Olas Is Turning the Web into a Network of Agents
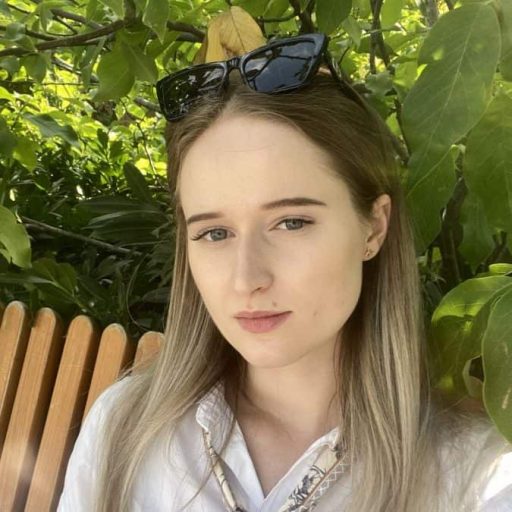
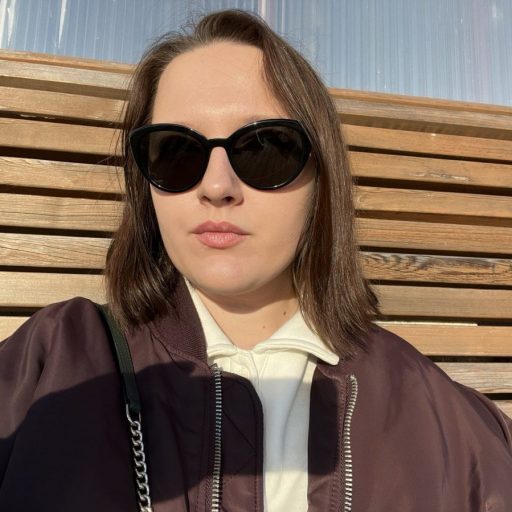
In Brief
David Minarsch, Founding Member of Olas, discusses the future of intelligent agents, combining game theory, machine learning, and decentralized systems, rather than just automating tasks.
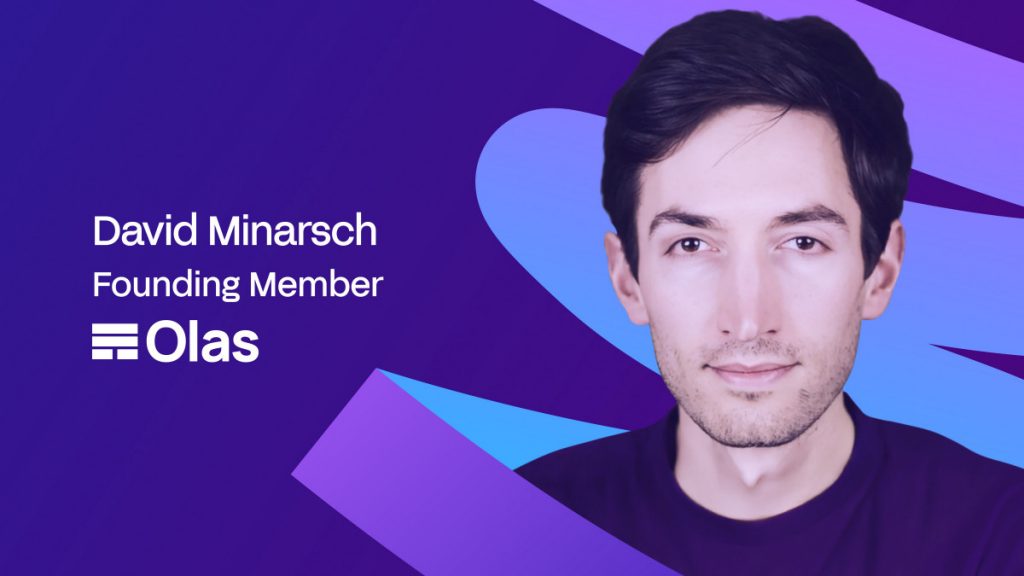
What if the next major shift in Web3 isn’t about apps or protocols, but agents acting on your behalf? In this conversation, we sit down with David Minarsch, the Founding Member of Olas, to unpack a future where intelligent agents don’t just automate tasks—they coordinate economies. With a background that fuses game theory, machine learning, and decentralized systems, David has been building toward this vision long before it became the trend everyone is chasing.
Can you please share your journey into Web3?
I started doing game theory at university, where I was pursuing a PhD in game theory. Afterwards, I worked for a few years in tech and actually tried my first startup in 2017, a venture fund startup. Unfortunately, that didn’t work out, but it made it clear to me that I didn’t want to continue in that direction. I wanted to combine what I had done in my PhD with what I was working on in tech, which at that point was mostly data science and machine learning.
By that time, I had also come across various blockchain technologies. So in 2019, I decided to combine those areas—AI, game theory, and crypto—especially from the perspective of incentives. The question of how to design protocols that incentivize different actors to work together was very compelling to me. In 2019, I joined a protocol called Fetch.AI, which was one of the first to look at agents in crypto. So that was the beginning.
