Best 10+ AI-powered Tools for Data Analysts & Data Scientists in 2023


In Brief
If you’re a data scientist/analyst looking for the perfect tool to streamline your workflow, we’ve compiled a list of 10+ AI-powered tools that you can explore.
These AI-powered data tools enable professionals to uncover hidden patterns, make accurate predictions, and generate actionable insights.
AI-powered tools have become indispensable assets for professionals seeking to extract meaningful insights from vast and complex datasets. These AI tools empower data analysts and scientists to tackle intricate challenges, automate workflows, and optimize decision-making processes.
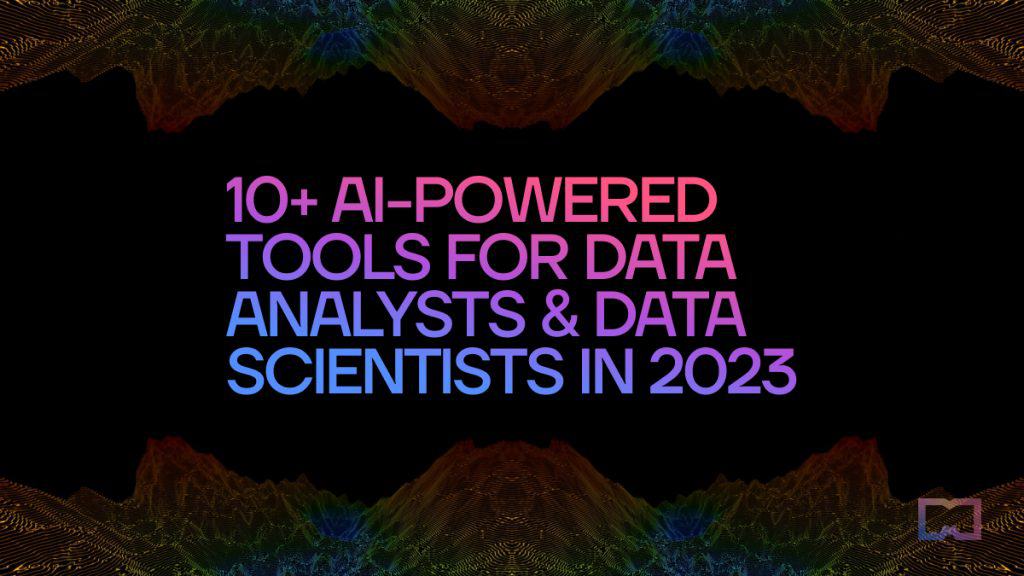
By leveraging advanced algorithms and machine learning techniques, these AI-powered data tools enable professionals to uncover hidden patterns, make accurate predictions, and generate actionable insights. These tools automate repetitive tasks, streamline data preparation and modeling processes, and empower users to extract maximum value from their datasets.
Each tool offers a unique set of features and functionalities tailored to different aspects of the data analysis process. From data extraction and cleansing to exploratory analysis and predictive modeling, these tools provide a comprehensive toolkit for end-to-end data analysis. They typically utilize intuitive interfaces, programming languages, or visual workflows to enable users to interact with data, perform complex computations, and visualize results effectively.
If you’re a data scientist/analyst looking for the perfect tool to streamline your workflow, we’ve compiled a list of 10+ AI-powered tools that you can explore.
Google Cloud AutoML
Google Cloud AutoML is a powerful AI tool that simplifies the process of building machine learning models. It streamlines the process of training machine learning models by automating repetitive tasks like hyperparameter tuning and model architecture selection.
It also provides an intuitive graphical interface, enabling data scientists to build and deploy models without extensive coding knowledge. It also seamlessly integrates with other Google Cloud tools and services.
Pros:
- Simplifies machine learning model development.
- No extensive coding skills required.
- Integrates well with the Google Cloud Platform.
Cons:
- Limited flexibility for advanced model customization.
- Pricing can be expensive for large-scale projects.
- Dependency on the Google Cloud ecosystem.
