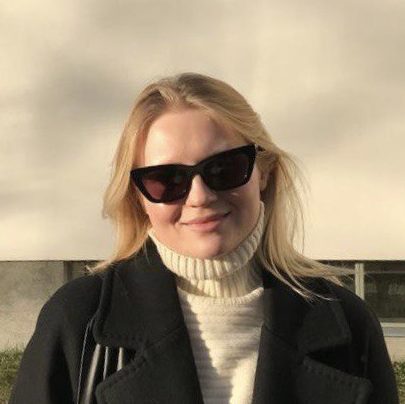
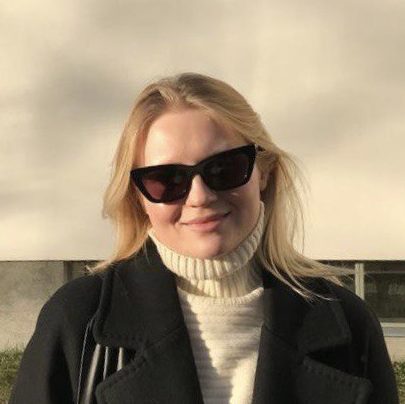
Alisa, a dedicated journalist at the MPost, specializes in cryptocurrency, zero-knowledge proofs, investments, and the expansive realm of Web3. With a keen eye for emerging trends and technologies, she delivers comprehensive coverage to inform and engage readers in the ever-evolving landscape of digital finance.