Why did Olas choose to focus on services and agents rather than traditional apps and smart contracts?
If you look at the web more broadly, we seem to be at the beginning of what I sometimes call the fourth wave. Initially, there were websites with “read” functionality. Then came apps that let you read and write—mobile apps, social apps, and web apps. Then came Web3, which introduced the idea of ownership—read, write, and own. And now, across the board, everyone is building agents.
It’s the beginning of turning applications into agents. Instead of a human interacting with an application, the application can now take action on its own. These are agents, and they often have goals and proactively go after them. That trend has been apparent to me for at least six or seven years. Since 2021, with Olas, we’ve been building toward that vision where humans have agents that complement them in various ways. Initially, we focused on crypto-native use cases, like agents helping you invest in digital assets.
In what ways does Olas act more like a coordination layer than just an infrastructure tool?
The protocol itself has a mechanism that is very focused on coordinating agents, which is one of its main growth drivers. That mechanism is called Olas Staking. Olas also includes a bunch of technologies that look like infrastructure—an agent framework, the protocol itself, and various tooling—but the coordination aspect is more dominant.
From a growth perspective, this mechanism, called staking, is built around an innovation we introduced: Proof of Active Agent. It defines KPIs for a given agent use case. If the agents meet those KPIs, they are rewarded. This is implemented as a staking mechanism. Whoever owns the agent can “stake” the agent, and if it hits its KPIs, it unlocks staking rewards.
This is particularly interesting because you can direct emissions very specifically toward particular agent use cases, which helps bootstrap marketplaces. For example, we have a marketplace, which is a bazaar for AI agents. Some agents buy services; others sell them. Like any marketplace, you need both demand and supply. If you have demand but no supply, or vice versa, the marketplace struggles. The staking mechanism helps solve that chicken-and-egg problem.
Olas claims to unlock permissionless coordination. How is this fundamentally different from simply being decentralized?
Part of the answer is that Olas is decentralized. Everything about it has a permissionless character. The only permissions are at the token governance level. The protocol reserves certain roles for holders of the Olas token.
Otherwise, it’s permissionless. With staking, we create incentives for actors to rally around certain objectives. For instance, we encourage coordination around agent use cases and also incentivize builders to create agents aligned with those use cases. If there are staking incentives for the agent marketplace, you as a builder are incentivized to build an agent that offers services there.
How can agents in the Olas ecosystem evolve their strategies without centralized updates?
There are at least two ways I can think of. First, agents are user-owned. They run on devices that the users control, like a laptop or server. So the user decides when and how to update the agent.
Second, agents are designed to access tools. These tools can be anything from writing to a database to using another model or running workflows. For example, we have agents that participate in prediction markets. They use tools that take market data as input and produce a probability. The agent then uses that output to decide whether to participate and with how much capital.
In Olas, agents can remotely access these tools. Some agents host tools for others. One agent might make a micropayment to use another agent’s tool remotely. So, a trading agent can outsource some of its prediction workflows to another agent via the marketplace. This happens dynamically and doesn’t require code updates. The agent just follows its goal and chooses the best tools based on performance.
What makes the Olas token more than just a remote utility? How does it coordinate?
The primary coordination mechanism is the staking system I mentioned earlier. For example, if I run a prediction agent, I gain utility from its performance in prediction markets. However, I can also stake Olas tokens to earn additional rewards if the agent meets its KPIs.
Users can delegate staking to the agent, which will then handle it autonomously. This enables the agent to earn extra yield. From the protocol’s perspective, Olas tokens coordinate behavior because the staking contract KPIs are defined through Olas governance.
Governance operates through a vote escrow mechanism: users lock tokens to participate. Governance decides which staking contracts receive emissions. So, Olas token holders shape protocol growth by directing incentives. This coordination creates a multi-sided marketplace with agents on both sides, users running them, and governance steering the ecosystem.
How does Olas safeguard against model collapse or malicious behavior in AI agents?
Olas doesn’t have a built-in safeguard mechanism. What it does is optimize for the emergence of agents that perform well based on the KPIs set in staking contracts. We don’t currently have contracts targeting anti-malicious behavior directly.
Instead, the system defines KPIs that aim for positive macro-level outcomes. If agents don’t participate as expected—say, not using the marketplace—they don’t earn rewards. So while there’s no direct enforcement, Proof of Active Agent staking gives us a way to nudge behavior toward desired outcomes.
As agent autonomy becomes modular, do you see the rise of specialist micro-agents replacing monolithic AI systems?
Absolutely, and that’s already happening in Olas. The marketplace facilitates this specialization. One agent can just offer predictions and sell them to other agents who act on that data.
We also have use cases like agents.fun—a social agent that acts like an influencer. You can give it a persona, and it will post as that persona. It might post texts, videos, audio, or images. It uses the marketplace to access the necessary tools, so the user doesn’t need API keys.
This approach benefits both users and agent autonomy. Agents become more capable over time as more tools appear on the marketplace. They can dynamically tap into those tools instead of trying to be a single, monolithic AI system.