Amazon SageMaker
Amazon SageMaker is a comprehensive machine-learning platform that provides data scientists with end-to-end model development capabilities. Its scalable infrastructure handles the heavy lifting of model training and deployment, making it suitable for large-scale projects.
Sagemaker offers a wide range of built-in algorithms for various tasks, such as regression, classification, and clustering. It also enables data analysts to collaborate and share their work seamlessly, enhancing productivity and knowledge sharing within teams.
Pros:
- Scalable infrastructure for large-scale projects.
- Diverse set of built-in algorithms.
- Collaborative environment enhances teamwork.
Cons:
- Steeper learning curve for beginners.
- Advanced customization may require coding skills.
- Cost considerations for extensive usage and storage.
IBM Watson Studio
IBM Watson Studio empowers data scientists, developers, and analysts to create, deploy, and manage AI models while optimizing decision-making processes. Available on IBM Cloud Pak® for Data, the platform enables teams to collaborate seamlessly, automates AI lifecycles, and accelerates time to value through its open multicloud architecture.
With IBM Watson Studio, users can leverage a range of open-source frameworks like PyTorch, TensorFlow, and scikit-learn, alongside IBM’s own ecosystem tools for both code-based and visual data science. The platform supports popular environments such as Jupyter notebooks, JupyterLab, and command-line interfaces (CLIs), allowing users to work efficiently in languages such as Python, R, and Scala.
Pros:
- Offers a wide range of tools and capabilities for data scientists, developers, and analysts
- Facilitates collaboration and automation.
- Can be seamlessly integrated with other IBM Cloud services and tools.
Cons:
- Learning curve may be steep for beginners.
- Advanced features and enterprise-level capabilities may require a paid subscription.
- Limited flexibility for users who prefer to work with non-IBM or open-source tools and technologies.
Alteryx
Alteryx is a powerful data analytics and workflow automation tool designed to empower data analysts with a wide range of capabilities. The tool allows data analysts to easily blend and clean diverse datasets from multiple sources, enabling them to create comprehensive and reliable analytical datasets.
It also provides a variety of advanced analytics tools, including statistical analysis, predictive modeling, and spatial analytics, allowing analysts to uncover patterns, trends, and make data-driven predictions.
Pros:
- Comprehensive data blending and preparation capabilities.
- Advanced analytics tools for in-depth analysis and modeling.
- Workflow automation reduces manual effort and increases efficiency.
Cons:
- Steeper learning curve for beginners due to the complexity of the tool.
- Advanced features and customization may require additional training.
- Pricing can be expensive for smaller teams or organizations.
Altair RapidMiner
Altair RapidMiner is an enterprise-focused data science platform that enables organizations to analyze the combined influence of their employees, expertise, and data. The platform is designed to support numerous analytics users throughout the entire AI lifecycle. In September 2022, RapidMiner was acquired by Altair Engineering
It combines data preparation, machine learning, and predictive analytics in a single platform and offers a visual interface that allows data analysts to build complex data workflows through a simple drag-and-drop mechanism. The tool automates the machine learning process, including feature selection, model training, and evaluation, simplifying the analytical pipeline. There is also an extensive library of operators, enabling analysts to perform diverse data manipulation and analysis tasks.
Pros:
- Intuitive drag-and-drop interface.
- Automated machine learning streamlines the process.
- Wide variety of operators for flexible data analysis.
Cons:
- Limited customization options for advanced users.
- Steeper learning curve for complex workflows.
- Certain features may require additional licensing.
Bright Data
Bright Data allows data analysts to collect and analyze vast amounts of web data through a global proxy network. All data collection on the platform is accomplished using its AI and ML-driven algorithms.
The platform ensures high-quality data by offering comprehensive data verification and validation processes, while also ensuring compliance with data privacy regulations. With additional attributes and metadata, Bright Data enables analysts to enrich their datasets, enhancing the depth and quality of their analysis.
Pros:
- Extensive web data collection capabilities.
- High-quality and compliant data.
- Data enrichment for deeper analysis.
Cons:
- Pricing may be prohibitive for small-scale projects.
- Steep learning curve for beginners.
- Reliance on web data sources can have limitations in certain industries.
Gretel.ai
Gretel provides a platform uses machine learning techniques to generate synthetic data that closely mimics real datasets. It harnesses advanced machine learning techniques to create synthetic data that closely mirrors real-world datasets. This synthetic data exhibits similar statistical properties and patterns, enabling organizations to perform robust model training and analysis without accessing sensitive or private information.
The platform prioritizes data privacy and security by eliminating the need to work directly with sensitive data. By utilizing synthetic data, organizations can safeguard confidential information while still deriving valuable insights and developing effective machine-learning models.
Pros:
- Synthetic data generation for privacy protection.
- Privacy-enhancing techniques for secure analyses.
- Data labeling and transformation capabilities.
Cons:
- Synthetic data may not perfectly represent the complexities of real data.
- Limited to privacy-focused use cases.
- Advanced customization may require additional expertise.
MostlyAI
Founded in 2017 by three data scientists, MostlyAI leverages machine learning techniques to generate realistic and privacy-preserving synthetic data for various analytical purposes. It ensures the confidentiality of sensitive data while retaining key statistical properties, allowing analysts to work with data while complying with privacy regulations.
The platform offers shareable AI-generated synthetic data, enabling efficient collaboration and data sharing across organizations. Users can also collaborate on various types of sensitive sequential and temporal data, such as customer profiles, patient journeys, and financial transactions. MostlyAI also offers the flexibility to define specific portions of its databases for synthesis, further enhancing customization options.
Pros:
- Realistic synthetic data generation.
- Anonymization and privacy preservation capabilities.
- Data utility assessment for reliable analysis.
Cons:
- Limited to synthetic data generation use cases.
- Advanced customization may require technical expertise.
- Potential challenges in capturing complex relationships within data.
Tonic AI
Tonic AI offering AI-powered data mimicking to generate synthesized data. Synthesized data is artificially generated data that is created using algorithms. It is often used to supplement or replace real-world data, which can be expensive, time-consuming, or difficult to obtain.
The platform offers de-identification, synthesis, and subsetting, allowing users to mix and match these methods according to their specific data needs. This versatility ensures that their data is handled appropriately and securely across various scenarios. Furthermore, Tonic AI’s subsetting functionality allows users to extract specific subsets of their data for targeted analysis, ensuring that only the necessary information is used while minimizing risk.
Pros:
- Effective data anonymization techniques.
- Rule-based transformations for compliance.
- Collaboration and version control capabilities.
Cons:
- Limited to data anonymization and transformation tasks.
- Advanced customization may require coding skills.
- Certain features may require additional licensing.
KNIME
KNIME, also known as the Konstanz Information Miner, is a robust data analytics, reporting, and integration platform that is both free and open-source. It offers a comprehensive range of functionalities for machine learning and data mining, making it a versatile tool for data analysis. KNIME’s strength lies in its modular data pipelining approach, which allows users to seamlessly integrate various components and leverage the “Building Blocks of Analytics” concept.
By adopting the KNIME platform, users can construct complex data pipelines by assembling and connecting different building blocks tailored to their specific needs. These building blocks encompass a wide array of capabilities, including data preprocessing, feature engineering, statistical analysis, visualization, and machine learning. KNIME’s modular and flexible nature empowers users to design and execute end-to-end analytical workflows, all within a unified and intuitive interface.
Pros:
- Versatile and modular platform for data analytics, reporting, and integration.
- Offers a wide range of building blocks and components for machine learning and data mining.
- Free and open-source.
Cons:
- Steeper learning curve for beginners.
- Limited scalability for large-scale or enterprise-level projects.
- Requires some technical proficiency.
DataRobot
DataRobot automates the end-to-end process of building machine learning models, including data preprocessing, feature selection, and model selection. It provides insights into the decision-making process of machine learning models, allowing analysts to understand and explain the model’s predictions. It also offers functionalities to deploy and monitor models, ensuring ongoing performance evaluation and improvement.
Pros:
- Automated machine learning for streamlined model development.
- Model explainability and transparency for reliable predictions.
- Model deployment and monitoring capabilities.