Could AI agents within Olas serve as interfaces for real-world legal, financial, or governance systems?
That would certainly be the hope. We already have financial use cases. With Modius, a user delegates funds to a self-custodial agent that manages those assets in DeFi.
We also experimented with a governance agent called Carbonator, which is more of a concept study, but it still runs. Users could potentially delegate voting power to such an agent. The key value is abstraction: users can express strategies in natural language, and the agent takes care of the complexity.
This has big implications. In traditional finance, apps are simple to use—you don’t need to understand stock markets or lending protocols. In crypto, that complexity is exposed. Agents abstract that away, making it easier for users to interact with Web3.
What new regulatory categories or policy frameworks might autonomous AI agents require?
That’s a complicated question. As agents become more autonomous, the challenges grow. While they behave like apps today, some developers are building more like digital personas with independent goal management. That could raise questions about legal personhood.
Even now, if I delegate goals to my agent and it makes a costly mistake, who is liable? In traditional software, the creator or provider is usually liable. However, with open-source agents, like those offered under the Apache 2.0 license, there are no warranties. The user runs the software. Is the user liable, or is the agent considered a separate legal entity?
It’s similar to what we saw with self-driving cars: if there’s an accident, who is responsible? I think regulators will have to look into this space, if they haven’t already.
Could agents not only perform tasks but also curate demand by predicting market needs?
I would say yes, because curating demand is also a task. From a game theory perspective, any actor—business, human, or software agent—takes actions that influence outcomes and their utility.
You might have an agent that invests your crypto funds in DeFi. It sources data, makes decisions based on indicators, or even taps into third-party services. This specialization is often necessary. For example, blockchains are hard to query directly. You need indexers who build a historical picture.
So one agent might specialize in indexing or analyzing data to predict demand. Others might act on that data. This already exists in Olas Predict, where agents participate in prediction markets. Some agents forecast outcomes like interest rate changes, which influence investment strategies downstream. So agents are already beginning to curate demand.
What’s the roadmap for Olas?
We’re core contributors, so I can speak to you about what we’re working on at Valerie. We focus on two areas. First is Pearl—an end-user-facing application and agent app store. You can download it on your desktop and run agents from the app store. We’re working to improve that experience and add more agents.
Second is the agent marketplace. We’re making it easier for developers to onboard agents from other ecosystems, improving discoverability, and helping the community understand what’s happening on the marketplace. These are our current core priorities.
Disclaimer
In line with the Trust Project guidelines, please note that the information provided on this page is not intended to be and should not be interpreted as legal, tax, investment, financial, or any other form of advice. It is important to only invest what you can afford to lose and to seek independent financial advice if you have any doubts. For further information, we suggest referring to the terms and conditions as well as the help and support pages provided by the issuer or advertiser. MetaversePost is committed to accurate, unbiased reporting, but market conditions are subject to change without notice.
About The Author
Victoria is a writer on a variety of technology topics including Web3.0, AI and cryptocurrencies. Her extensive experience allows her to write insightful articles for the wider audience.
More articles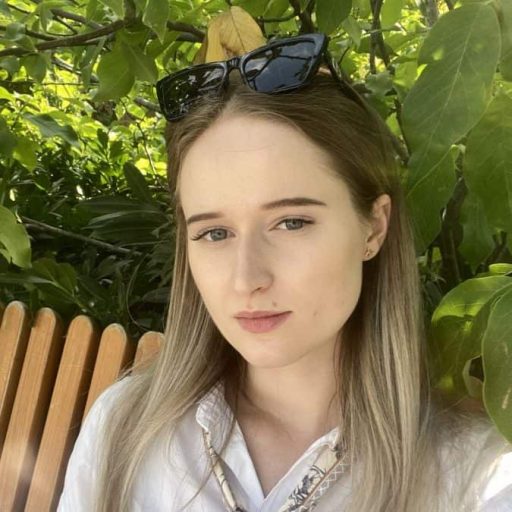
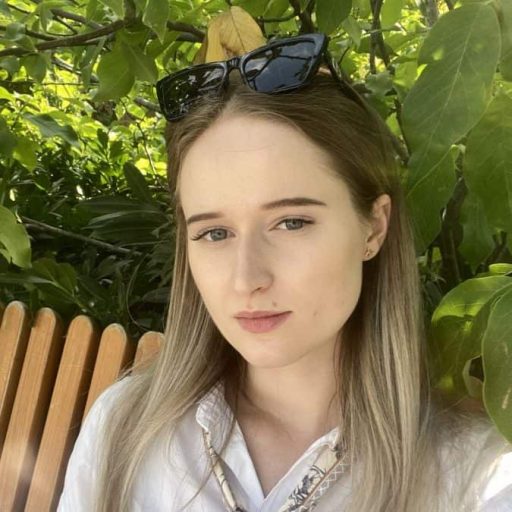
Victoria is a writer on a variety of technology topics including Web3.0, AI and cryptocurrencies. Her extensive experience allows her to write insightful articles for the wider audience.