Cons:
- Advanced customization may require coding skills.
- Steeper learning curve for beginners.
- Pricing can be expensive for large-scale projects.
Comparison Sheet of AI-powered Tools for Data Analysts/Scientists
AI Tool | Features | Price | Pros | Cons |
Google Cloud AutoML | Custom machine learning models | Pay as you go | – Simplifies machine learning model development. – No extensive coding skills required. – Integrates well with the Google Cloud Platform. | – Limited flexibility for advanced model customization. – Pricing can be expensive for large-scale projects. – Dependency on the Google Cloud ecosystem. |
Amazon SageMaker | End-to-end machine learning platform | Tiered usage | – Scalable infrastructure for large-scale projects. – Diverse set of built-in algorithms. – Collaborative environment enhances teamwork. | – Steeper learning curve for beginners. – Advanced customization may require coding skills. – Cost considerations for extensive usage and storage. |
IBM Watson Studio | AI model building, deployment, and management | Lite: Free Professional: $1.02 USD/Capacity Unit-Hour | – Offers a wide range of tools and capabilities for data scientists, developers, and analysts – Facilitates collaboration and automation. – Can be seamlessly integrated with other IBM Cloud services and tools. | – Learning curve may be steep for beginners. – Advanced features and enterprise-level capabilities may require a paid subscription. – Limited flexibility for users who prefer to work with non-IBM or open-source tools and technologies. |
Alteryx | Data blending, advanced analytics, and predictive modeling | Designer Cloud: Starting at $4,950 Designer Desktop: $5,195 | – Comprehensive data blending and preparation capabilities. – Advanced analytics tools for in-depth analysis and modeling. – Workflow automation reduces manual effort and increases efficiency. | – Steeper learning curve for beginners due to the complexity of the tool. – Advanced features and customization may require additional training. -Pricing can be expensive for smaller teams or organizations. |
RapidMiner | Data science platform for enterprise analytics | Available upon request | – Intuitive drag-and-drop interface. – Automated machine learning streamlines the process. – Wide variety of operators for flexible data analysis. | – Limited customization options for advanced users. – Steeper learning curve for complex workflows. – Certain features may require additional licensing. |
Bright Data | Web data collection and analysis | Pay as you go: $15/gb Growth: $500 Business: $1,000 Enterprise: Upon request | – Extensive web data collection capabilities. – High-quality and compliant data. – Data enrichment for deeper analysis. | – Pricing may be prohibitive for small-scale projects. – Steep learning curve for beginners. – Reliance on web data sources can have limitations in certain industries. |
Gretel.ai | Platform for creating synthetic data | Individual: $2.00 /credit Team: $295 /mo + $2.20 /credit Enterprise: Custom | – Synthetic data generation for privacy protection. – Privacy-enhancing techniques for secure analyses. – Data labeling and transformation capabilities. | – Synthetic data may not perfectly represent the complexities of real data. – Limited to privacy-focused use cases. – Advanced customization may require additional expertise. |
MostlyAI | Shareable AI-generated synthetic data | Free Team: $3/credit Enterprise: $5/credit | – Realistic synthetic data generation. – Anonymization and privacy preservation capabilities. – Data utility assessment for reliable analysis. | – Limited to synthetic data generation use cases. – Advanced customization may require technical expertise. – Potential challenges in capturing complex relationships within data. |
Tonic AI | Data anonymization and transformation | Basic: Free trial Professional & enterprise: Custom | – Effective data anonymization techniques. – Rule-based transformations for compliance. – Collaboration and version control capabilities. | – Limited to data anonymization and transformation tasks. Advanced customization may require coding skills. – Certain features may require additional licensing.- |
KNIME | Open-source data analytics and integration platform | Free and paid tiers | – Versatile and modular platform for data analytics, reporting, and integration. – Offers a wide range of building blocks and components for machine learning and data mining. – Free and open-source. | – Steeper learning curve for beginners. – Limited scalability for large-scale or enterprise-level projects. – Requires some technical proficiency. |
DataRobot | Automated machine learning platform | Custom pricing | – Automated machine learning for streamlined model development. – Model explainability and transparency for reliable predictions. – Model deployment and monitoring capabilities. | – Advanced customization may require coding skills. – Steeper learning curve for beginners. – Pricing can be expensive for large-scale projects. |
FAQs
They typically offer a range of features. These include data preprocessing and cleaning capabilities to handle messy datasets, advanced statistical analysis for hypothesis testing and regression modeling, machine learning algorithms for predictive modeling and classification tasks, and data visualization tools to create informative charts and graphs. Additionally, many AI tools provide automation features to streamline repetitive tasks and enable efficient data processing.
AI tools are powerful assistants for data analysts, but they cannot replace the critical thinking and expertise of human analysts. While AI tools can automate certain tasks and perform complex analyses, it is still essential for data analysts to interpret the results, validate assumptions, and make informed decisions based on their domain knowledge and experience. The collaboration between data analysts and AI tools leads to more accurate and insightful outcomes.
AI tools designed for data analysis usually prioritize data privacy and security. They often provide encryption mechanisms to protect sensitive data during storage and transmission. Moreover, reputable AI tools adhere to privacy regulations, such as GDPR, and implement stringent access controls to ensure that only authorized individuals can access and manipulate the data. It is crucial for data analysts to choose AI tools from trustworthy providers and assess their security measures before utilizing them.
While AI tools have numerous benefits, they do have limitations. One limitation is the reliance on quality training data. If the training data is biased or insufficient, it can impact the accuracy and reliability of the tool’s outputs. Another limitation is the need for continuous monitoring and validation. Data analysts must verify the results generated by AI tools and ensure they align with their domain expertise. Additionally, some AI tools may require substantial computational resources, limiting their scalability for larger datasets or organizations with limited computing capabilities.
Data analysts can mitigate risks by adopting a cautious and critical approach when using AI tools. It is crucial to thoroughly understand the tool’s algorithms and underlying assumptions. Data analysts should validate the outputs by comparing them with their own analyses and domain expertise. Regularly monitoring and auditing the tool’s performance is also important to identify any biases or inconsistencies. Additionally, maintaining up-to-date knowledge about data privacy regulations and compliance standards is necessary to ensure proper handling of sensitive information.
Conclusion
While these AI-powered tools offer immense value, it is essential to consider certain factors when using them. Firstly, understanding the limitations and assumptions of the underlying algorithms is crucial to ensure accurate and reliable results. Second, data privacy and security should be prioritized, particularly when working with sensitive or confidential information. It is also important to evaluate the scalability, integration capabilities, and cost implications associated with each tool to align them with specific project requirements.
Read more:
Disclaimer
In line with the Trust Project guidelines, please note that the information provided on this page is not intended to be and should not be interpreted as legal, tax, investment, financial, or any other form of advice. It is important to only invest what you can afford to lose and to seek independent financial advice if you have any doubts. For further information, we suggest referring to the terms and conditions as well as the help and support pages provided by the issuer or advertiser. MetaversePost is committed to accurate, unbiased reporting, but market conditions are subject to change without notice.
About The Author
Cindy is a journalist at Metaverse Post, covering topics related to web3, NFT, metaverse and AI, with a focus on interviews with Web3 industry players. She has spoken to over 30 C-level execs and counting, bringing their valuable insights to readers. Originally from Singapore, Cindy is now based in Tbilisi, Georgia. She holds a Bachelor's degree in Communications & Media Studies from the University of South Australia and has a decade of experience in journalism and writing. Get in touch with her via [email protected] with press pitches, announcements and interview opportunities.
More articles

Cindy is a journalist at Metaverse Post, covering topics related to web3, NFT, metaverse and AI, with a focus on interviews with Web3 industry players. She has spoken to over 30 C-level execs and counting, bringing their valuable insights to readers. Originally from Singapore, Cindy is now based in Tbilisi, Georgia. She holds a Bachelor's degree in Communications & Media Studies from the University of South Australia and has a decade of experience in journalism and writing. Get in touch with her via [email protected] with press pitches, announcements and interview opportunities.